AI’s Mid-Market Makeover in Financial Services
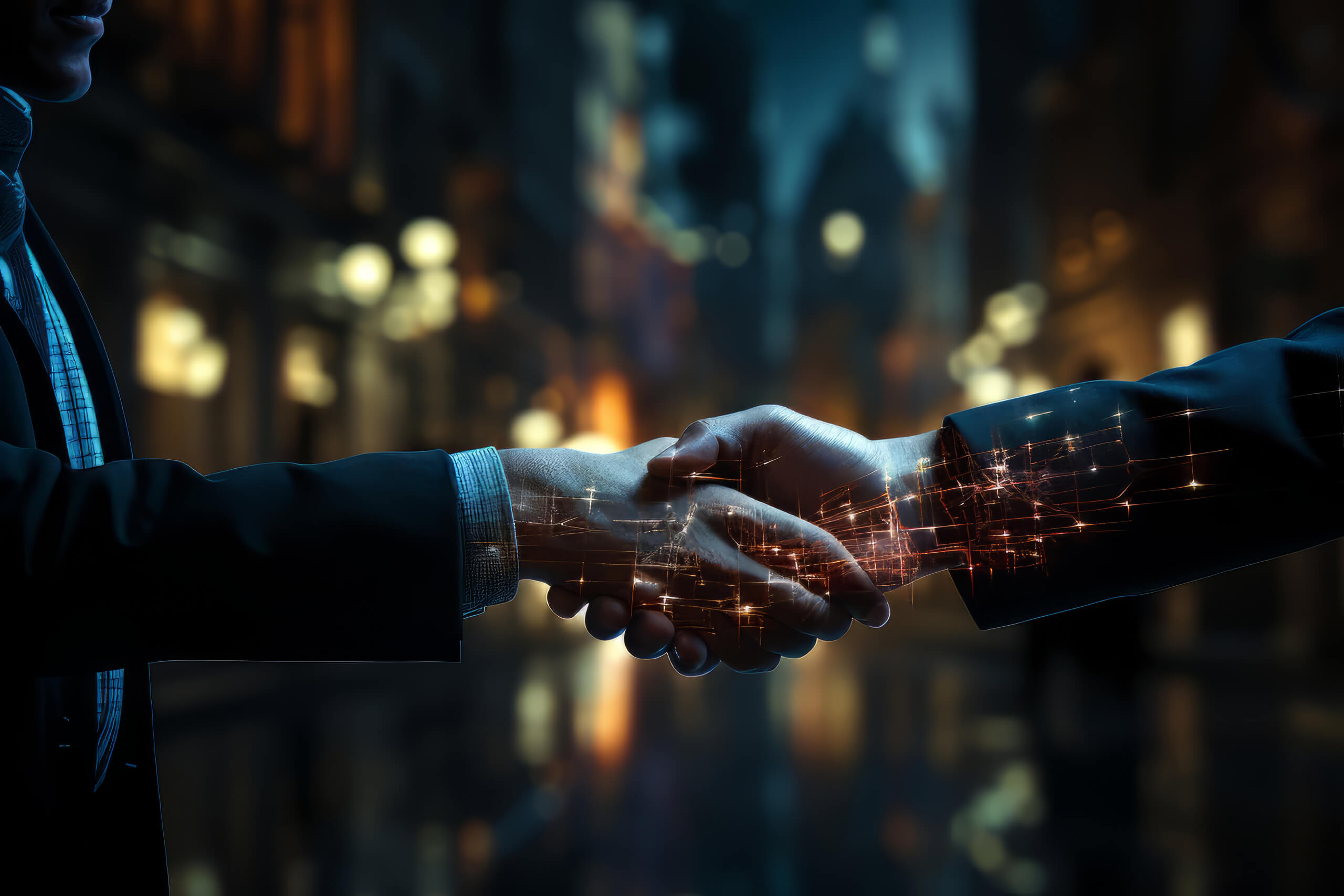
Summary
Mid-sized financial services institutions (FIs) are facing significant challenges during this period of rapid technological change, particularly with the rise of artificial intelligence (AI). As customer expectations grow, smaller banks and lenders must stay competitive and responsive. Canada’s largest financial institutions are already advancing in AI, while many others remain in ‘observer’ mode, hesitant to invest and experiment. Yet, mid-sized FIs that adopt the right strategy have unique agility, allowing them to adapt swiftly and efficiently to technological disruptions—even more so than their larger counterparts.
This report explores how AI can tackle these challenges by transforming operations and generating substantial value across departments and business lines. We present a detailed approach for identifying, prioritizing, and implementing AI use cases, empowering mid-sized financial institutions to maintain a competitive edge in the fast-changing financial industry.
AI offers a major opportunity to enhance productivity, personalize customer interactions, improve decision-making, and create new business models. Successful AI integration requires a balanced, strategic approach that aligns with business goals and carefully evaluates potential risks.
Several challenges facing mid-sized financial services companies
In a time of rapid technological advancements and changing market conditions, mid-sized financial services companies are at a crossroads. They must meet rising customer expectations and deal with growing competition, presenting them with unique challenges that can either hinder their growth or drive them to seek new opportunities. Here are the top four challenges in this area:
Rising Customer Expectations
Today’s customers seek personalized, responsive, and holistic banking and borrowing experiences tailored to their individual preferences and needs. 72% of customers now view personalization as a critical factor in their interactions with financial institutions.[1] The shift to personalized banking requires adopting technologies for 24/7 digital access and real-time responses, transforming customer service into more dynamic, user-centered models.
Competitive Pressures
The competition in financial services includes not only larger, resource-rich banks but also agile FinTech startups, significantly challenging mid-sized companies from two different sides. With FinTech revenues expected to increase sixfold by 2030, the industry’s competitive dynamics are increasingly based on technological advancement and new financial products.[2]
Workforce Dynamics and People Constraints
As technology reshapes the financial sector, the demand for skilled talent, particularly in emerging technologies like AI and machine learning, is soaring. However, mid-sized firms often face challenges in acquiring and retaining such talent. Training and upskilling employees in crucial areas are vital, yet there is a significant hurdle: resistance to change. With only 10% of millennials committed long-term to their financial services roles, companies need to create more engaging and supportive work environments to retain their top talent.[3]
Technology and AI Disruption
The disruption brought about by technology and AI offers both opportunities and challenges. As of mid-2024, there are over 700,000 generative AI models available on the Hugging Face repository—a doubling in just eight months—highlighting the rapid pace of technological change.[4] The integration of new technologies can set industry benchmarks, but the cost and evolving regulatory frameworks surrounding AI pose difficultiesthat must be navigated carefully.
In this exploration, we look at how mid-sized FIs can use AI to overcome challenges and improve operations and customer engagement. In a following article, we will prioritize AI use cases to help these institutions make informed decisions.
Creating Value Across All Departments
Amid the challenges faced by mid-sized financial firms, AI stands out as a key driver of progress. It enables transformative change, boosts operational efficiency, personalizes customer interactions, enhances decision-making, and fosters the development of new business models. By addressing these challenges, AI has the potential to create significant differentiation and impact every aspect of the business.
Here’s how AI can boost businesses:
Increase Productivity and Efficiency | Personalize Customer Experiences | Augment Decision Making | Invent New Business Models |
Increase Productivity & Efficiency
AI-driven tools are revolutionizing operational workflows by streamlining processes and reducing manual intervention. Through intelligent document processing, regulatory compliance monitoring, and process automation, financial services firms can achieve unprecedented levels of productivity. By leveraging AI-powered copilots to augment humans in tasks like email management and coding modernization, organizations can optimize their day-to-day operations and enable their workforce to focus on higher-value activities.
Personalize Customer Experience
In today’s competitive market, personalization is key to customer satisfaction, and AI is at the forefront of this capability. With AI-powered sentiment analysis and recommendation engines, financial services companies can tailor services and products to meet individual customer needs more effectively. Furthermore, AI-driven customer engagement tools, from text-to-video communications to generative experiences, enable businesses to create seamless, personalized journeys that enhance customer loyalty and retention.
Augment Decision Making
AI augments decision-making processes by providing real-time, data-driven insights that enhance business strategies. From underwriting assistance to dynamic pricing and risk scoring, AI enables financial institutions and other businesses to make informed decisions faster and more accurately. By integrating AI in areas like fraud detection and smart financial advisory, companies can mitigate risks while optimizing their decision-making processes, leading to improved outcomes and competitive advantage.
Invent New Business Models
AI is not just about optimizing existing processes; it also drives the creation of entirely new business models. For example. the advent of AI-powered embedded finance and autonomous lending platforms demonstrates how AI can unlock new revenue streams and business opportunities. Through insights-as-a-service offerings and new partnership value streams, organizations can capitalize on their data, leverage AI to explore untapped markets, and invent business models that could disrupt traditional banks and lenders.
In a subsequent article, we’ll explore these topics in depth, breaking them down with detailed explanations and real-world use cases.
GenAI Value Creation: Aspiration vs. Reality
As financial institutions navigate the complex landscape of AI adoption, two distinct sentiments emerge, each underpinned by its own set of expectations and concerns.
On one hand, the optimists in the financial sector view GenAI as a revolutionary force capable of driving significant industry changes. This group is excited by the potential for:
True Hyper-personalization: Tailoring products and services to individual preferences and needs for every single customer.
Maximum Customer Experience: Leveraging conversational AI to improve service quality and user engagement across all channels.
Automation Everywhere: Implementing AI-driven processes to streamline operations and reduce the need for manual and paper-based work.
Giant Productivity Leaps: Increasing efficiency & effectiveness across all organizational levels, by augmenting employees to focus on the highest value activities.
Zero-Fraud Financial Systems: Using advanced AI algorithms to detect and prevent fraud in real-time.
These optimists are encouraged by projections suggesting that GenAI could unlock over $1 trillion in banking revenue by 2030[1], signaling a major shift in how financial services are structured and delivered. Moreover, 75% of executives anticipate that GenAI will drive disruptive changes within the industry over the next three years.[2]
Conversely, the skeptics approach GenAI with caution, emphasizing the potential pitfalls over the promised perks. Their concerns include:
Boom & Bust Cycles: Risk of overinvestments driving unsustainable growth, low ROI, and financial problems down the road, as was the case with several technology waves in the past decades.
Reliability Issues: Worries about the limitations and flaws of new GenAI technologies, and their impact on mission-critical financial processes where the room for error is very small.
Regulatory Constraints: Challenges to AI growth due to evolving, stringent regulations across local and federal jurisdictions in financial services.
Security and Privacy Risks: Increased risk of cyber threats, data privacy concerns, and potential bias as AI continues to handle and process more sensitive information on customers and employees.
Overpromise, Underdeliver: Many worry about the prospect of AI failing to meet the current hype, and see in it an over-marketed tech that is less revolutionary that it seems.
Interestingly, despite the much touted prospects of AI, a significant 66% of executives surveyed express ambivalence or dissatisfaction with their organization’s progress in AI integration, highlighting a gap between expectation and execution.[3]
Embracing AI Optimism with Prudence
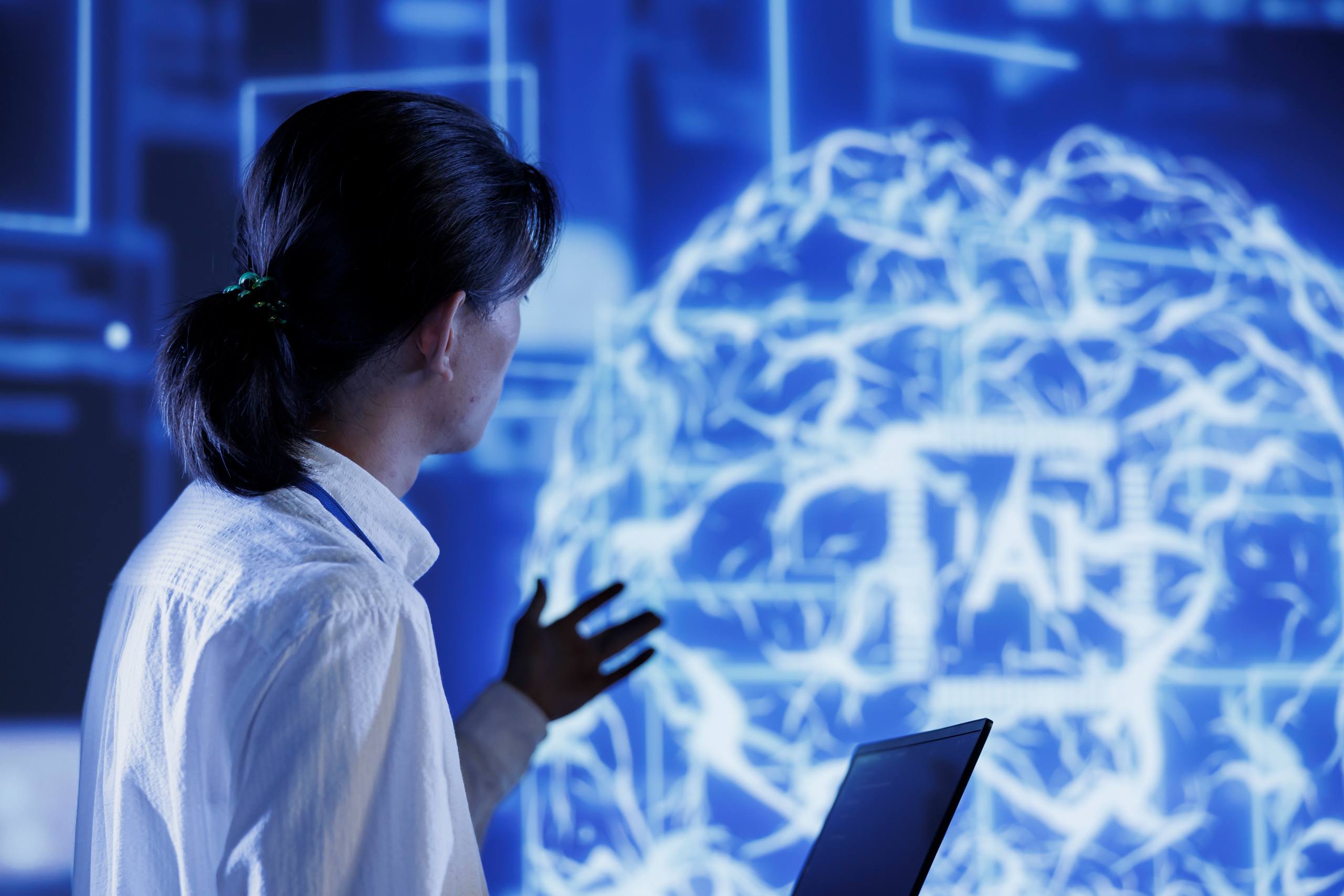
AI encompasses a wide array of tools and capabilities, including battle-tested solutions based on both predictive and generative AI. Recognizing the full range of AI technologies will enable us to leverage the most effective solutions for our specific needs.
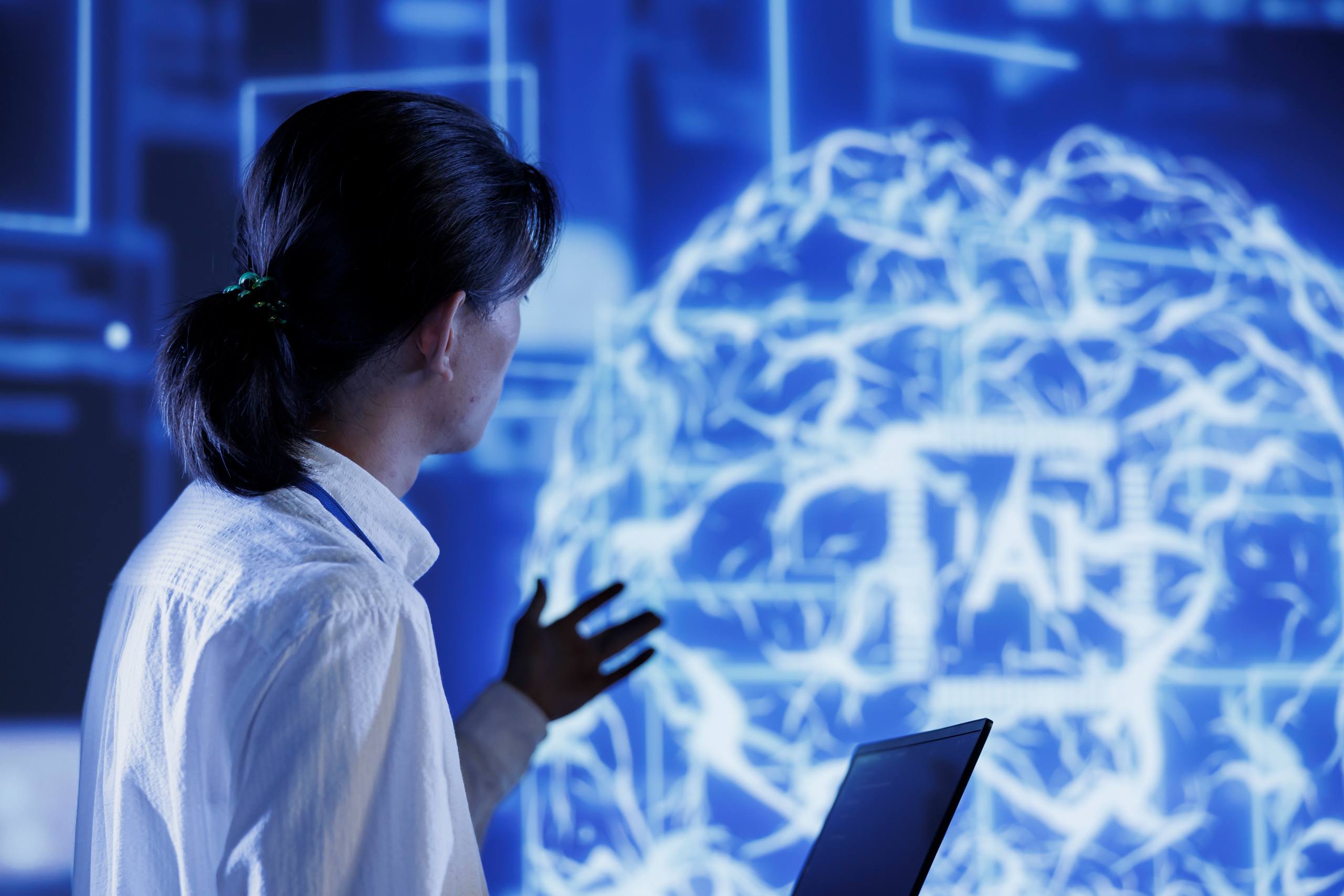
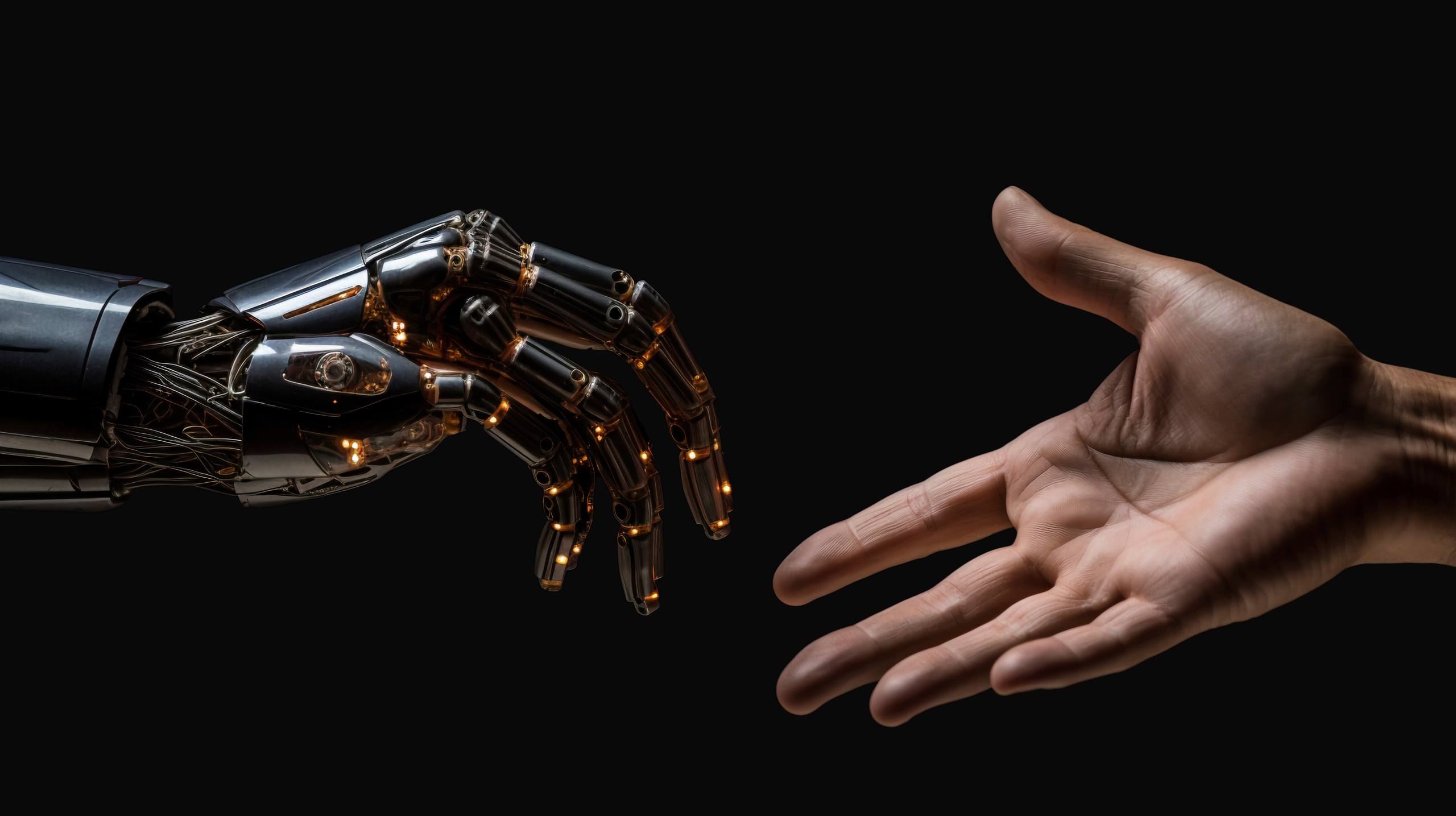
Like humans, AI is not infallible—it makes mistakes. However, combining human oversight with GenAI’s capabilities could enhance our decision-making processes beyond what’s achievable by human efforts alone.
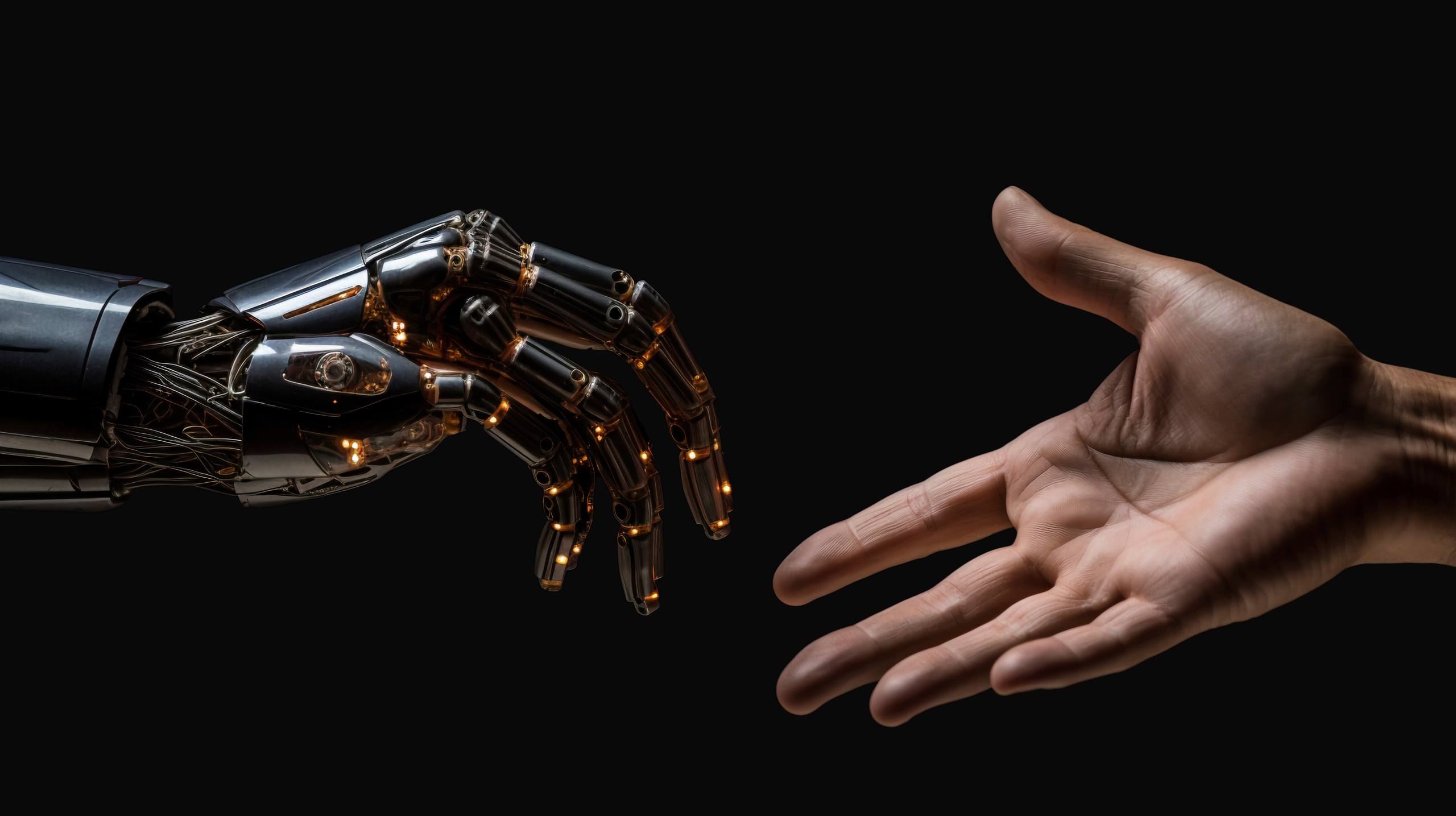
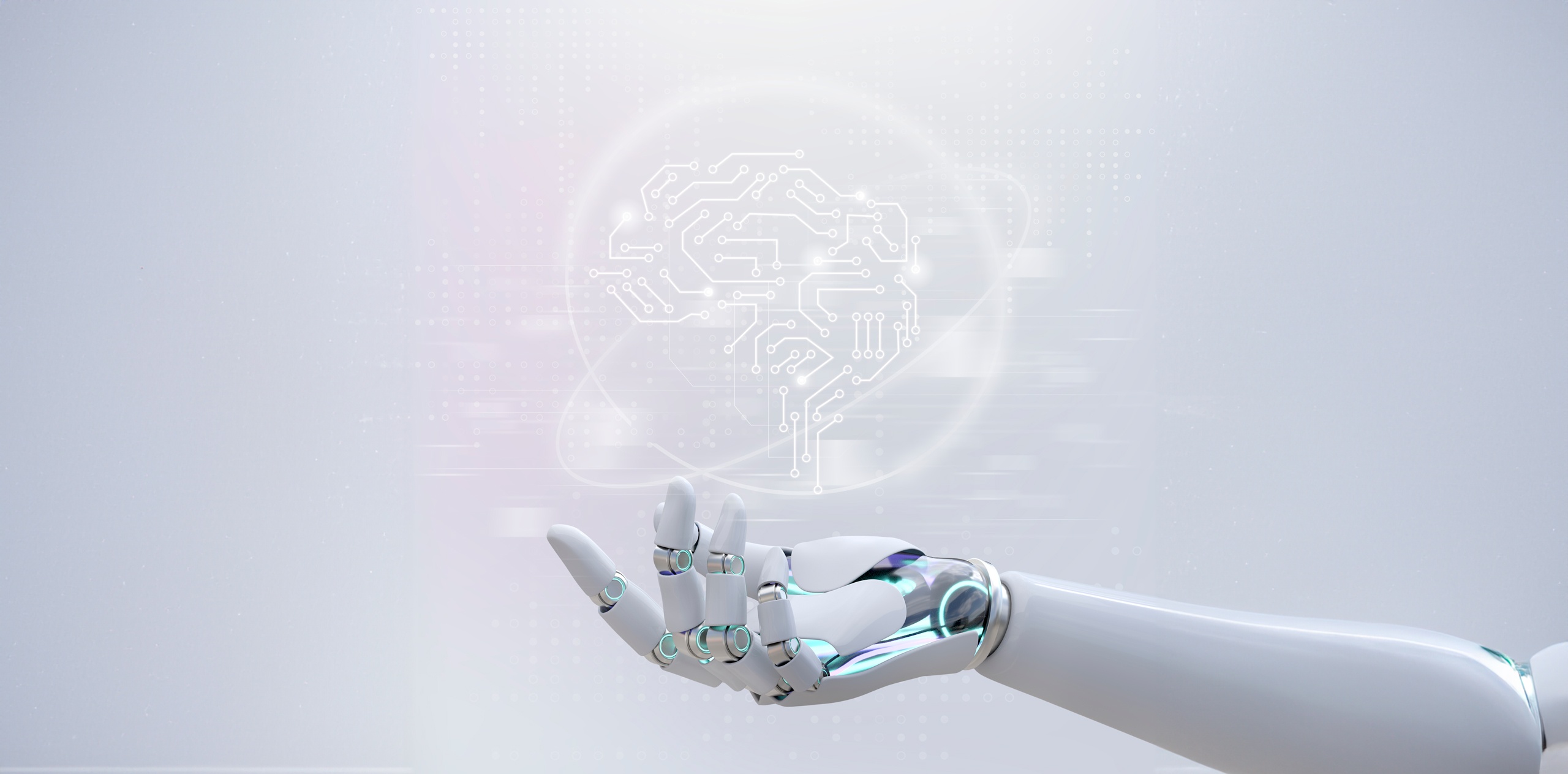
The rapid progress of AI means what’s cutting-edge today might be outdated tomorrow. We should consider the potential of future AI developments, much like how each model (e.g. GPT-4o) has expanded on the capabilities of its predecessors. Looking into the not-so-distant future, imagine what GPT-14 would be capable of vs. GPT-4.
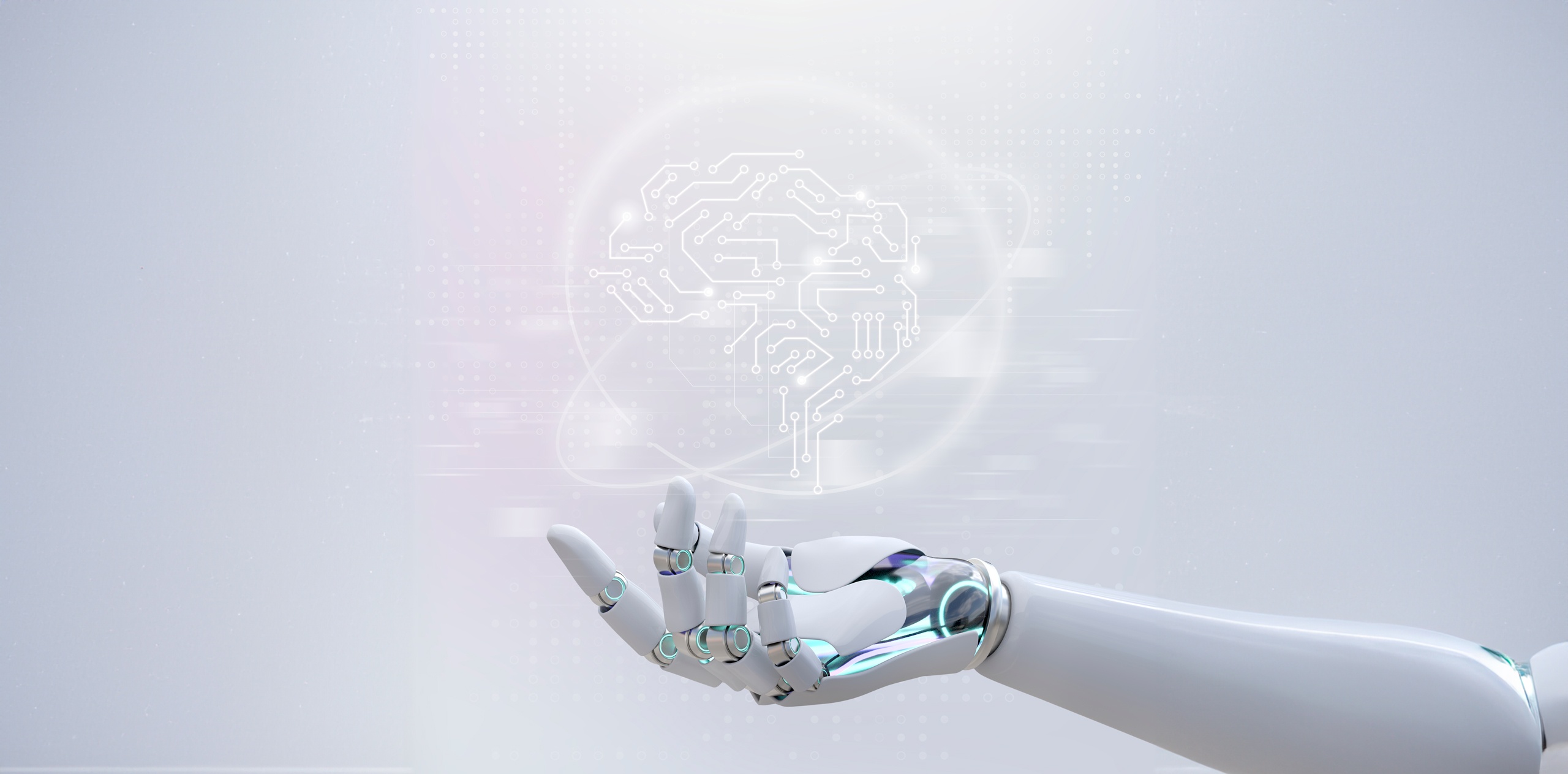
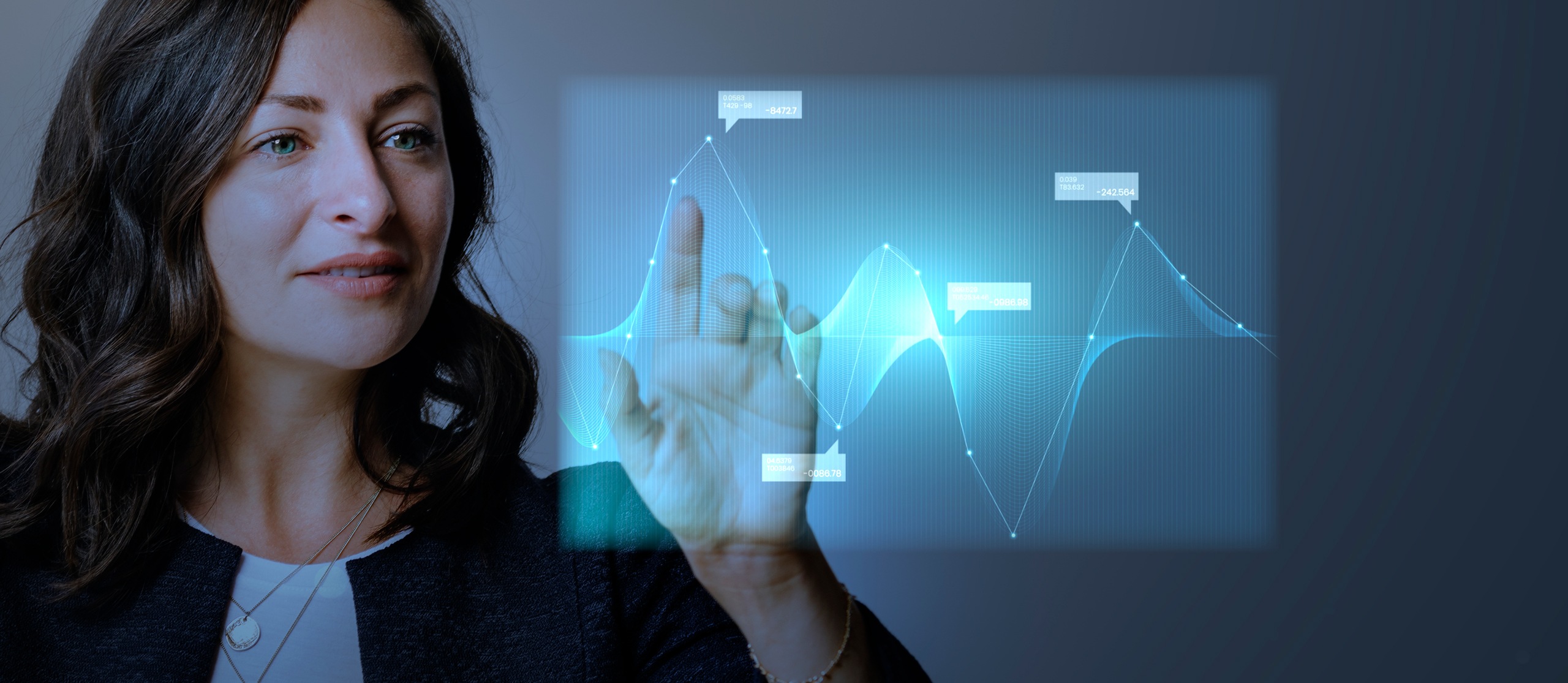
AI isn’t a one-size-fits-all solution, and should not be treated solely as a technology problem. Rather than adopting AI for its own sake, it’s vital to focus on strategic AI use cases that align with our specific business goals.
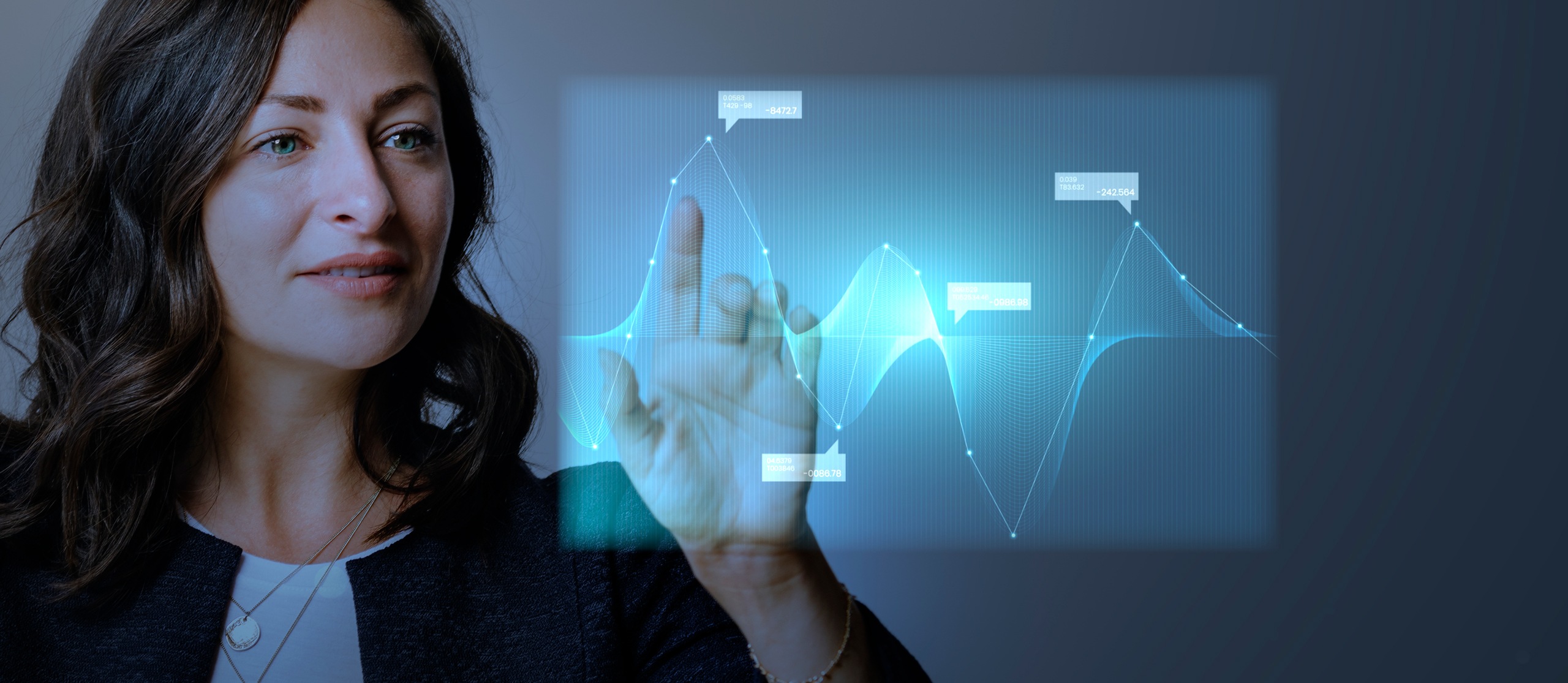
The Spectrum of AI Adoption in Financial Services: Where Companies Stand
In the financial services sector, firms vary significantly in their approach and adoption of artificial intelligence. This diversity is best understood through four distinct groups: Observers, Experimenters, Implementers, and Innovators. Each category defines the group’s current engagement with AI technologies and also underscores its strategic vision and operational readiness for future disruptions.
Observers
Many observers are choosing to wait for large, proven returns on investment (ROI) and clearer technology convergence before diving into AI. While this cautious stance lets them learn from early adopters, it risks putting these FIs behind competitors who are seizing AI-driven advantages now.
Experimenters
Experimenting FIs are cautiously dipping their toes into AI through small-scale projects and pilot programs, testing the waters to gauge capabilities and potential benefits without diving into full-scale implementation. This approach nurtures a culture of learning and adaptability, but without a comprehensive strategy, its impact and scalability may remain limited.
Implementers
Implementers are more advanced in their AI journey, embedding AI systems within specific departments and processes to generate tangible business value. These FIs are building significant AI capabilities and gaining advantages in differentiation and operational efficiency. However, their challenge is to scale these benefits across the entire organization to prevent siloed success and ensure widespread impact.
Innovators
Innovators are embedding comprehensive AI strategies across their business models, reshaping their operations and potentially revolutionizing the financial services industry for a powerful competitive edge. However, this ambitious approach comes with heightened risks and substantial investments, making the journey to success both challenging and uncertain.
Competitive AI Landscape: Lessons from Canada’s Big Financial Institutions
As mid-sized FIs consider the question of AI, it’s crucial to recognize the significant strides made by Canada’s largest banks. Notably, three of the Big Five banks are ranked among the top ten globally for AI maturity [4], showcasing their leadership in using AI to transform financial services. Here’s how some of these banks are creating competitive advantage with AI:
![]() |
Ranked among the top three globally for AI maturity, RBC owns Borealis AI, a dedicated research and development institute. It has launched AI-driven products like Aiden for trading and NOMI for personal finance management, and utilizes AI to enhance internal processes and modernize legacy systems. |
![]() |
With its AI R&D center, Layer 6, TD has grown its AI talent to over 200 professionals and is developing over 50 AI solutions across its business lines. It is the leading patent filer in AI among Canadian FIs (450+ AI-related patent filings) and uses predictive AI models to pre-approve mortgages in seconds, demonstrating a strong commitment to integrating AI across its operations.[5] |
![]() |
Winner of the Best Gen-AI Initiative by The Digital Banker 2024, CIBC has developed its own custom built ‘CIBA AI’ system to improve productivity and enable staff to focus on high-value activities. The introduction of tools like GitHub CoPilot boosts developer efficiency, and the bank plans to significantly expand its data and AI workforce by hiring 200+ related roles over the next 12 months.[6] |
![]() |
Scotiabank’s customer-facing AI chatbot handles 40% of queries with a 90% accuracy rate and has significantly sped up agent training. The bank has also launched a global AI platform to enhance customer insights across all touchpoints and employs large language models to improve employee experiences.[7] |
![]() |
BMO considers AI integral to its bank strategy. Its mobile app provides AI-driven personalized insights to improve customer engagement, its ‘Digital Workbench’ offers real-time analytics for commercial clients, and its contact centers’ service quality is boosted through NLP tech. Last year, it upskilled over 3,500 employees through learning & development programs centered on AI and cloud. Externally, BMO demonstrated commitment to AI by sponsoring the ‘Next AI’ accelerator.[8] |
Strategic Imperative for Mid-Sized FIs
The AI advancements by Canada’s largest banks illustrate a clear trend: AI is not just a technological upgrade but a strategic necessity. Mid-sized FIs must recognize the need to keep pace and innovate beyond traditional technologies and models. Key actions for staying ahead include:
- Accelerated AI Adoption and Scale-Up: Mid-sized FIs need to prioritize their own AI roadmaps and seek opportunities to rapidly scale adoption of AI solutions across various departments.
- Target High-Value AI Applications: Focus on areas where AI can quickly deliver the most significant returns, such as improving customer experience and increasing operational efficiency – before exploring how AI can enable the development of new financial products & services.
- Invest in AI Talent and Partnerships: Expand internal capabilities by hiring AI specialists and consider partnerships with AI tech & service providers to tap into external expertise.
- Monitor and Adapt Best Practices: Keep a close eye on industry leaders and continuously adapt their AI best practices to suit smaller scale operations and unique market demands.
By embracing these strategies, mid-sized FIs can respond to the competitive pressures exerted by larger banks and carve out their own niche in the rapidly evolving financial landscape.
Competitive AI Landscape: Lessons from Canada’s Big Financial Institutions
As mid-sized FIs consider the question of AI, it’s crucial to recognize the significant strides made by Canada’s largest banks. Notably, three of the Big Five banks are ranked among the top ten globally for AI maturity [4], showcasing their leadership in using AI to transform financial services. Here’s how some of these banks are creating competitive advantage with AI:
![]() Ranked among the top three globally for AI maturity, RBC owns Borealis AI, a dedicated research institute. It has launched AI-driven products like Aiden for trading and NOMI for personal finance management, and utilizes AI to enhance internal processes and modernize legacy systems. |
![]() With its AI R&D center, Layer 6, TD has grown its AI talent to over 200 professionals and is developing over 50 AI solutions across its business lines. It is the leading patent filer in AI among Canadian FIs (450+ AI-related patent filings) and uses predictive AI models to pre-approve mortgages in seconds, demonstrating a strong commitment to integrating AI across its operations.[5] |
![]() Winner of the Best Gen-AI Initiative by The Digital Banker 2024, CIBC has developed its own custom built ‘CIBA AI’ system to improve productivity and enable staff to focus on high-value activities. The introduction of tools like GitHub CoPilot boosts developer efficiency, and the bank plans to significantly expand its data and AI workforce by hiring 200+ related roles over the next 12 months.[6] |
![]() Scotiabank’s customer-facing AI chatbot handles 40% of queries with a 90% accuracy rate and has significantly sped up agent training. The bank has also launched a global AI platform to enhance customer insights across all touchpoints and employs large language models to improve employee experiences.[7] |
![]() BMO considers AI integral to its bank strategy. Its mobile app provides AI-driven personalized insights to improve customer engagement, its ‘Digital Workbench’ offers real-time analytics for commercial clients, and its contact centers’ service quality is boosted through NLP tech. Last year, it upskilled over 3,500 employees through learning & development programs centered on AI and cloud. Externally, BMO demonstrated commitment to AI by sponsoring the ‘Next AI’ accelerator.[8] |
Strategic Imperative for Mid-Sized FIs
The AI advancements by Canada’s largest banks illustrate a clear trend: AI is not just a technological upgrade but a strategic necessity. Mid-sized FIs must feel the urgency to not only catch up but also innovate beyond traditional models to remain competitive. The following strategic actions are recommended:
- Rapid AI Adoption and Scale-Up: Mid-sized FIs need to prioritize AI adoption and seek opportunities to scale AI solutions across their operations quickly.
- Focus on High-Impact AI Applications: Identify areas where AI can create significant value, such as enhancing customer experience, improving operational efficiency, and developing new financial products.
- Invest in AI Talent and Partnerships: Expand internal capabilities by hiring AI specialists and consider partnerships with AI tech & services firms to leverage external expertise.
- Monitor and Adapt Best Practices: Keep a close eye on industry leaders and continuously adapt their AI best practices to suit smaller scale operations and unique market demands.
By embracing these strategies, mid-sized FIs can respond to the competitive pressures exerted by larger banks and carve out their own niche in the rapidly evolving financial landscape.
Conclusion
The financial services industry is at a turning point, especially for mid-sized FIs. Adopting AI is no longer a choice but a necessity to remain competitive and meet the growing demands of the market. With extensive experience working with mid-sized FIs, Blanc Labs recognizes the transformative potential AI brings—from boosting operational efficiency to tailoring customer experiences and introducing new business models. As technology continues to advance at a rapid pace, it’s crucial that these institutions take decisive action to leverage these tools. By embracing AI now, your organization can gain a competitive advantage, fuel growth, and position itself for long-term success in an increasingly fast-paced industry.
The next article in this series will explore real-world examples of AI in action within financial services and offer a structured approach to identifying and prioritizing the most impactful AI applications for mid-sized FIs.
Latest Insights
Lenders Transformation Playbook: Bridging Strategy and Execution
AI’s Mid-Market Makeover in Financial Services
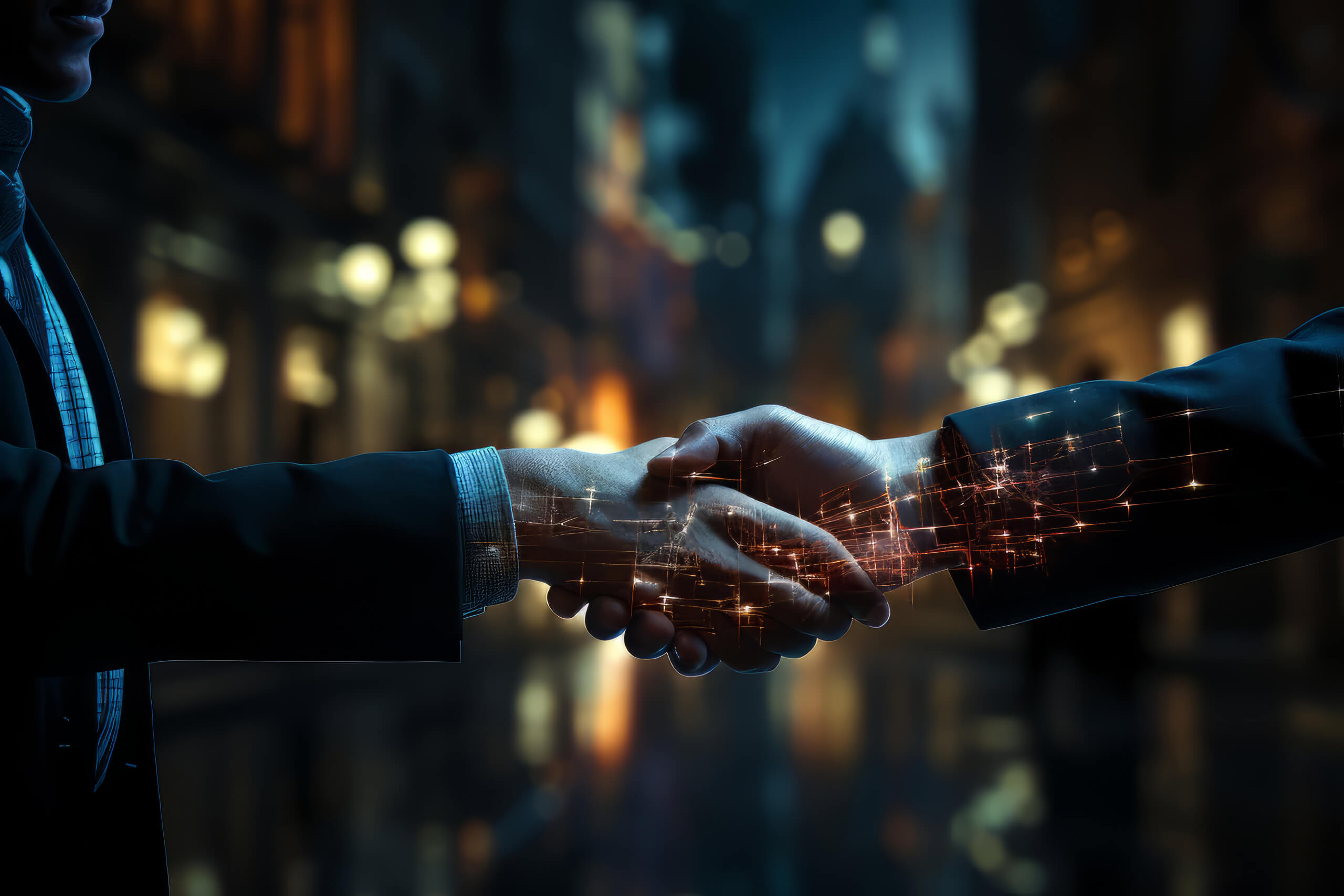
Mid-sized financial services institutions (FIs) are facing significant challenges during this period of rapid technological change, particularly with the rise of artificial intelligence (AI). As customer expectations grow, smaller banks and lenders must stay competitive and responsive. Canada’s largest financial institutions are already advancing in AI, while many others remain in ‘observer’ mode, hesitant to invest and experiment. Yet, mid-sized FIs that adopt the right strategy have unique agility, allowing them to adapt swiftly and efficiently to technological disruptions—even more so than their larger counterparts.
Process Improvement and Automation Support the Mission at Trez Capital 🚀
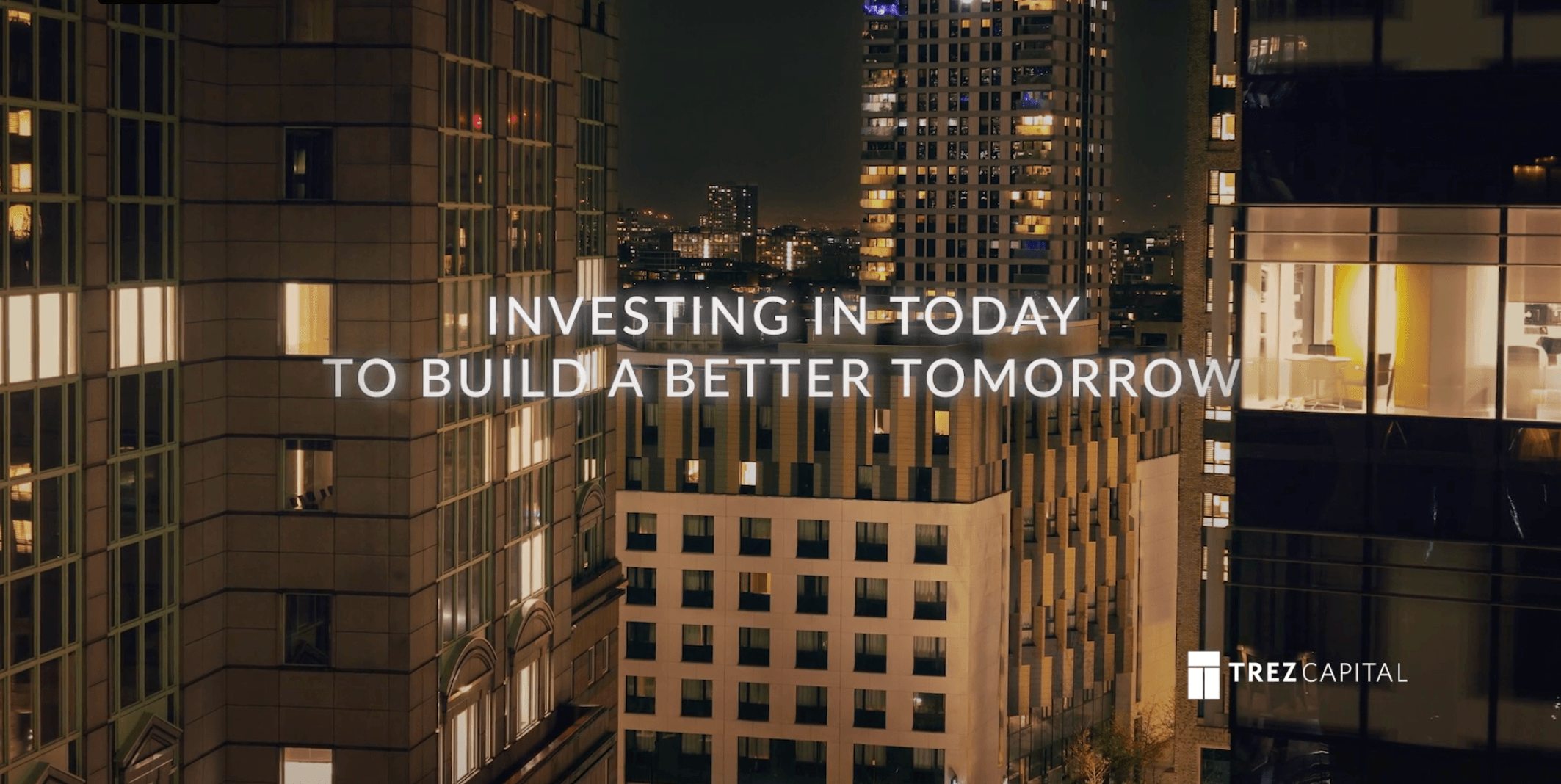
Trez distributes capital based on very specific criteria. But with over 300 investments in their portfolio, they process numerous payment requests and deal with documents in varied data formats. They saw an opportunity to enhance efficiency, improve task management, and better utilize data insights for strategic decision-making.
Align, Assemble, Assure: A Framework for AI Adoption
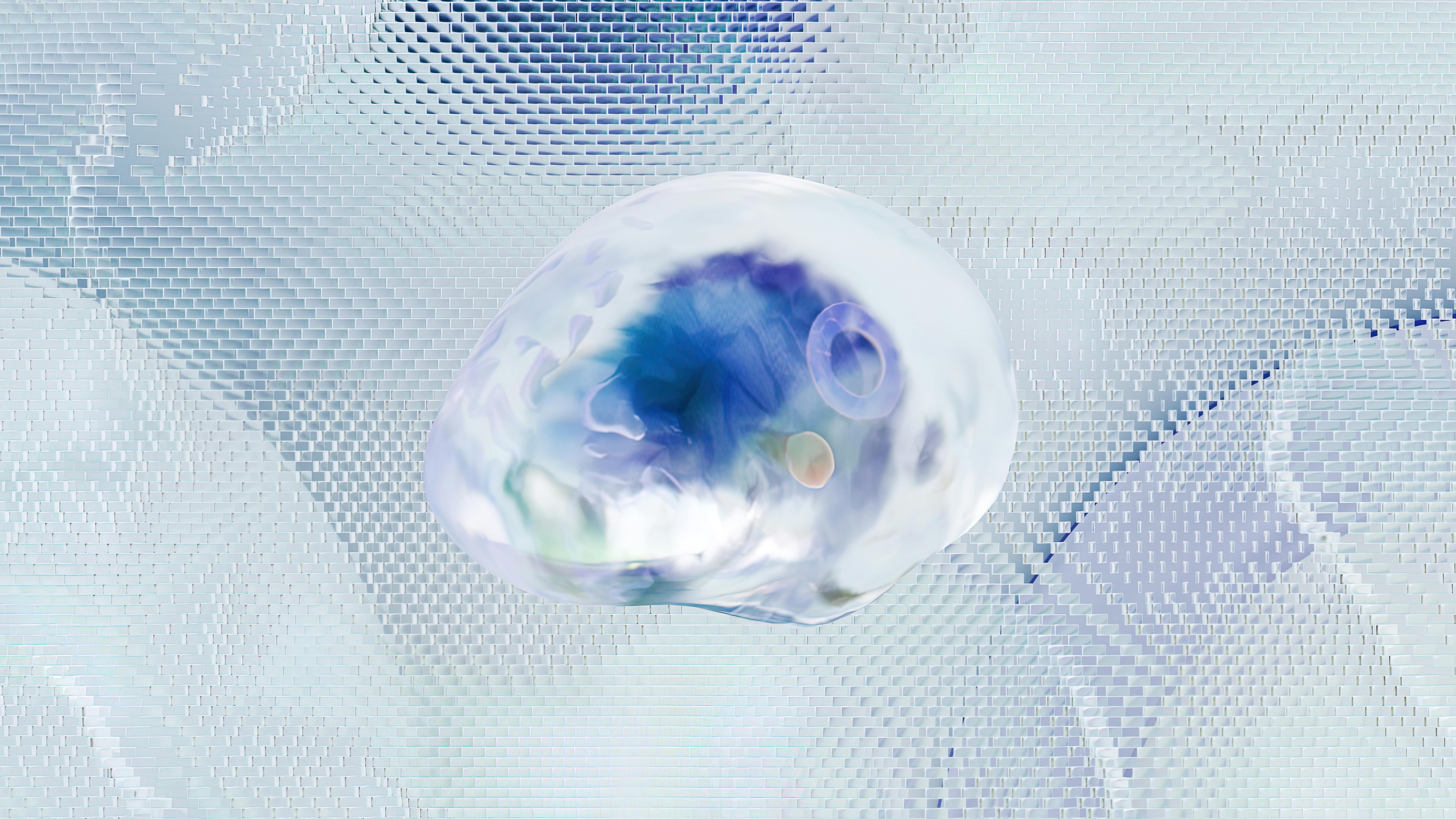
An in-depth guide for adopting and scaling AI in the enterprise using actionable and measurable steps.
Blanc Labs Welcomes Two New Leaders to Advance AI Innovation and Enhance Tech Advisory Services for Financial Institutions Across North America
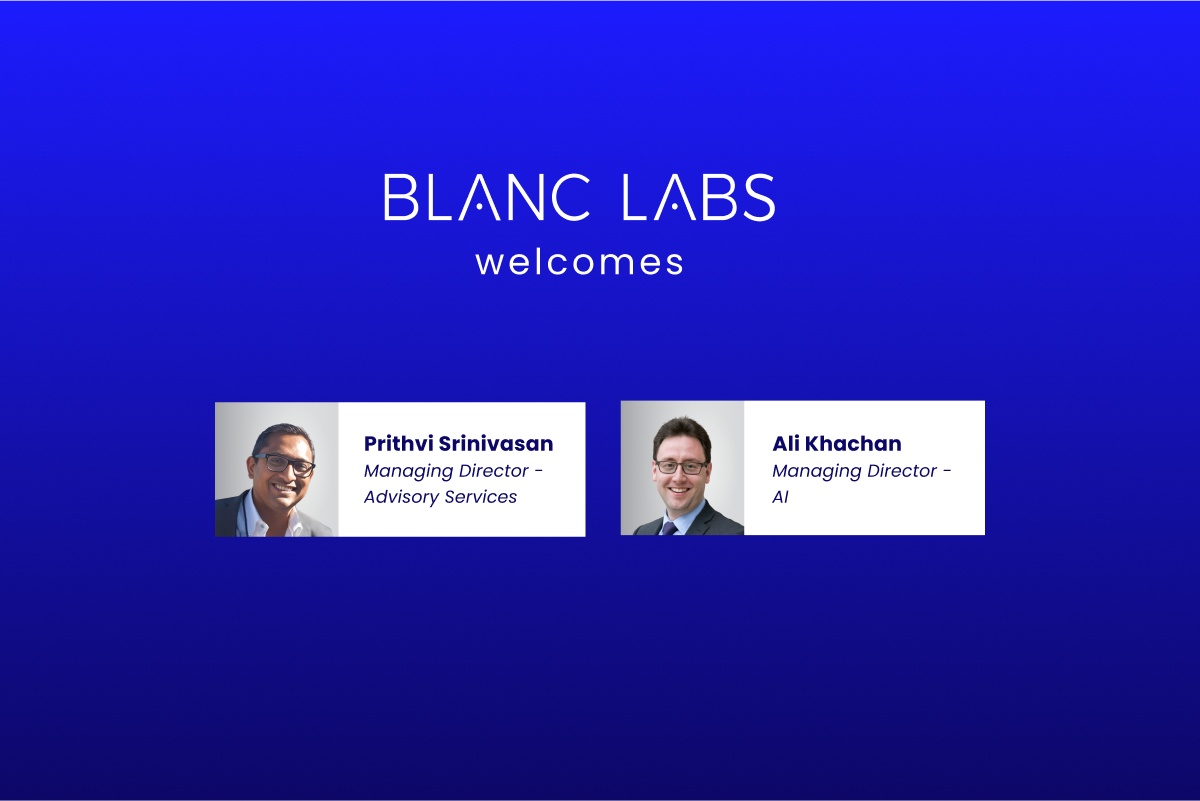
Blanc Labs and TCG Process have partnered to transform lending operations with innovative automation solutions, using the DocProStar platform to enhance efficiency, compliance, and customer satisfaction in the Canadian lending market.
Blanc Labs Partners with TCG Process to Integrate their Automation and Orchestration Platform and deliver Advanced Intelligent Workflow Automation to Financial Institutions
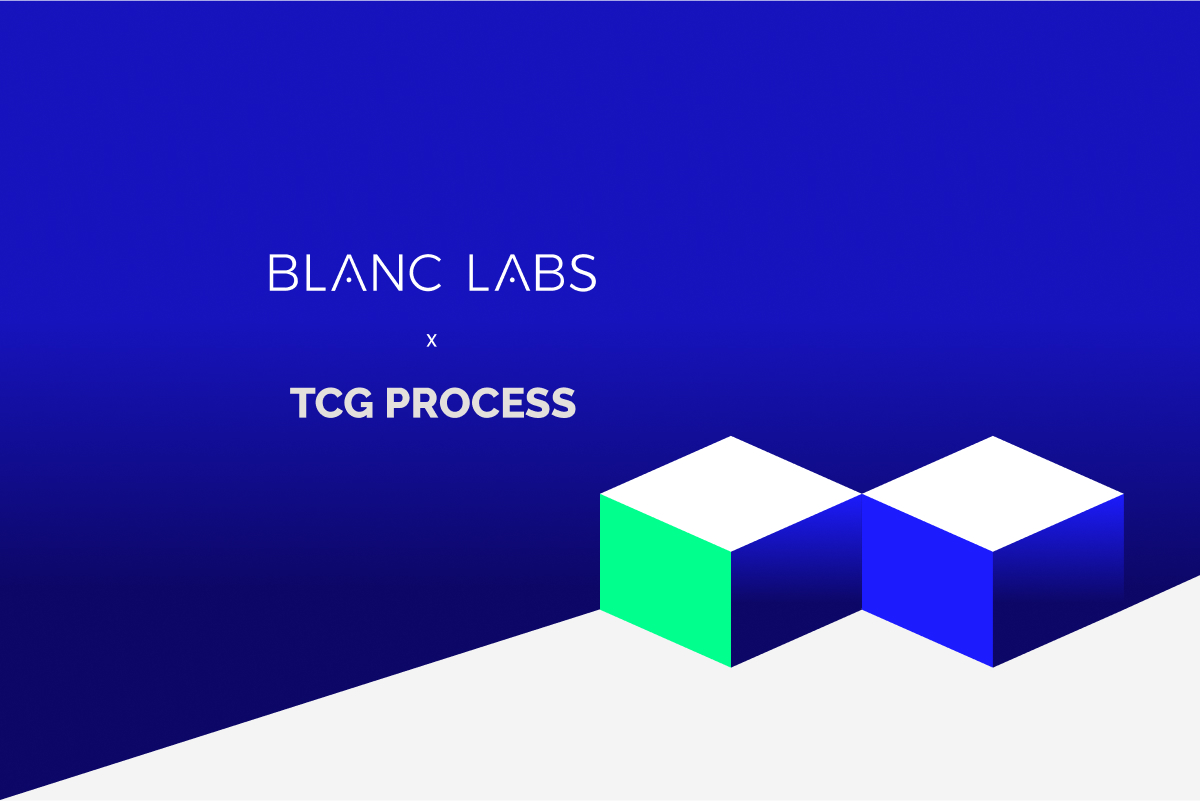
Blanc Labs and TCG Process have partnered to transform lending operations with innovative automation solutions, using the DocProStar platform to enhance efficiency, compliance, and customer satisfaction in the Canadian lending market.
BPI in Banking and Financial Services in the US & Canada
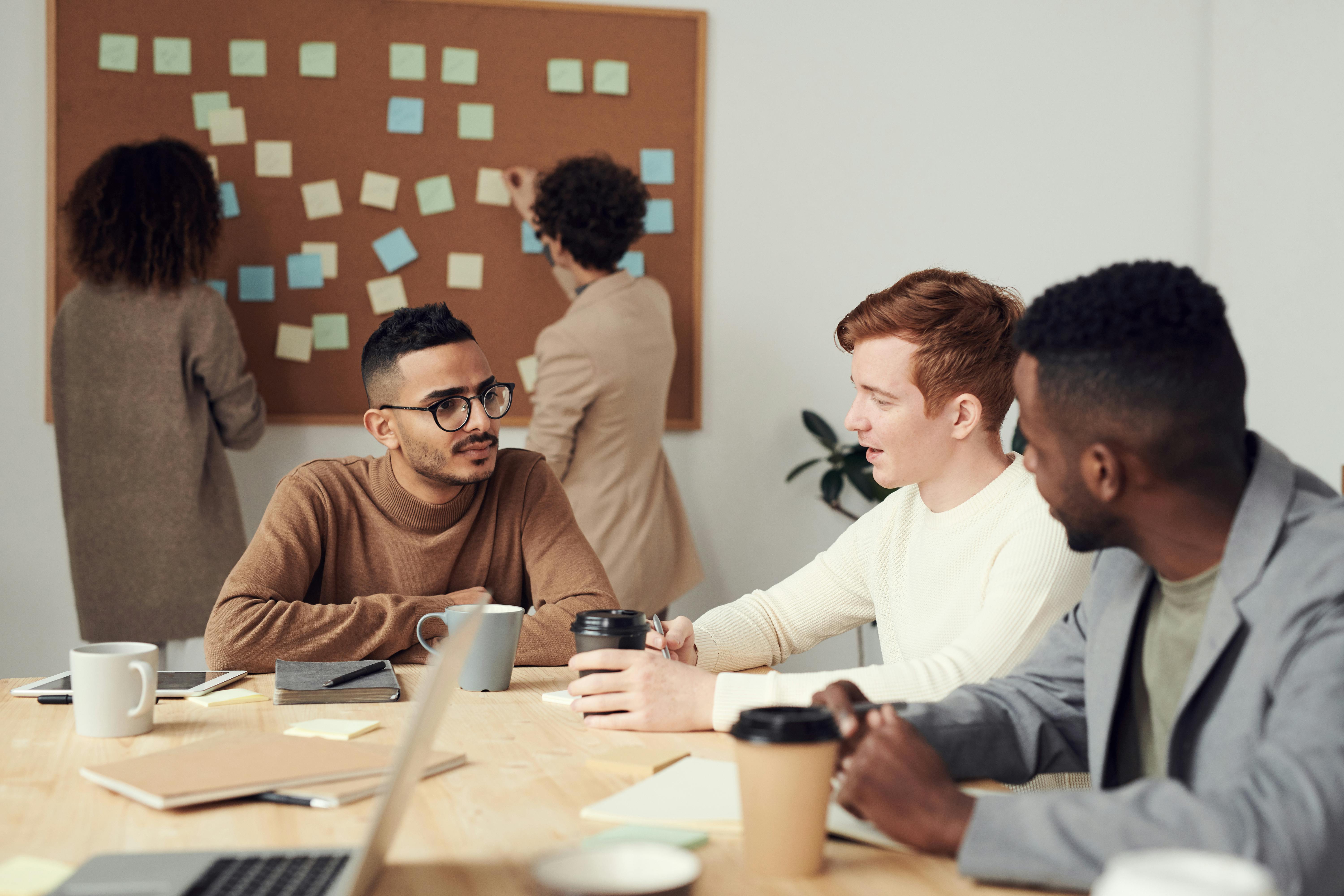
Banking and financial services are changing fast. Moving from old, paper methods to new, digital ones is key to staying in business. It’s important to think about how business process improvement (BPI) can help.
Business Process Improvement vs Business Process Reengineering
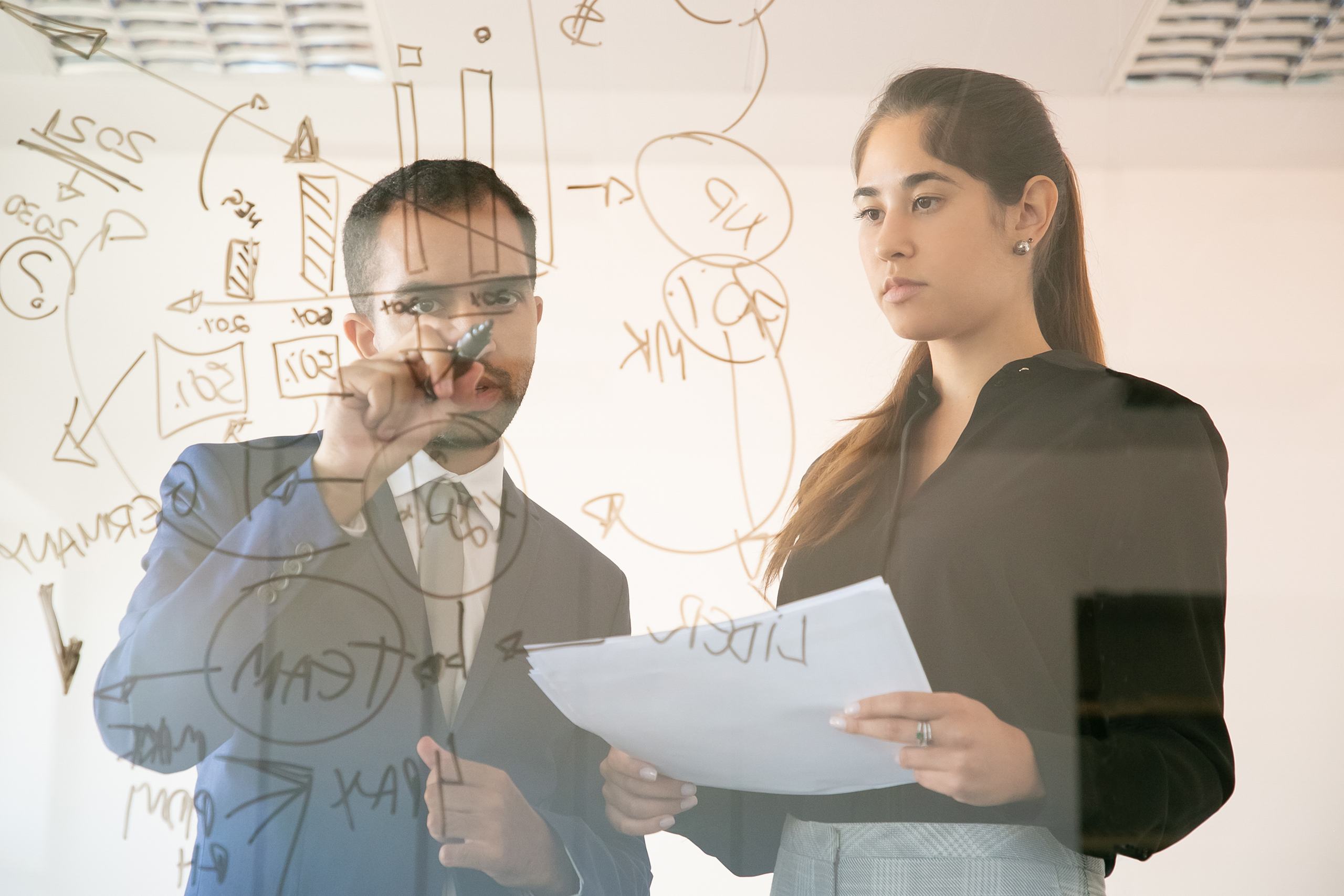
Business process improvement vs. reengineering is a tough choice. In this guide, we help you choose between the two based on four factors.
What is the role of a Business Process Improvement Specialist?
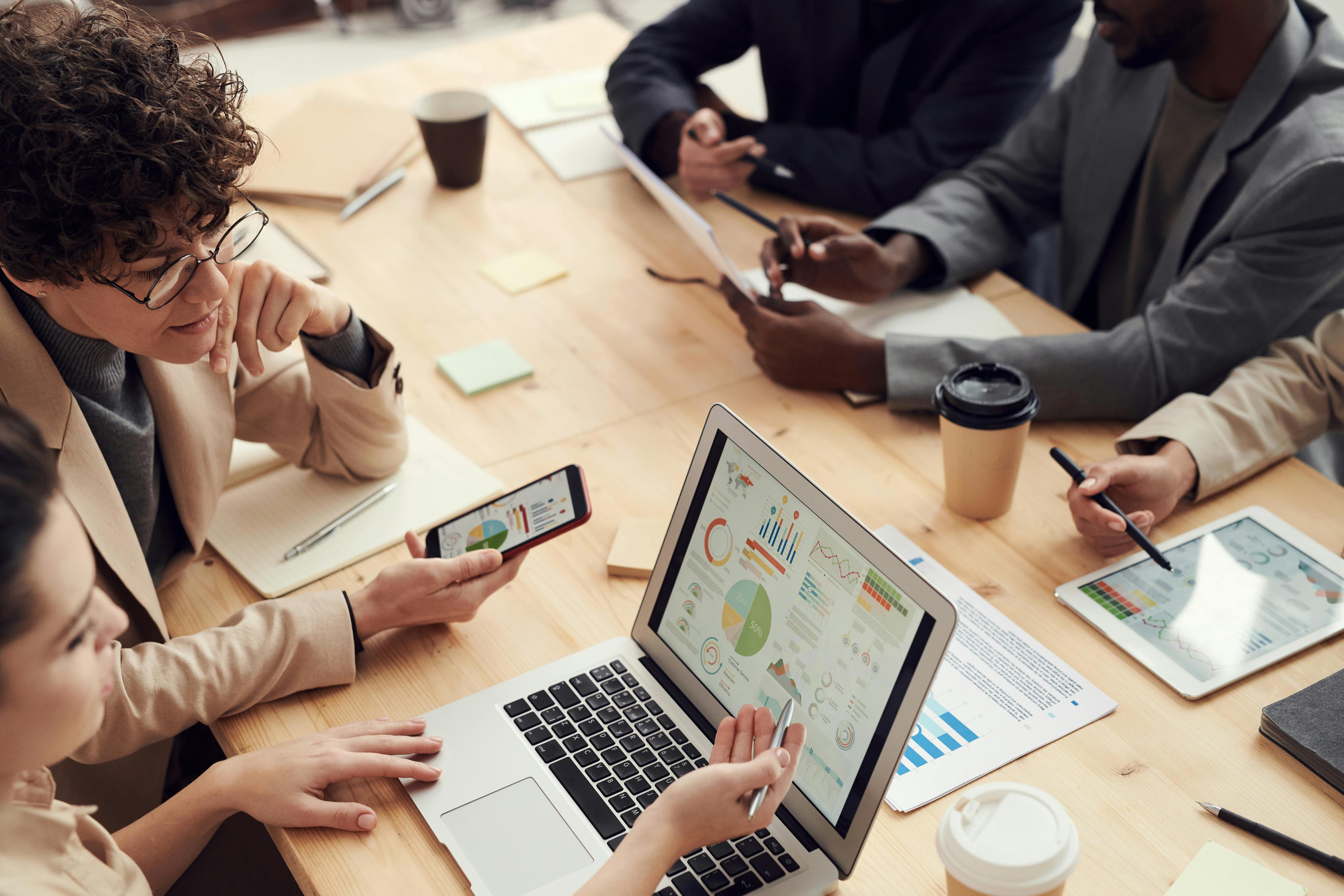
A business process improvement specialist identifies bottlenecks and inefficiencies in your workflows, allowing you to focus efforts on automating the right processes.
Open Banking Technology Architecture Whitepaper
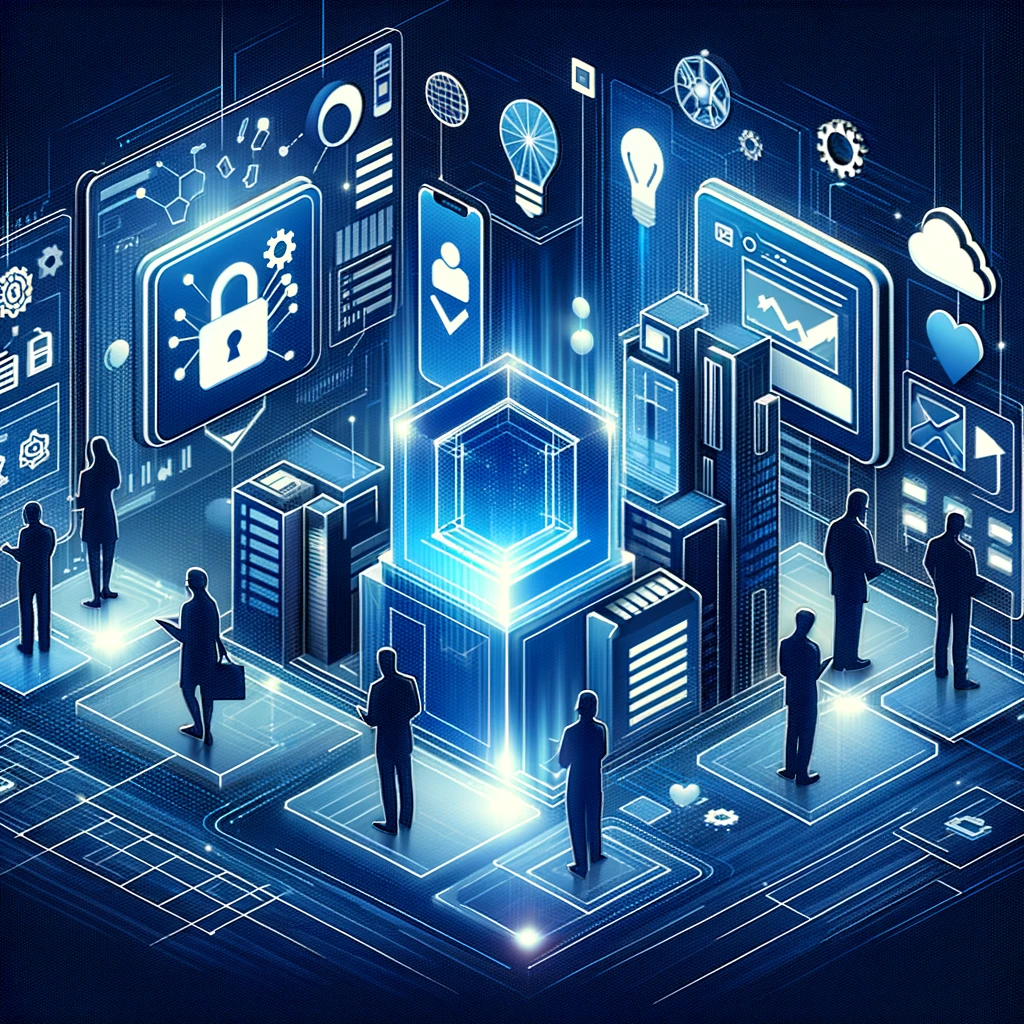
We’ve developed this resource to help technical teams adopt an Open Banking approach by explaining a high-level solution architecture that is organization agnostic.
Winning the Open Banking Race: A Challenger’s Path to Entering the Ecosystem Economy
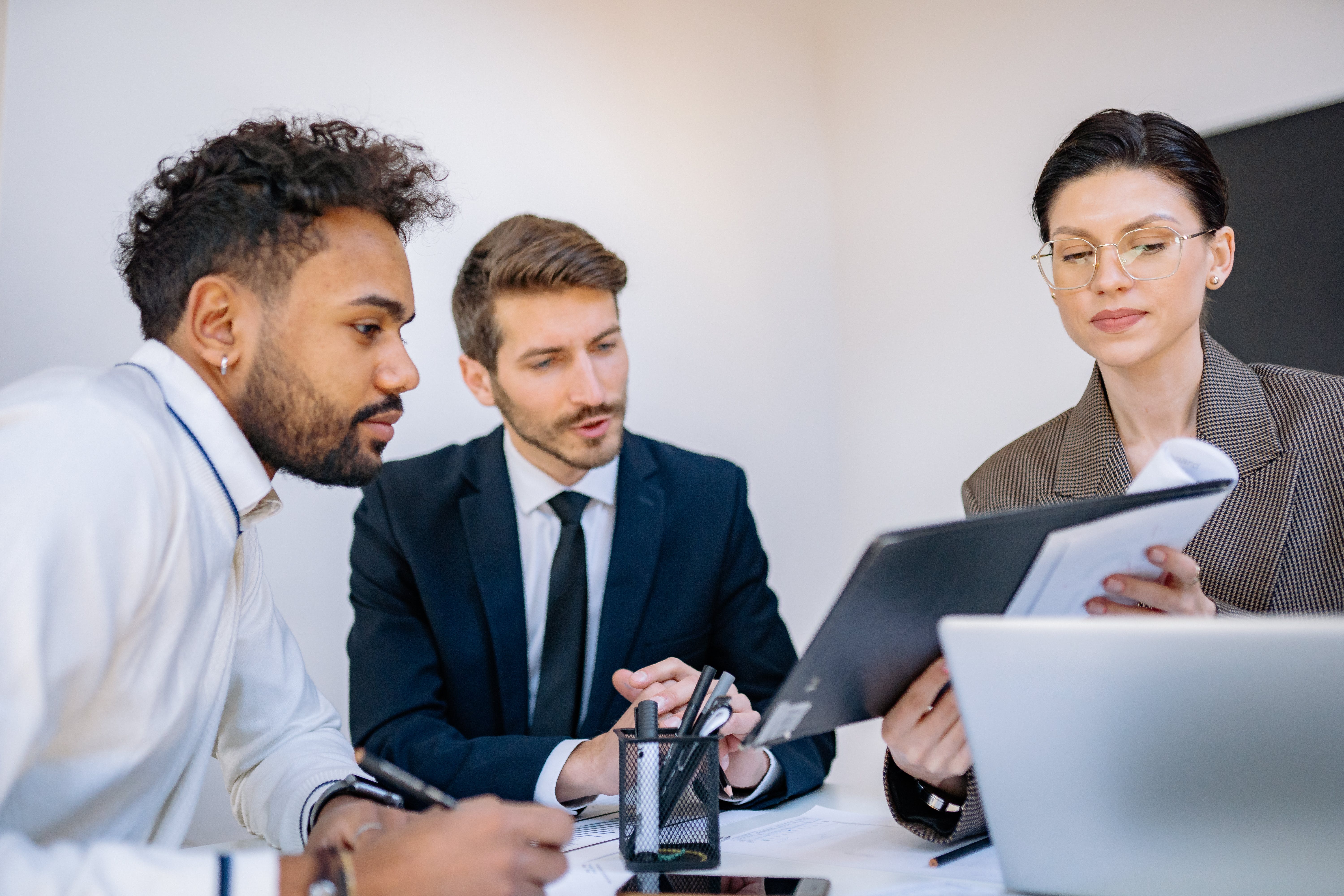
Learn about the steps you can take in forming an open banking strategy and executing on it.
Canadian IT services firms offer a strategic opportunity for US Banks and FIs
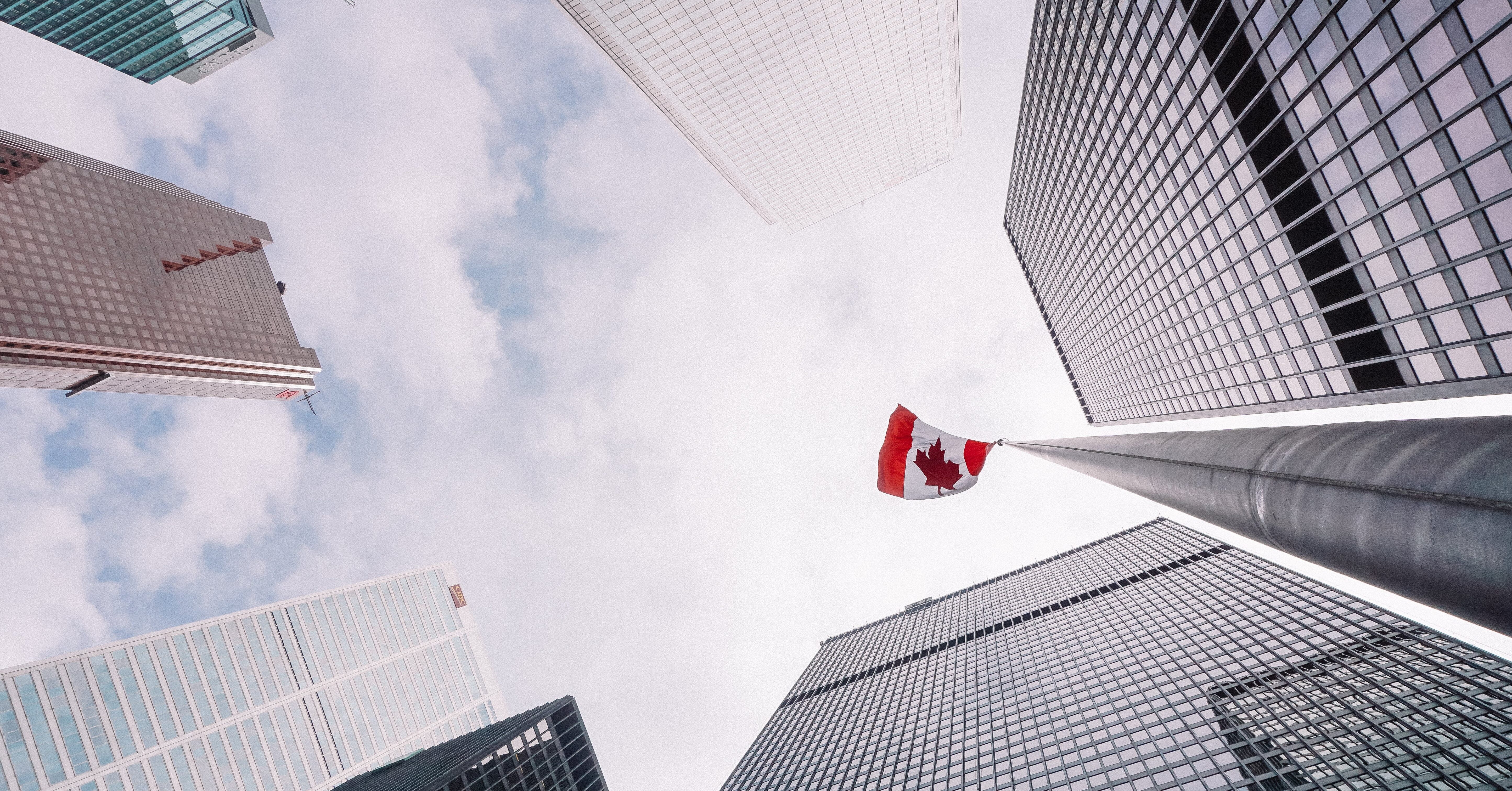
Discover the strategic advantage for U.S. banks embracing innovation with cost-effective Canadian nearshore IT support.
These are not your grandmother’s models: the impact of LLM’s on Document Processing
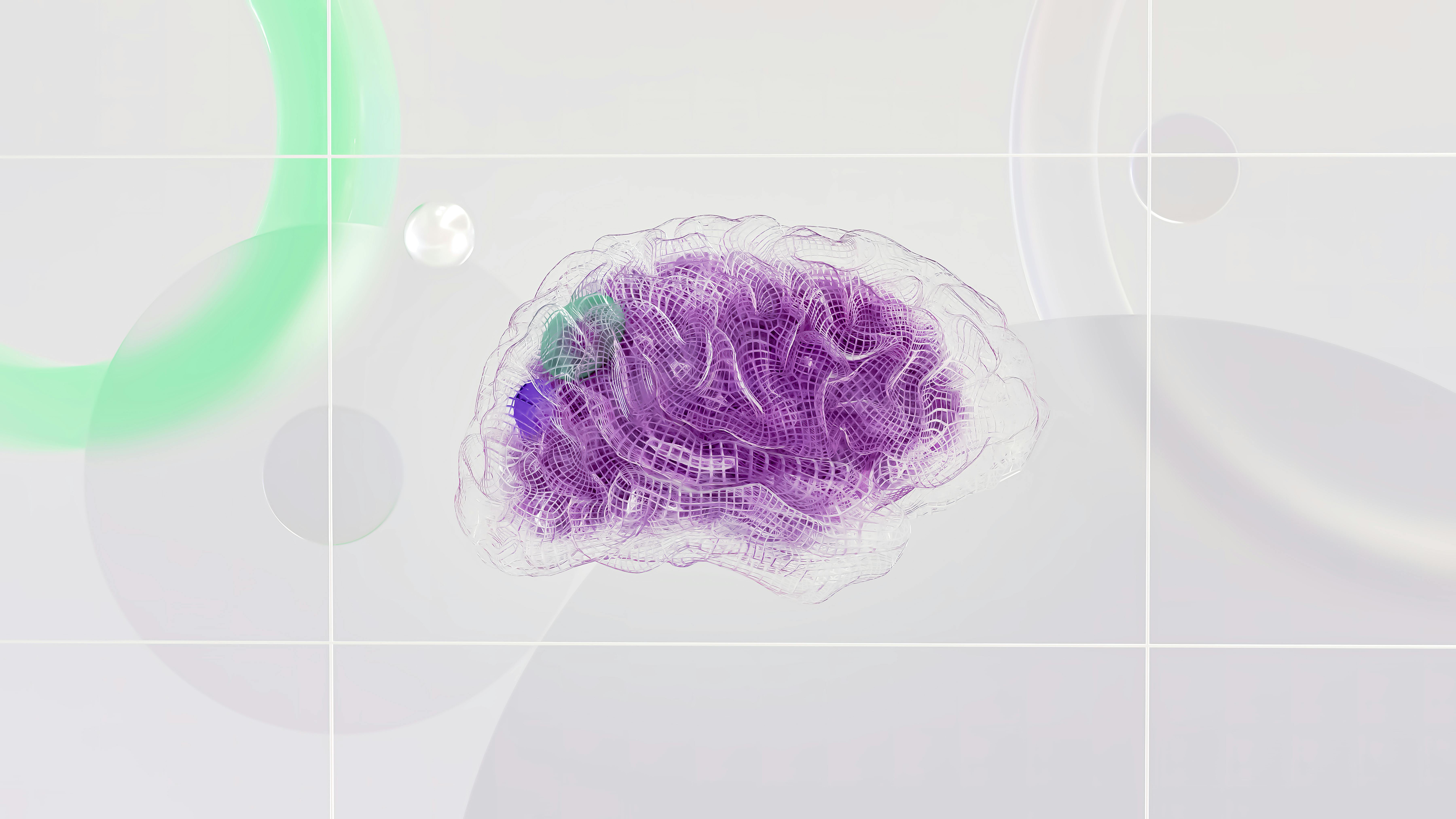
Explore the transformative influence of large language models (LLMs) on document processing in this insightful article. Discover how these cutting-edge models are reshaping traditional approaches, unlocking new possibilities in data analysis, and revolutionizing the way we interact with information.
From Chaos to Clarity: Achieving Operational Excellence through Business Process Improvement
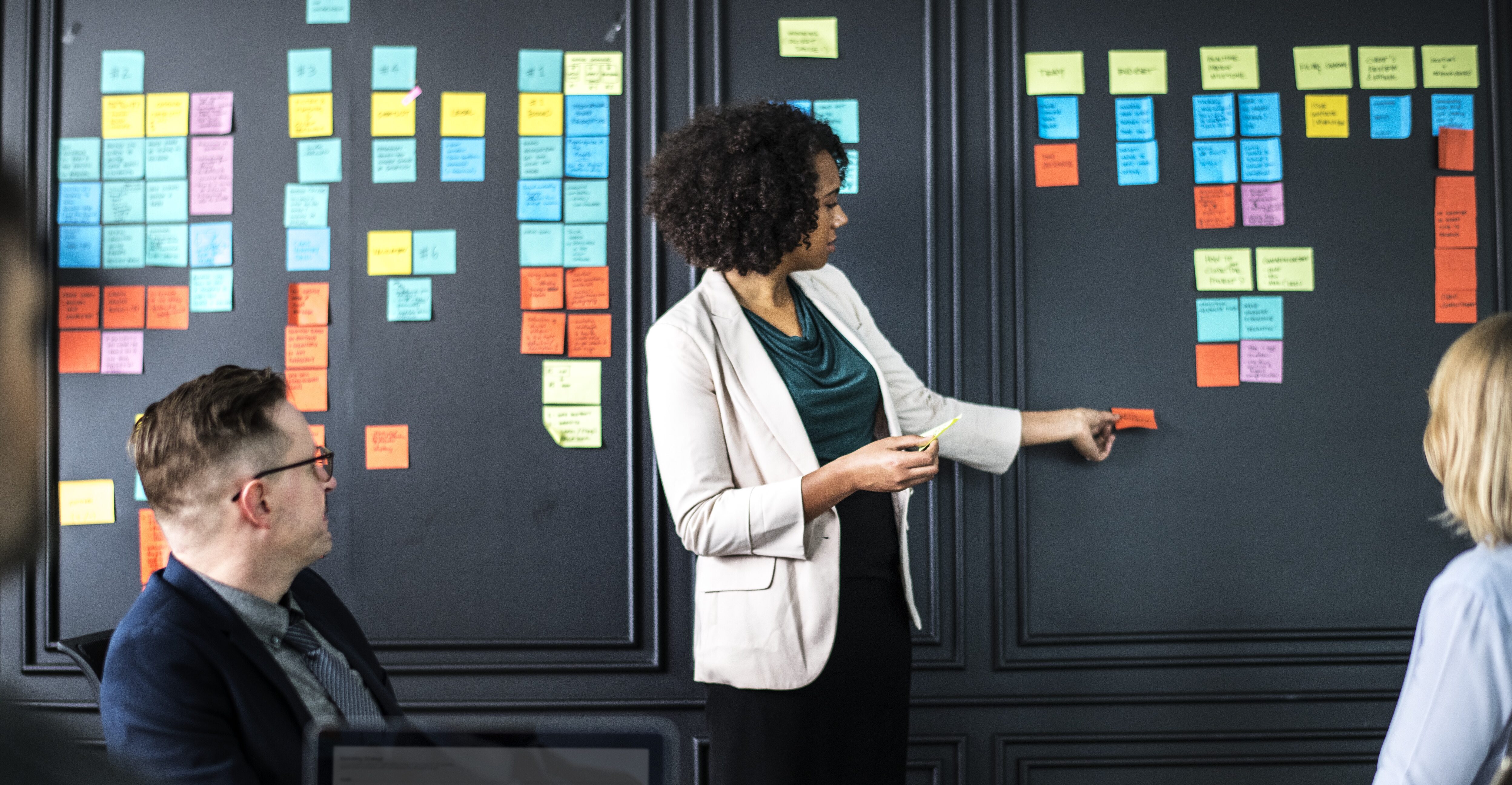
Discover transformative insights and strategies to streamline operations, enhance efficiency, and drive success.
Finding the right API Management Platform
5 Factors to Evaluate Open Banking Readiness in Canada
Open Banking in Canada: How Banks and Customers Can Benefit
4 Ways APIs Can Improve Your Bank
Challenges in Digital Lending
Lenders Transformation Playbook: Bridging Strategy and Execution
AI’s Mid-Market Makeover in Financial Services
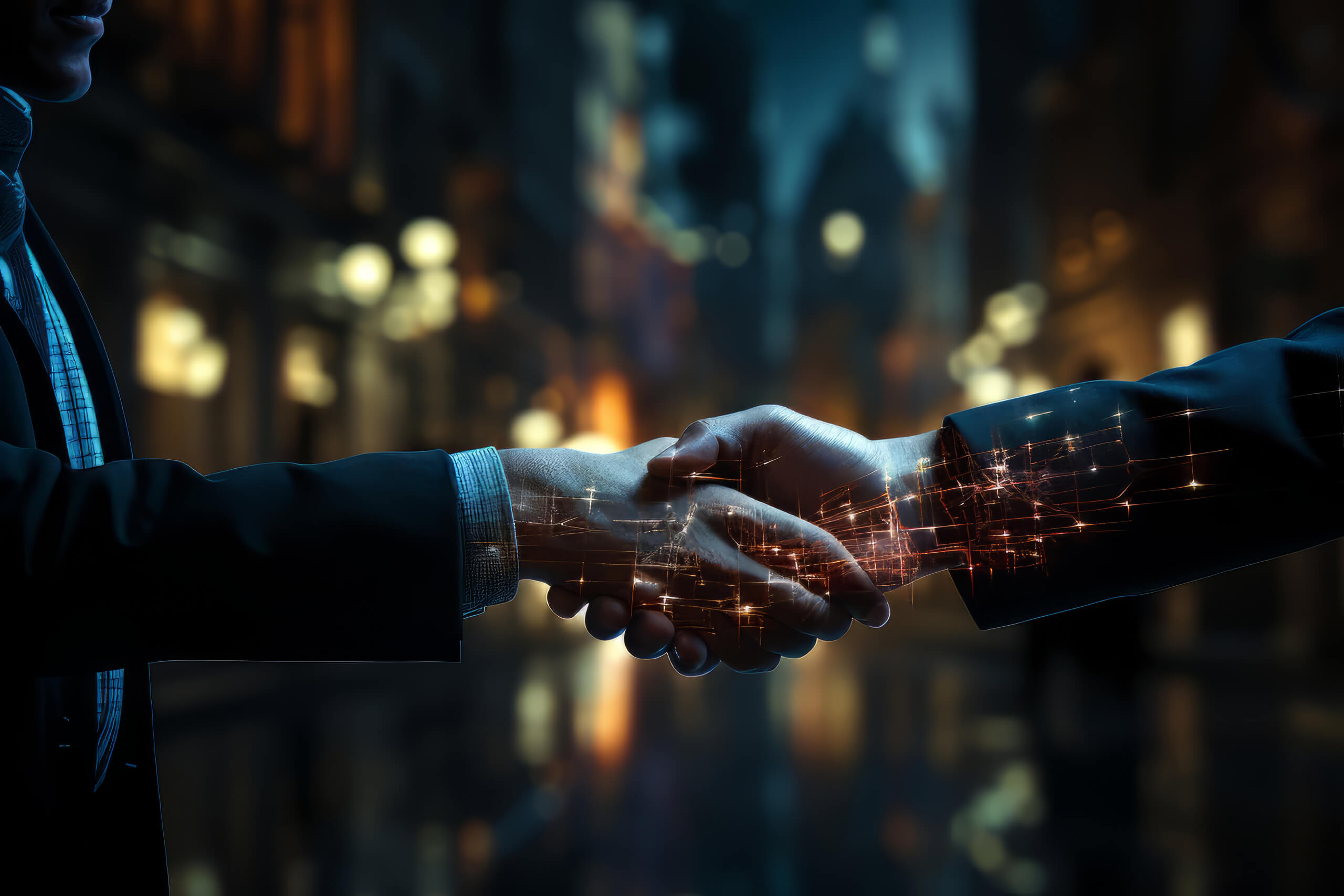
Mid-sized financial services institutions (FIs) are facing significant challenges during this period of rapid technological change, particularly with the rise of artificial intelligence (AI). As customer expectations grow, smaller banks and lenders must stay competitive and responsive. Canada’s largest financial institutions are already advancing in AI, while many others remain in ‘observer’ mode, hesitant to invest and experiment. Yet, mid-sized FIs that adopt the right strategy have unique agility, allowing them to adapt swiftly and efficiently to technological disruptions—even more so than their larger counterparts.
Process Improvement and Automation Support the Mission at Trez Capital 🚀
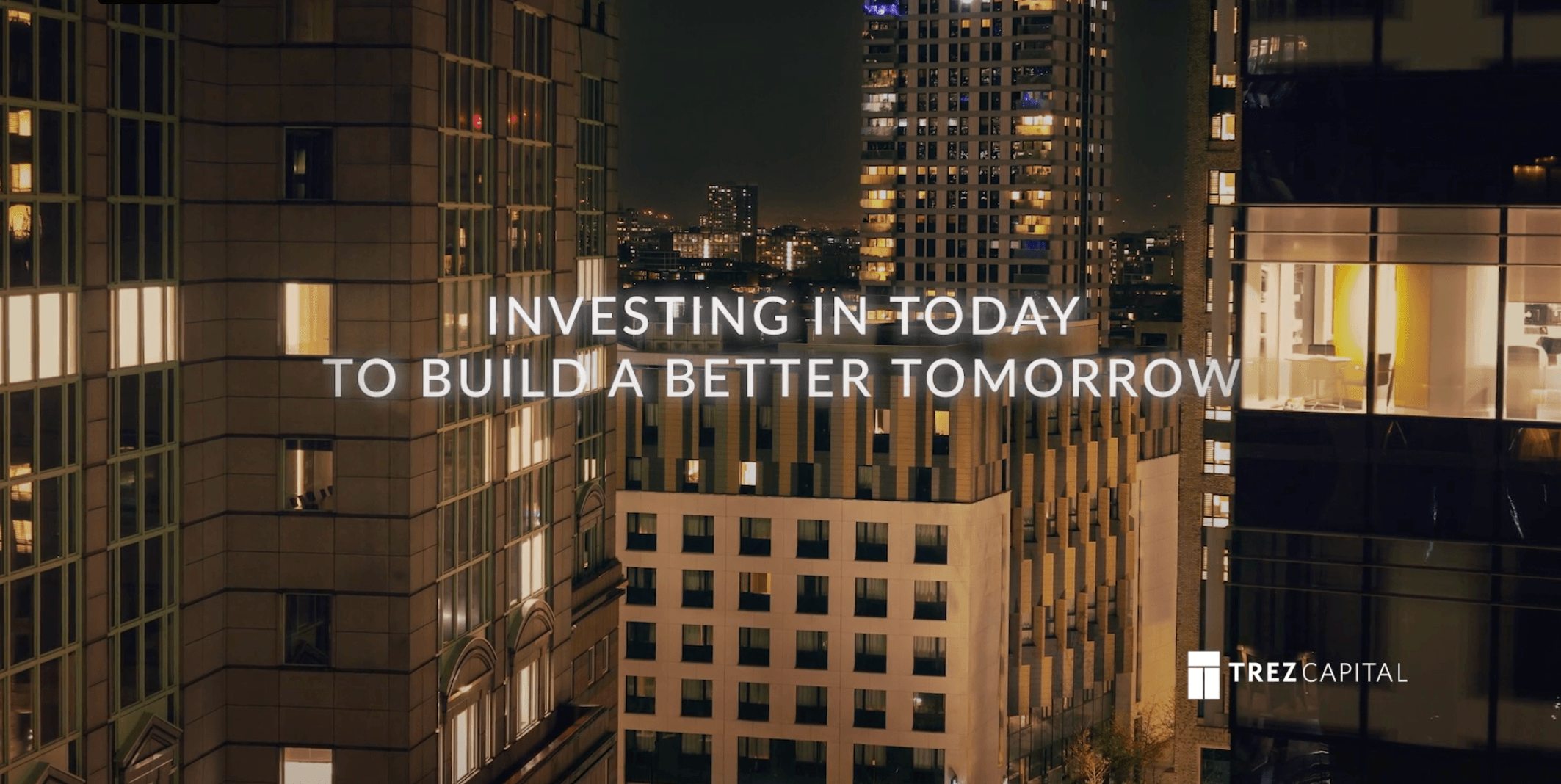
Trez distributes capital based on very specific criteria. But with over 300 investments in their portfolio, they process numerous payment requests and deal with documents in varied data formats. They saw an opportunity to enhance efficiency, improve task management, and better utilize data insights for strategic decision-making.
Align, Assemble, Assure: A Framework for AI Adoption
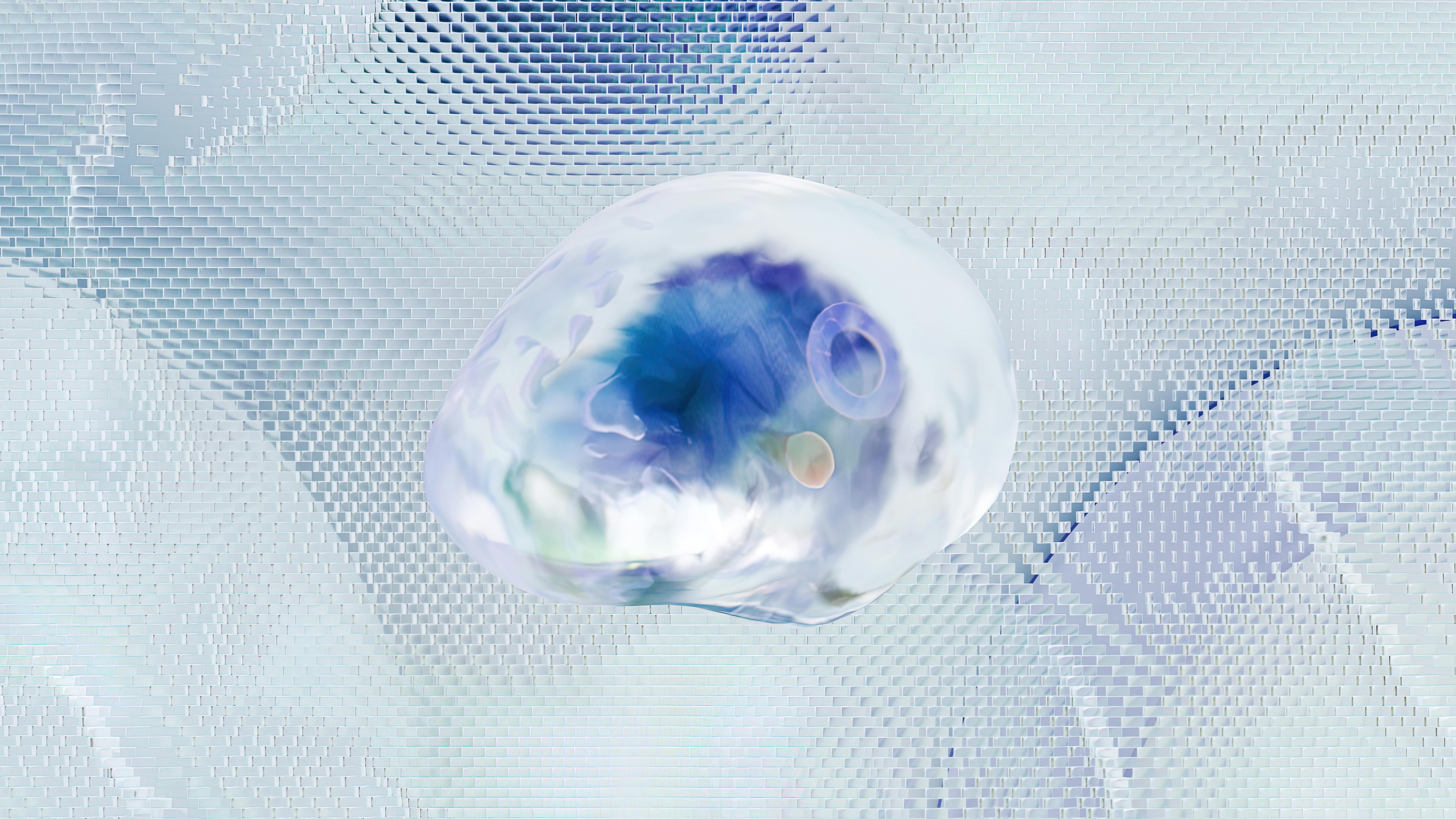
An in-depth guide for adopting and scaling AI in the enterprise using actionable and measurable steps.
Blanc Labs Welcomes Two New Leaders to Advance AI Innovation and Enhance Tech Advisory Services for Financial Institutions Across North America
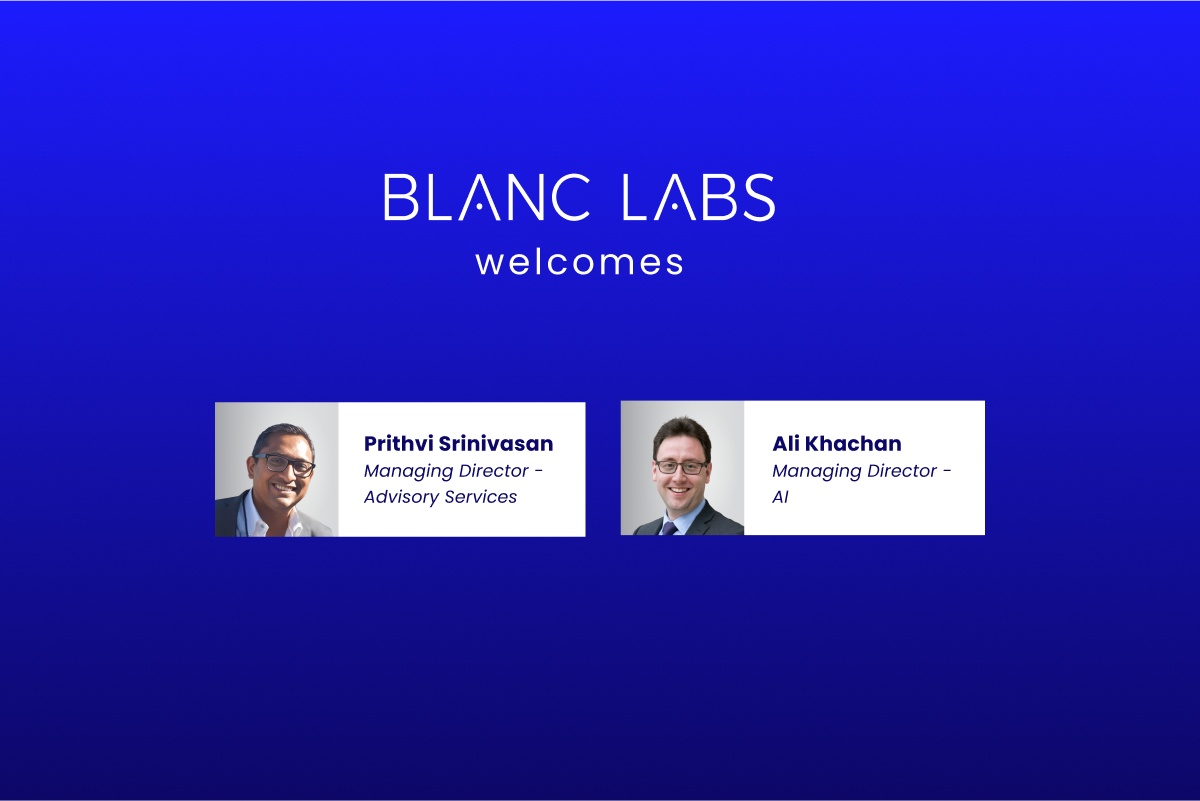
Blanc Labs and TCG Process have partnered to transform lending operations with innovative automation solutions, using the DocProStar platform to enhance efficiency, compliance, and customer satisfaction in the Canadian lending market.
Blanc Labs Partners with TCG Process to Integrate their Automation and Orchestration Platform and deliver Advanced Intelligent Workflow Automation to Financial Institutions
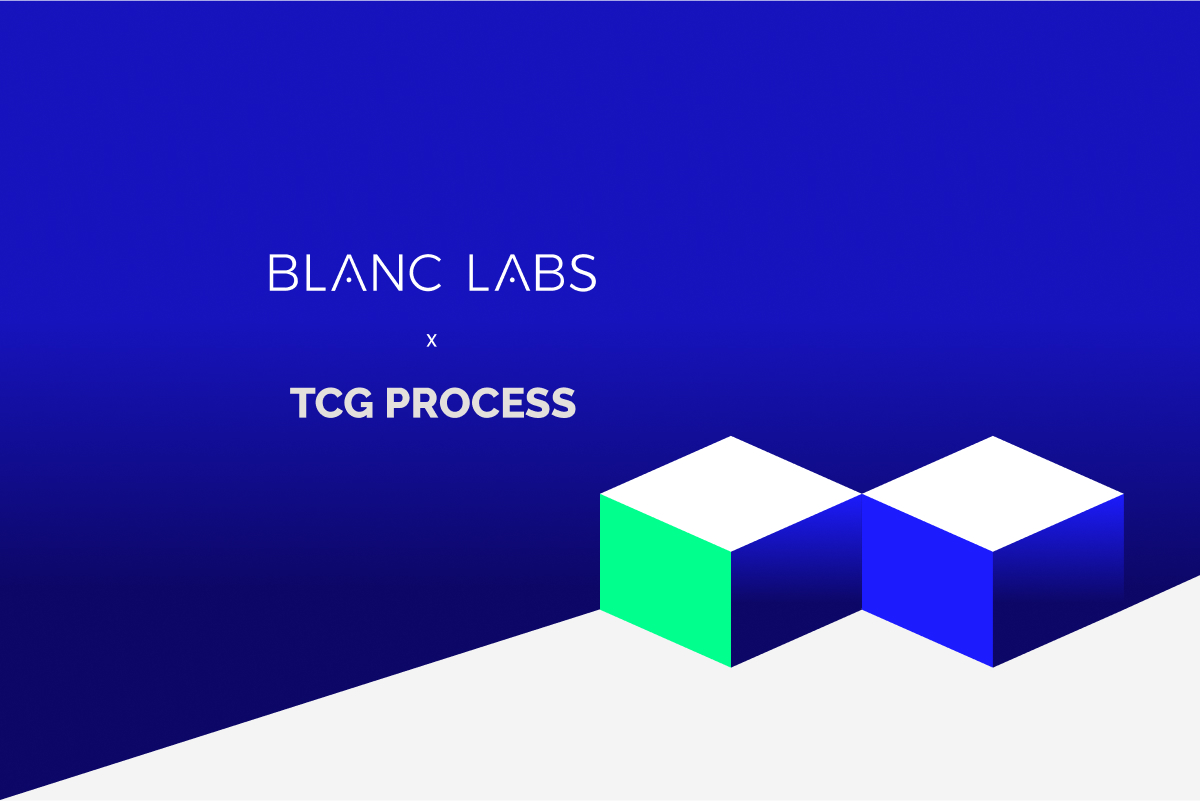
Blanc Labs and TCG Process have partnered to transform lending operations with innovative automation solutions, using the DocProStar platform to enhance efficiency, compliance, and customer satisfaction in the Canadian lending market.
BPI in Banking and Financial Services in the US & Canada
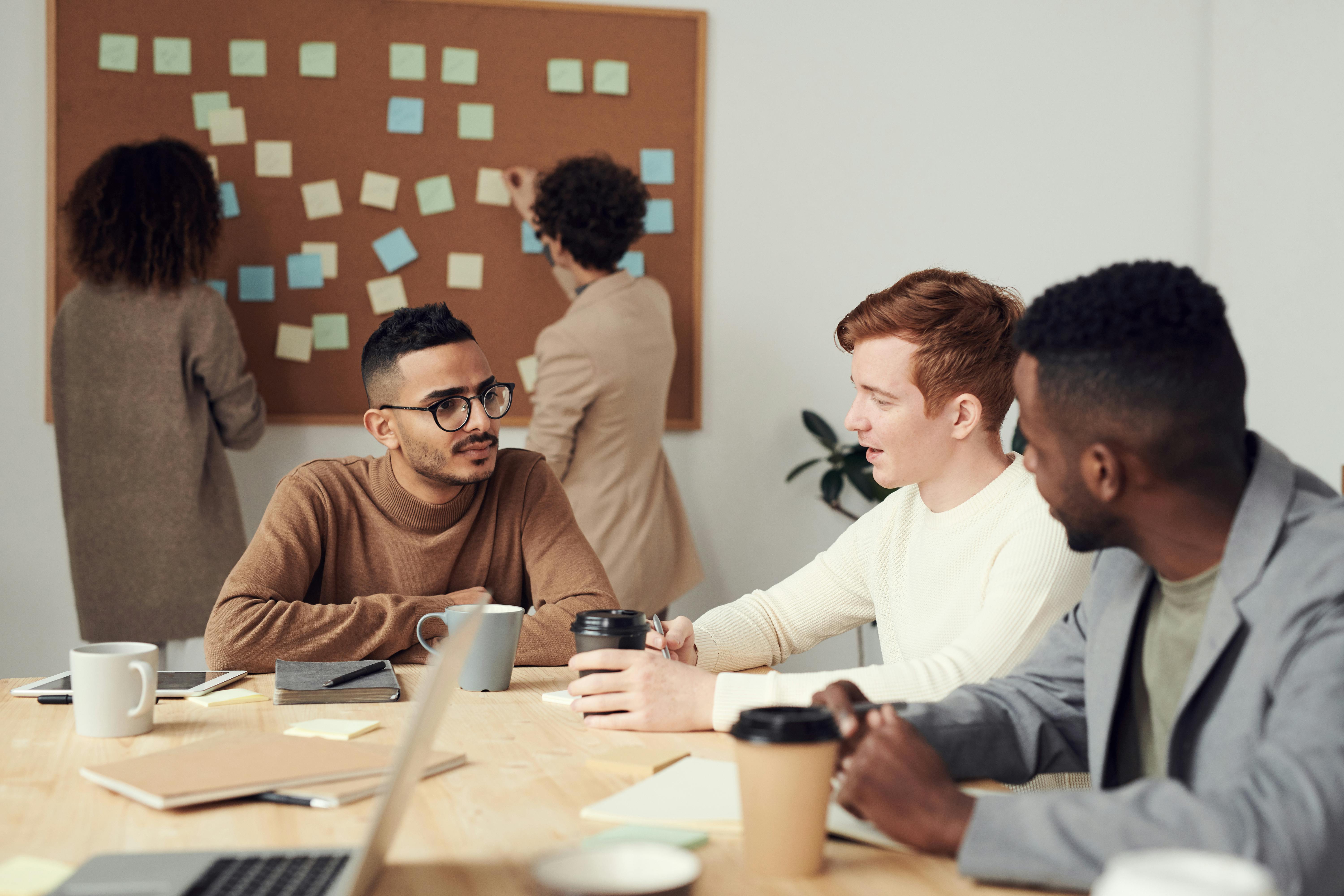
Banking and financial services are changing fast. Moving from old, paper methods to new, digital ones is key to staying in business. It’s important to think about how business process improvement (BPI) can help.
Business Process Improvement vs Business Process Reengineering
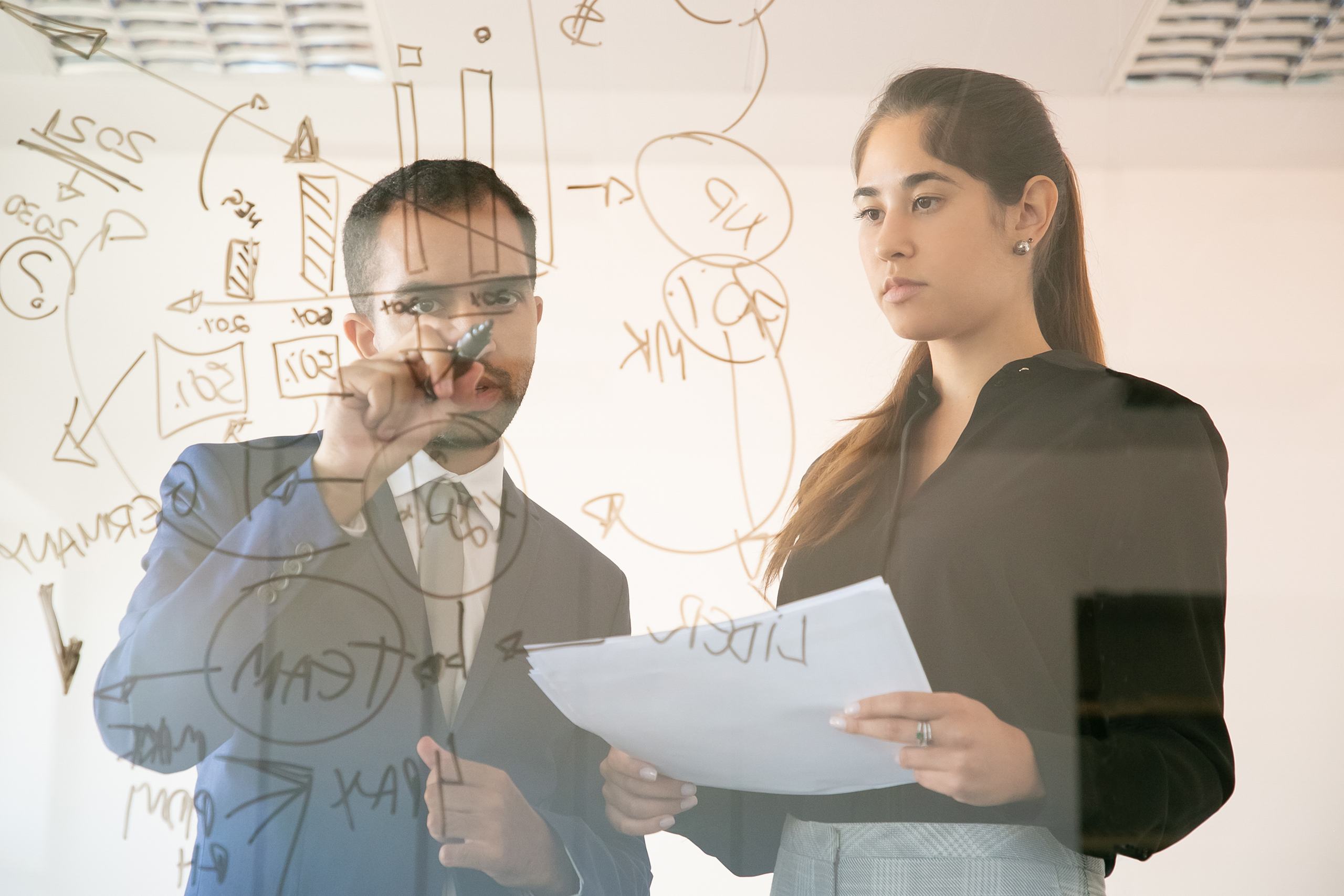
Business process improvement vs. reengineering is a tough choice. In this guide, we help you choose between the two based on four factors.
What is the role of a Business Process Improvement Specialist?
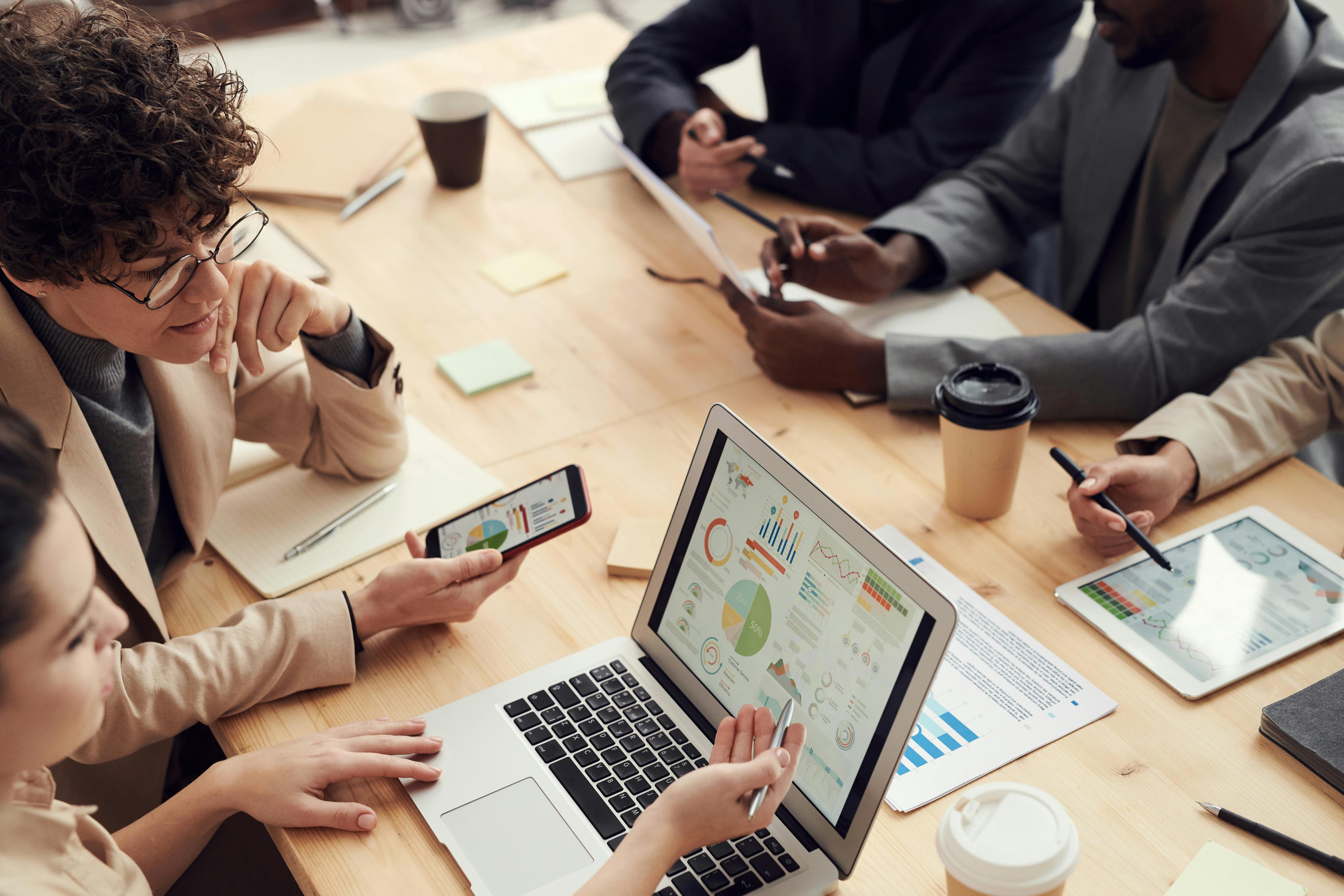
A business process improvement specialist identifies bottlenecks and inefficiencies in your workflows, allowing you to focus efforts on automating the right processes.
Open Banking Technology Architecture Whitepaper
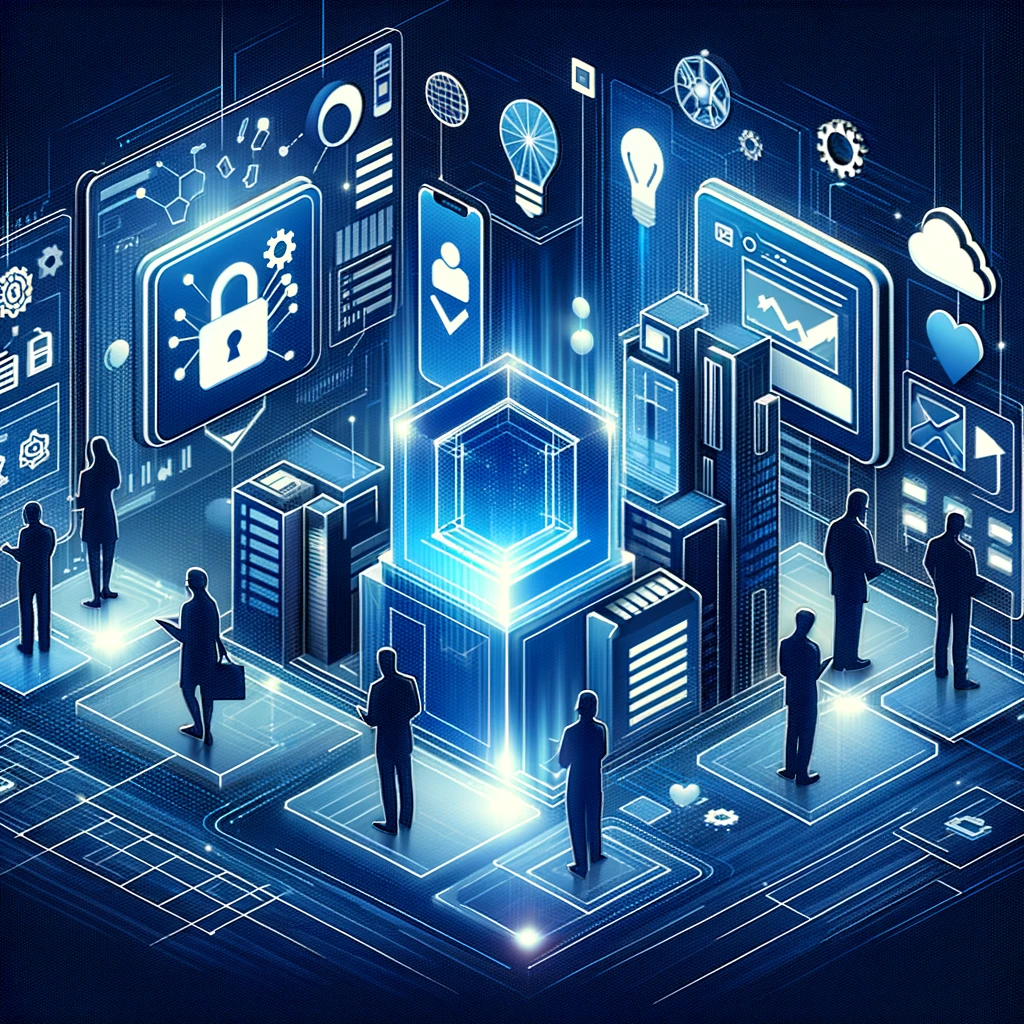
We’ve developed this resource to help technical teams adopt an Open Banking approach by explaining a high-level solution architecture that is organization agnostic.
Winning the Open Banking Race: A Challenger’s Path to Entering the Ecosystem Economy
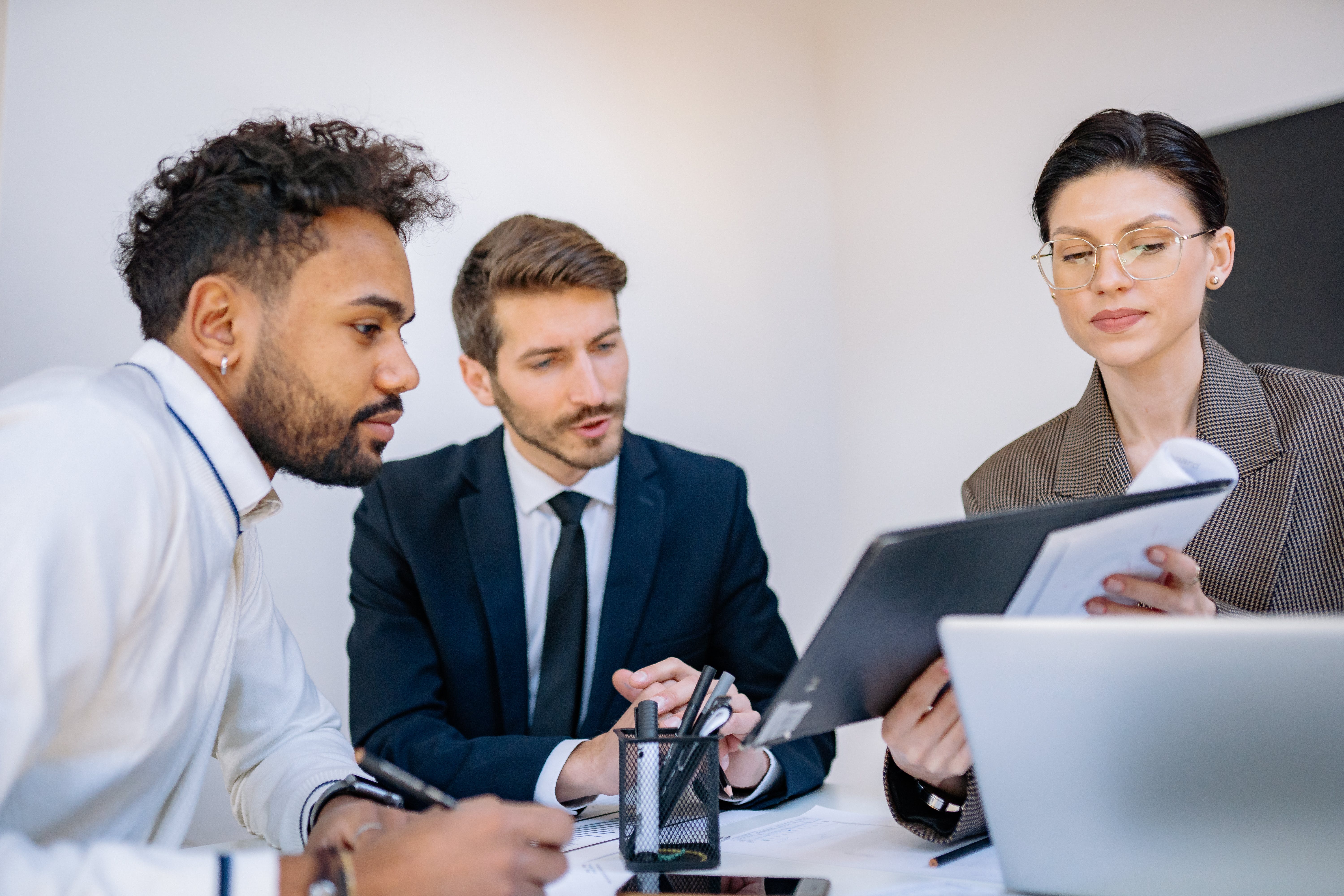
Learn about the steps you can take in forming an open banking strategy and executing on it.
Canadian IT services firms offer a strategic opportunity for US Banks and FIs
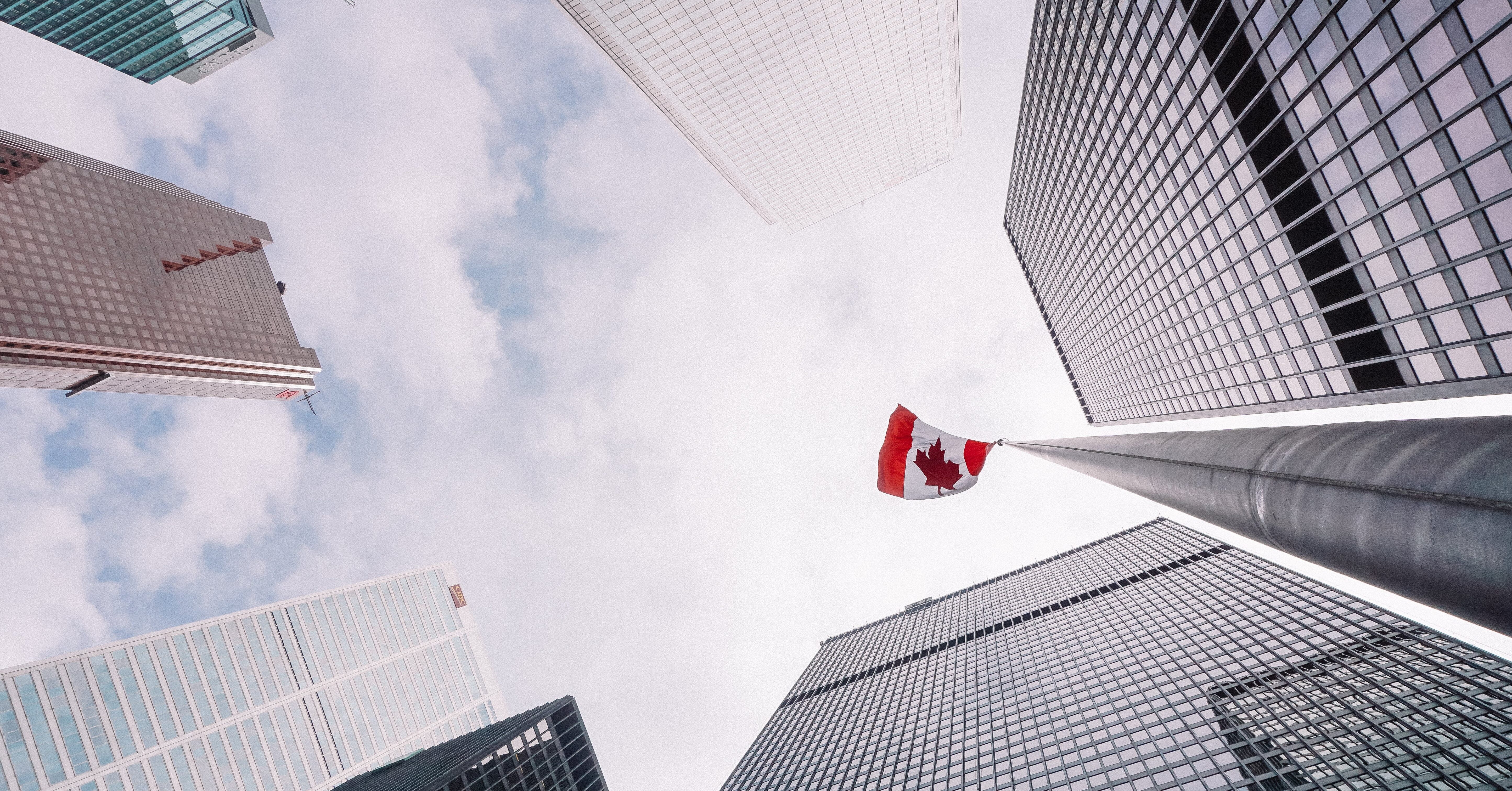
Discover the strategic advantage for U.S. banks embracing innovation with cost-effective Canadian nearshore IT support.
These are not your grandmother’s models: the impact of LLM’s on Document Processing
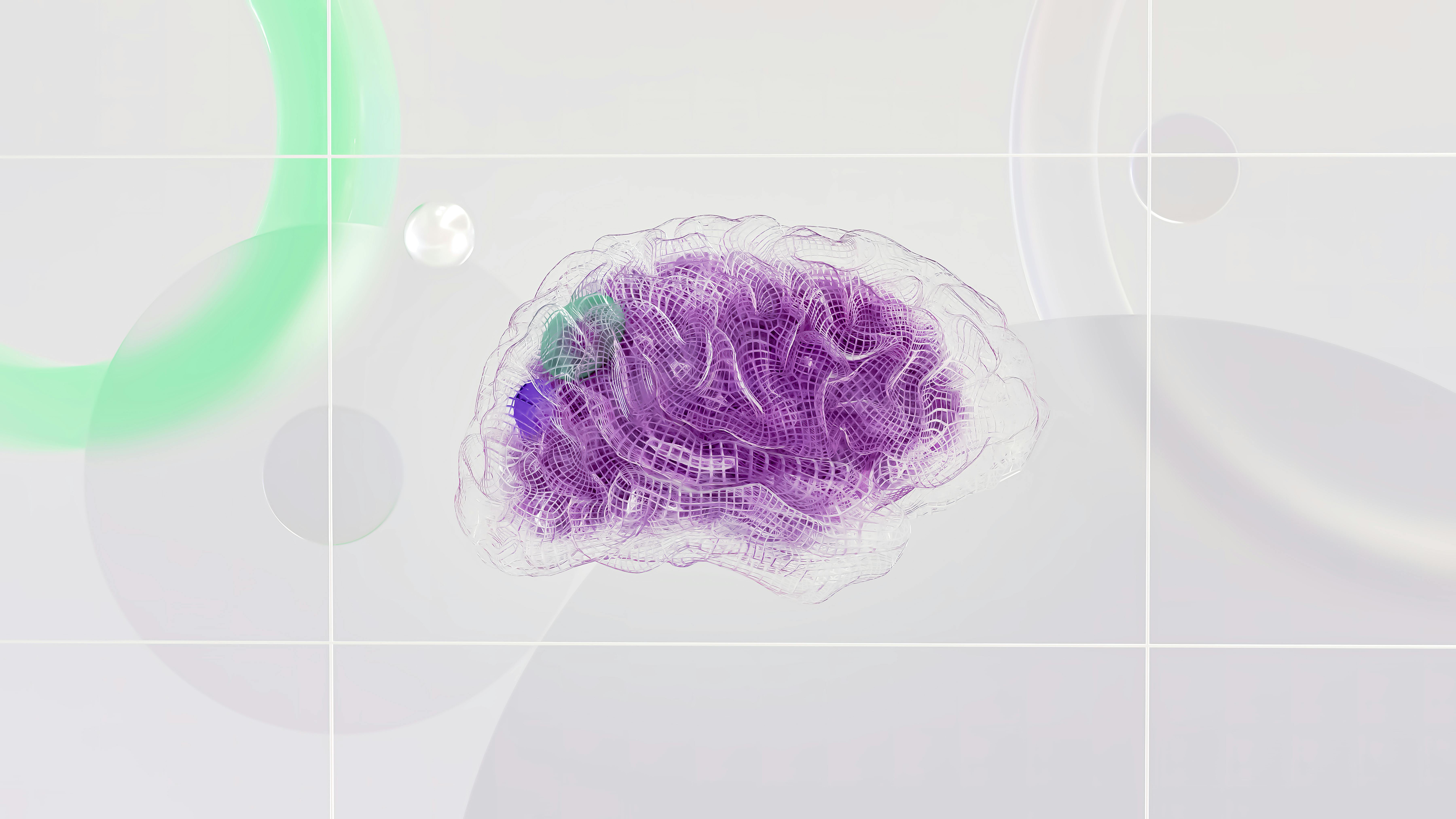
Explore the transformative influence of large language models (LLMs) on document processing in this insightful article. Discover how these cutting-edge models are reshaping traditional approaches, unlocking new possibilities in data analysis, and revolutionizing the way we interact with information.
From Chaos to Clarity: Achieving Operational Excellence through Business Process Improvement
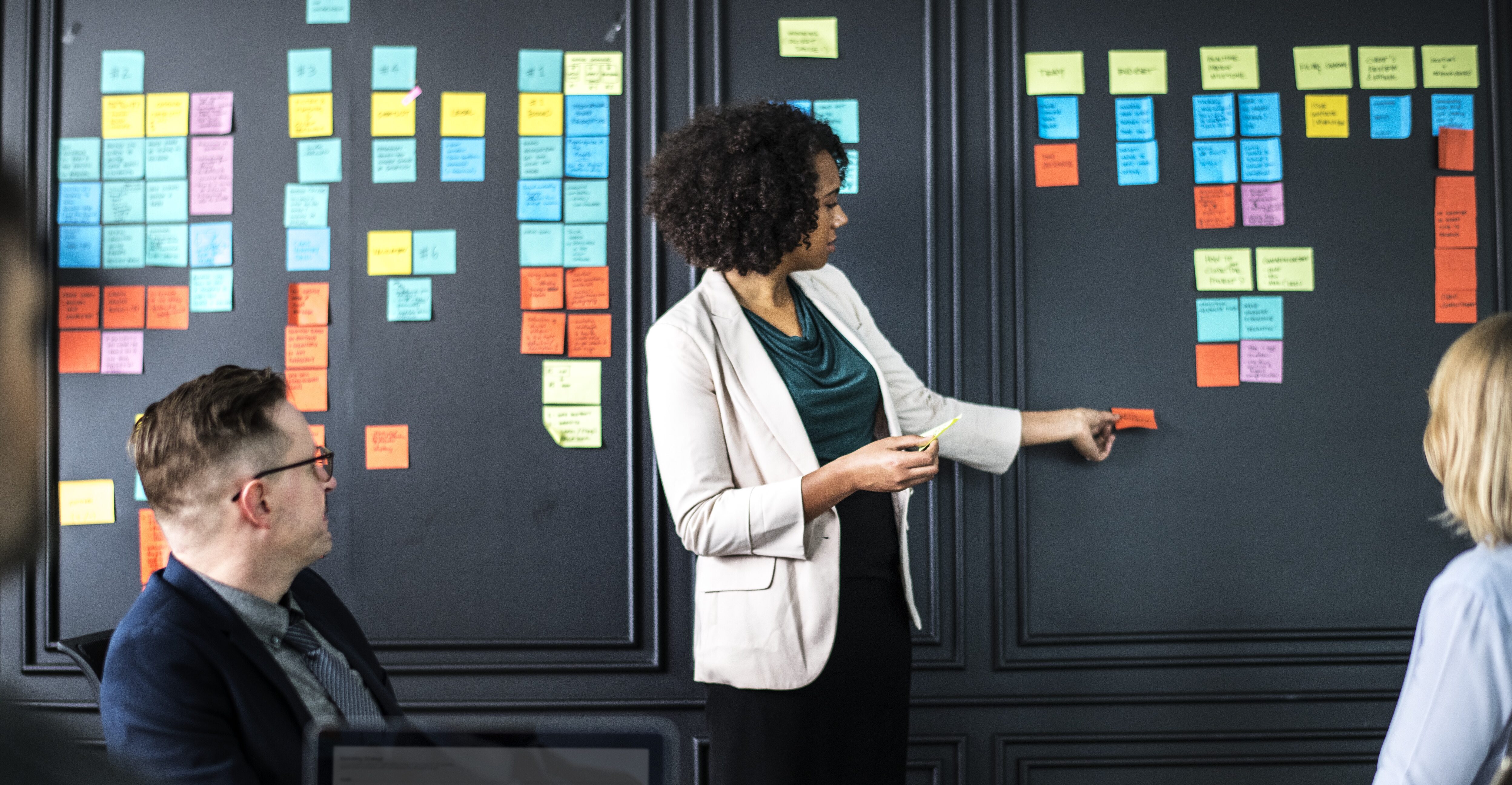
Discover transformative insights and strategies to streamline operations, enhance efficiency, and drive success.
Finding the right API Management Platform
5 Factors to Evaluate Open Banking Readiness in Canada
Open Banking in Canada: How Banks and Customers Can Benefit
4 Ways APIs Can Improve Your Bank
Challenges in Digital Lending
Lenders Transformation Playbook: Bridging Strategy and Execution
AI’s Mid-Market Makeover in Financial Services
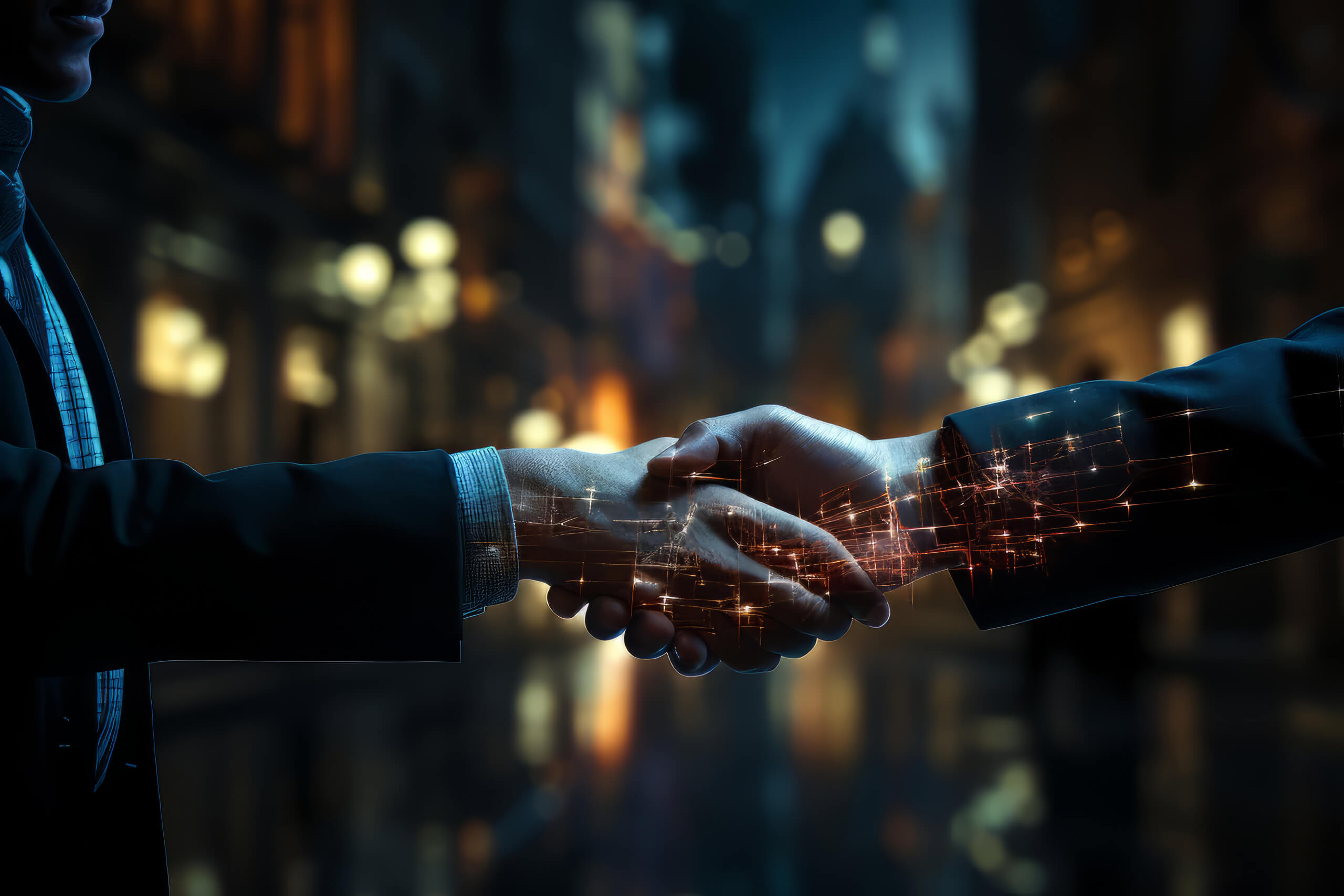
Mid-sized financial services institutions (FIs) are facing significant challenges during this period of rapid technological change, particularly with the rise of artificial intelligence (AI). As customer expectations grow, smaller banks and lenders must stay competitive and responsive. Canada’s largest financial institutions are already advancing in AI, while many others remain in ‘observer’ mode, hesitant to invest and experiment. Yet, mid-sized FIs that adopt the right strategy have unique agility, allowing them to adapt swiftly and efficiently to technological disruptions—even more so than their larger counterparts.
Process Improvement and Automation Support the Mission at Trez Capital 🚀
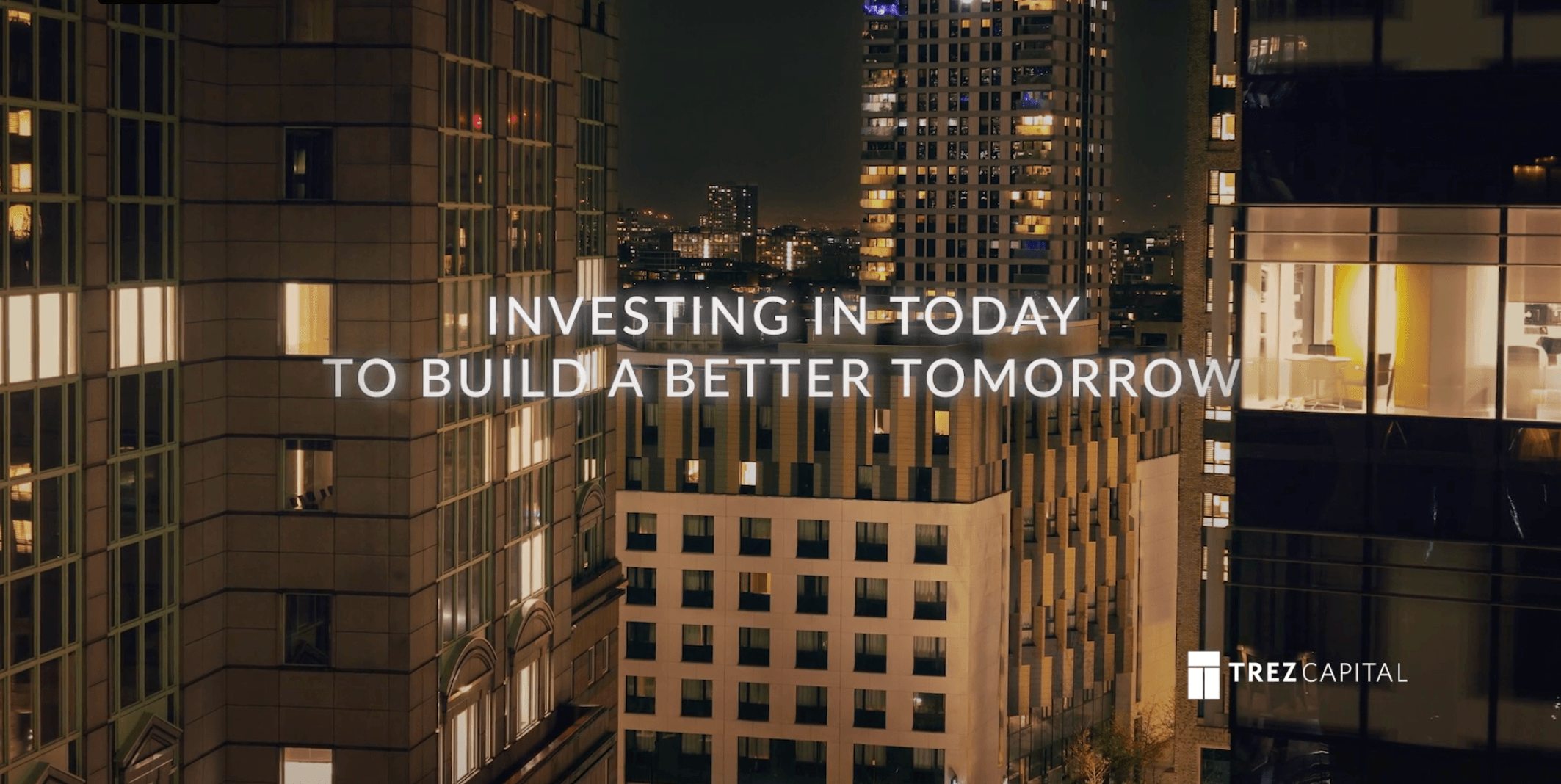
Trez distributes capital based on very specific criteria. But with over 300 investments in their portfolio, they process numerous payment requests and deal with documents in varied data formats. They saw an opportunity to enhance efficiency, improve task management, and better utilize data insights for strategic decision-making.
Align, Assemble, Assure: A Framework for AI Adoption
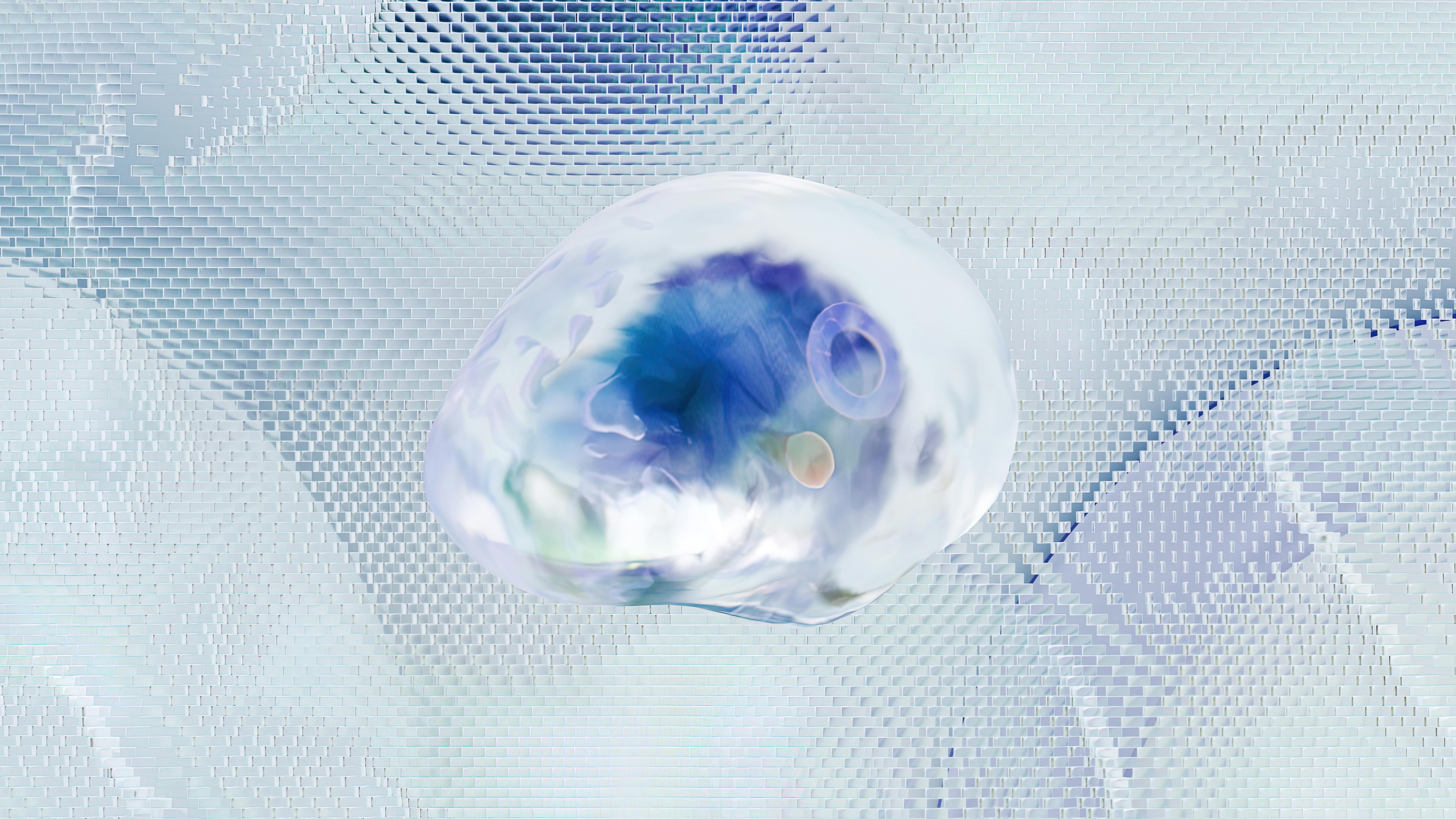
An in-depth guide for adopting and scaling AI in the enterprise using actionable and measurable steps.
Blanc Labs Welcomes Two New Leaders to Advance AI Innovation and Enhance Tech Advisory Services for Financial Institutions Across North America
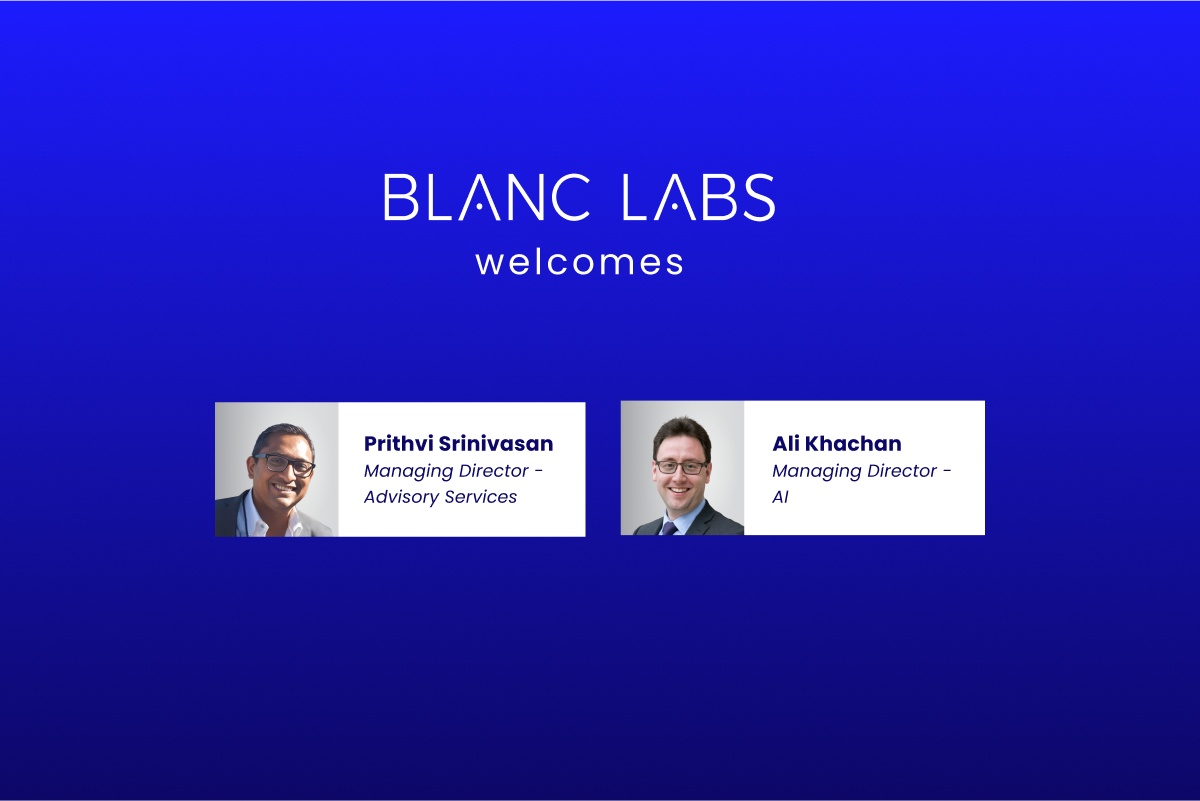
Blanc Labs and TCG Process have partnered to transform lending operations with innovative automation solutions, using the DocProStar platform to enhance efficiency, compliance, and customer satisfaction in the Canadian lending market.
Blanc Labs Partners with TCG Process to Integrate their Automation and Orchestration Platform and deliver Advanced Intelligent Workflow Automation to Financial Institutions
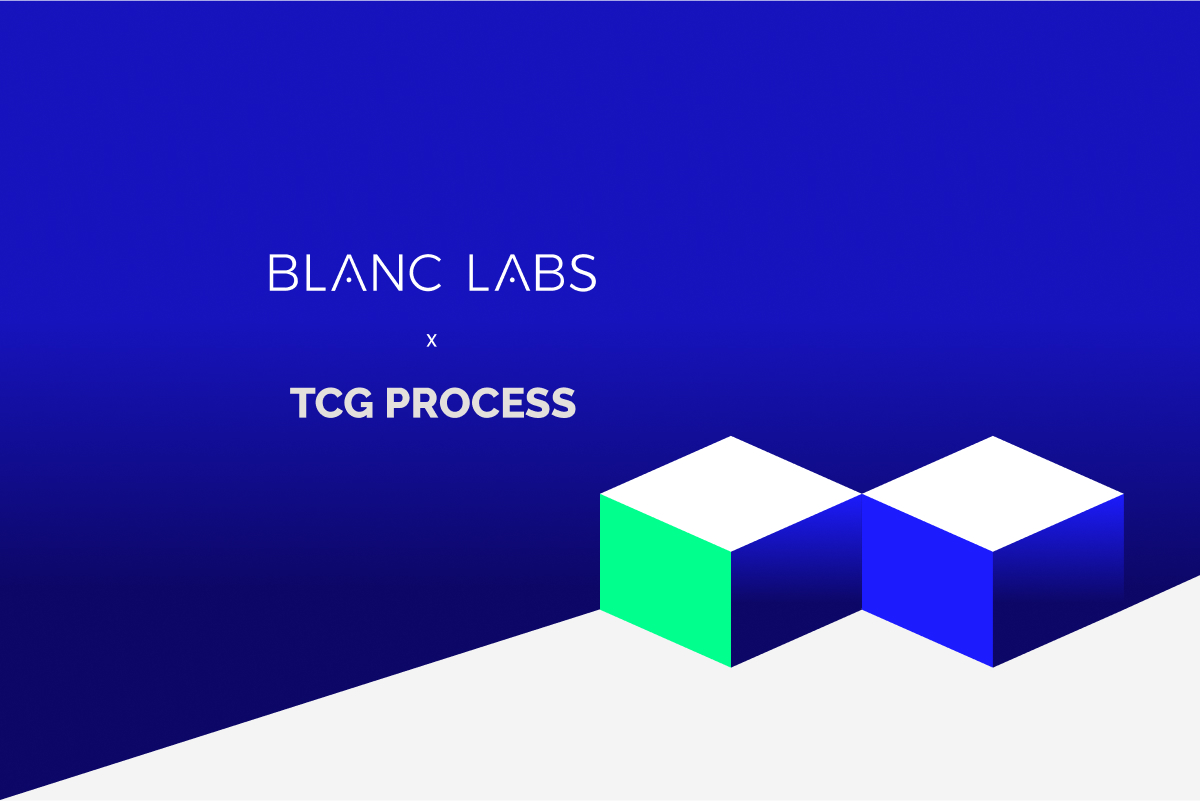
Blanc Labs and TCG Process have partnered to transform lending operations with innovative automation solutions, using the DocProStar platform to enhance efficiency, compliance, and customer satisfaction in the Canadian lending market.
BPI in Banking and Financial Services in the US & Canada
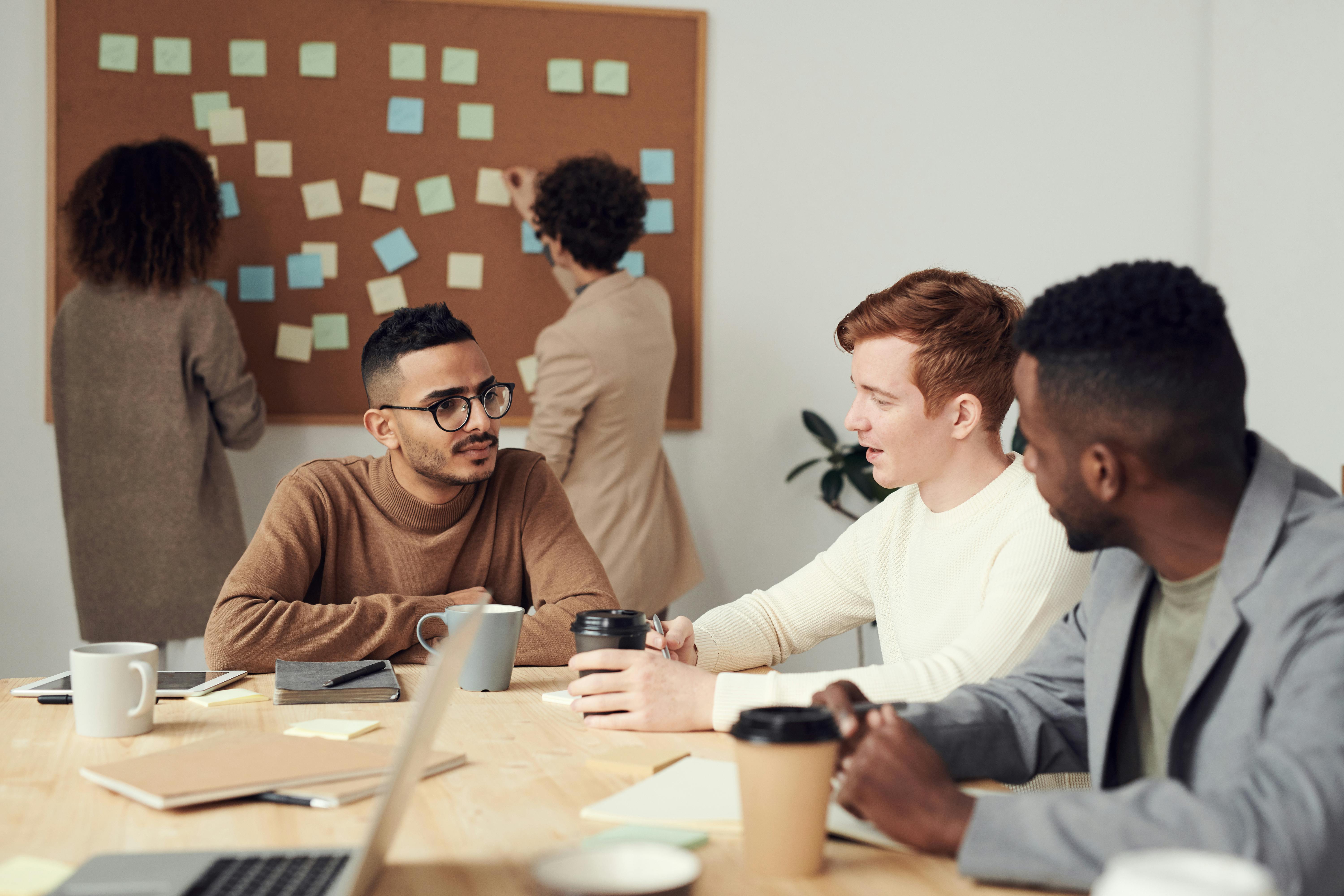
Banking and financial services are changing fast. Moving from old, paper methods to new, digital ones is key to staying in business. It’s important to think about how business process improvement (BPI) can help.
Business Process Improvement vs Business Process Reengineering
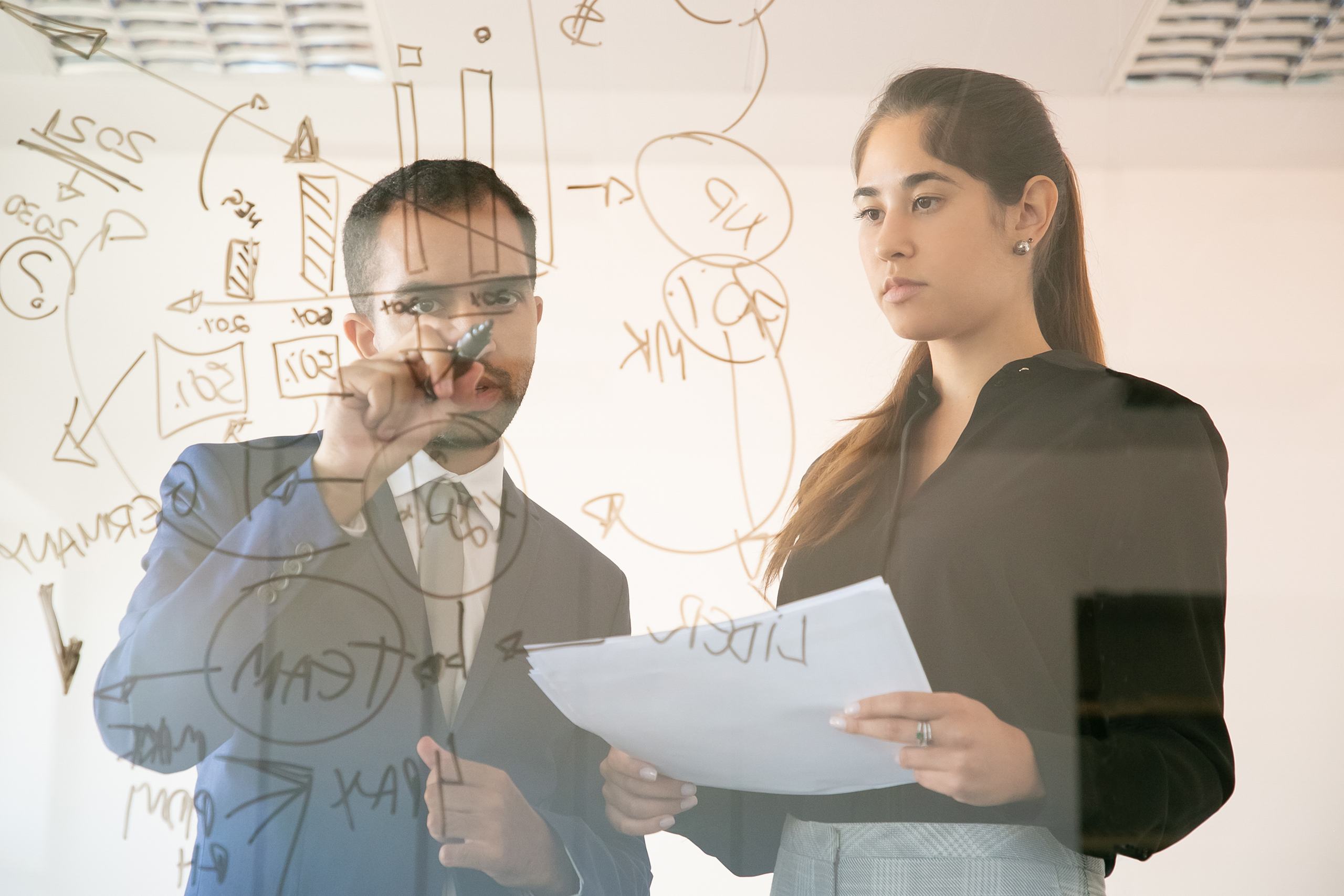
Business process improvement vs. reengineering is a tough choice. In this guide, we help you choose between the two based on four factors.
What is the role of a Business Process Improvement Specialist?
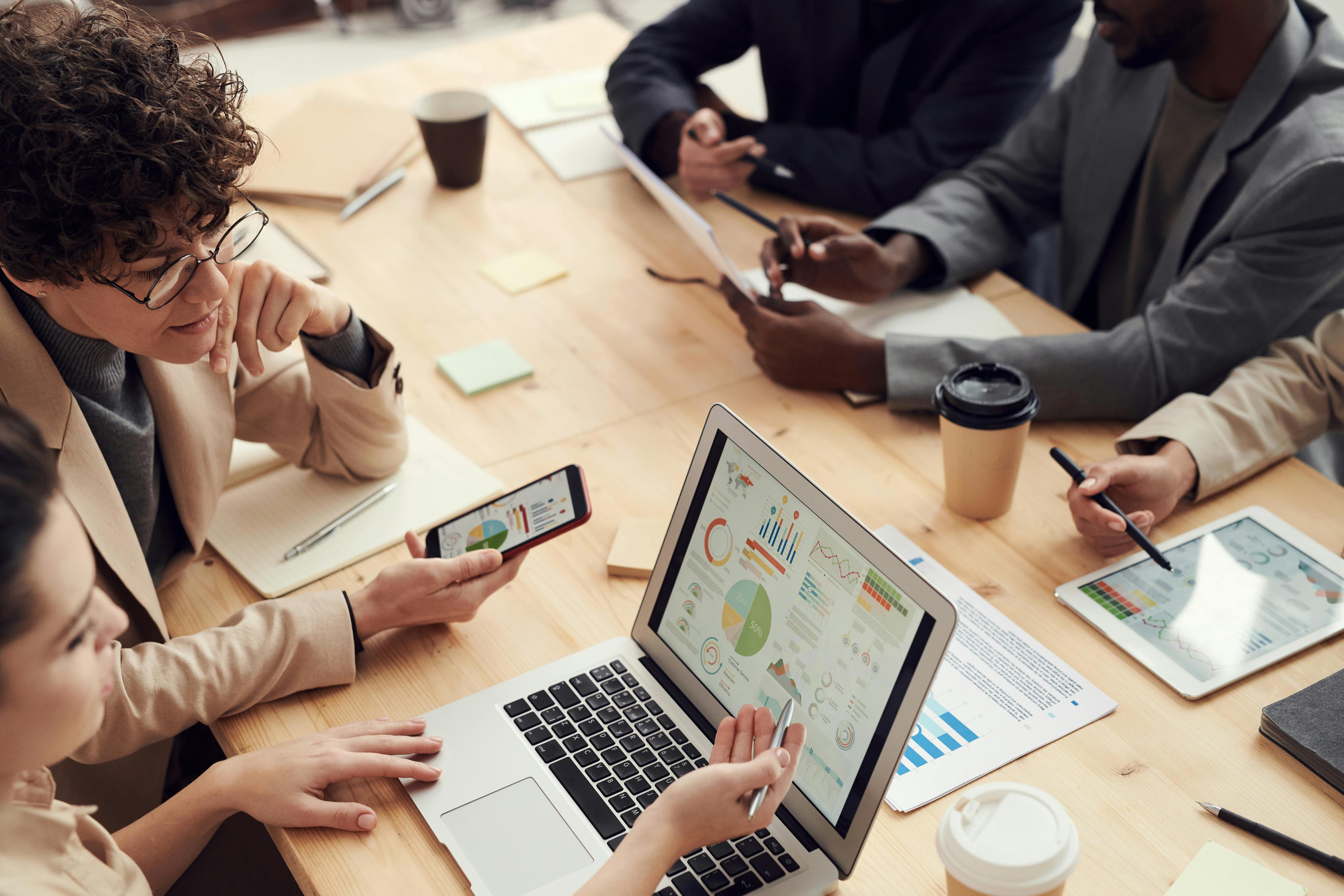
A business process improvement specialist identifies bottlenecks and inefficiencies in your workflows, allowing you to focus efforts on automating the right processes.
Open Banking Technology Architecture Whitepaper
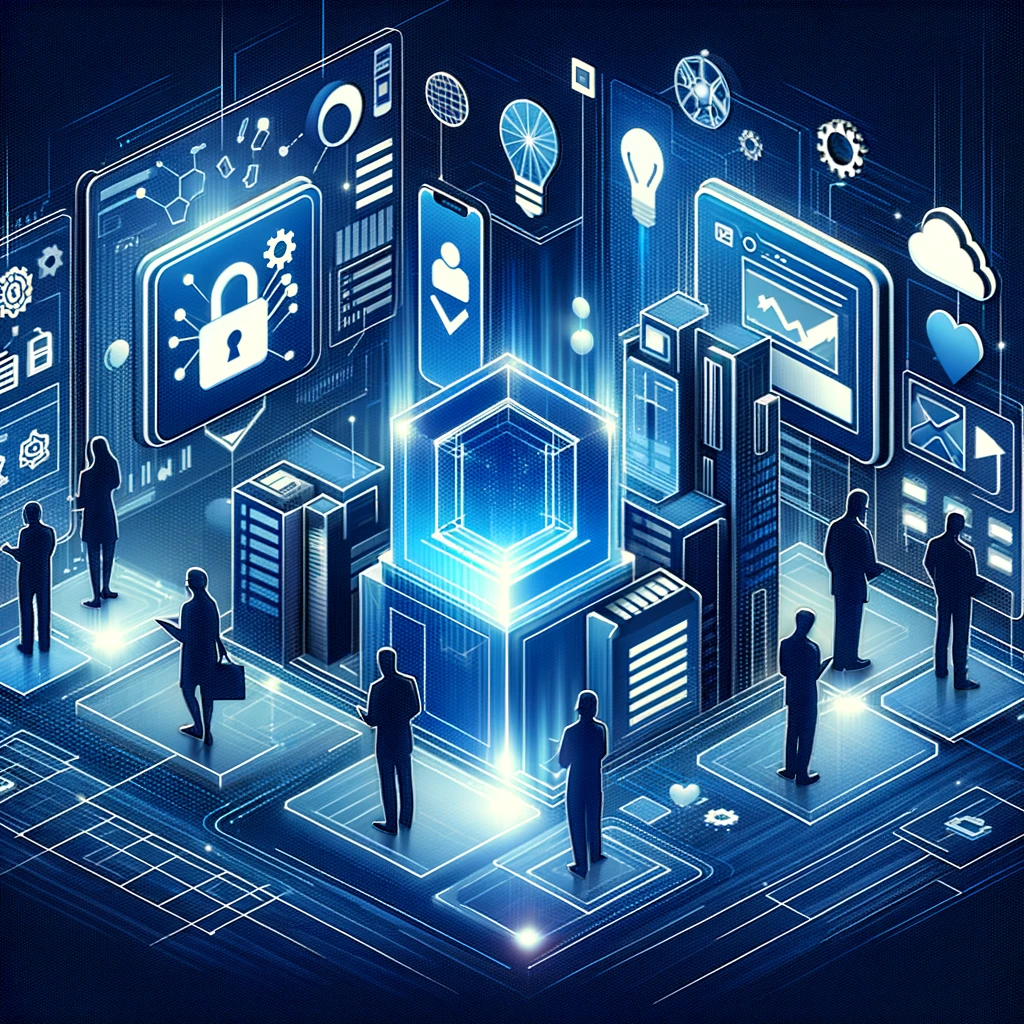
We’ve developed this resource to help technical teams adopt an Open Banking approach by explaining a high-level solution architecture that is organization agnostic.
Winning the Open Banking Race: A Challenger’s Path to Entering the Ecosystem Economy
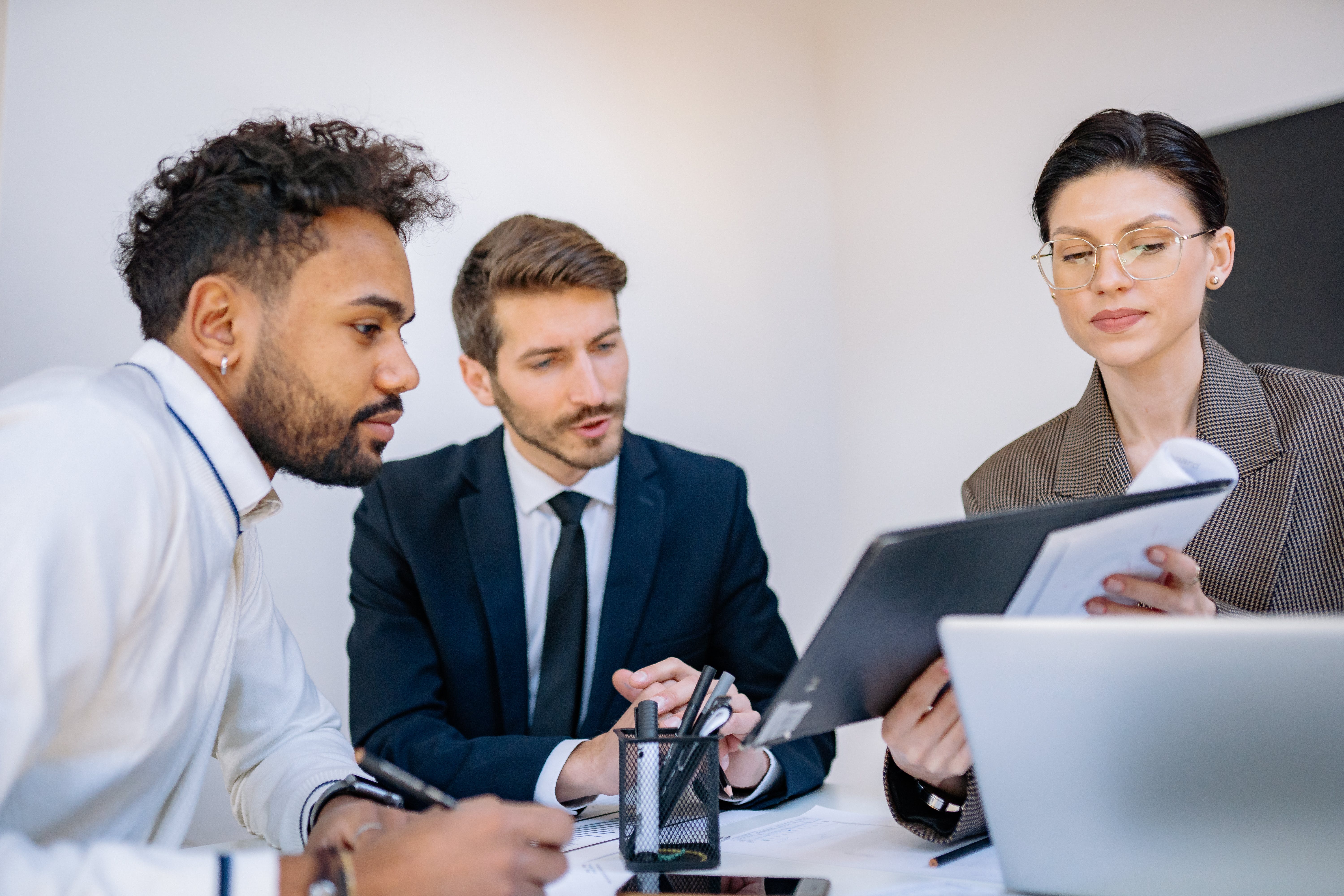
Learn about the steps you can take in forming an open banking strategy and executing on it.
Canadian IT services firms offer a strategic opportunity for US Banks and FIs
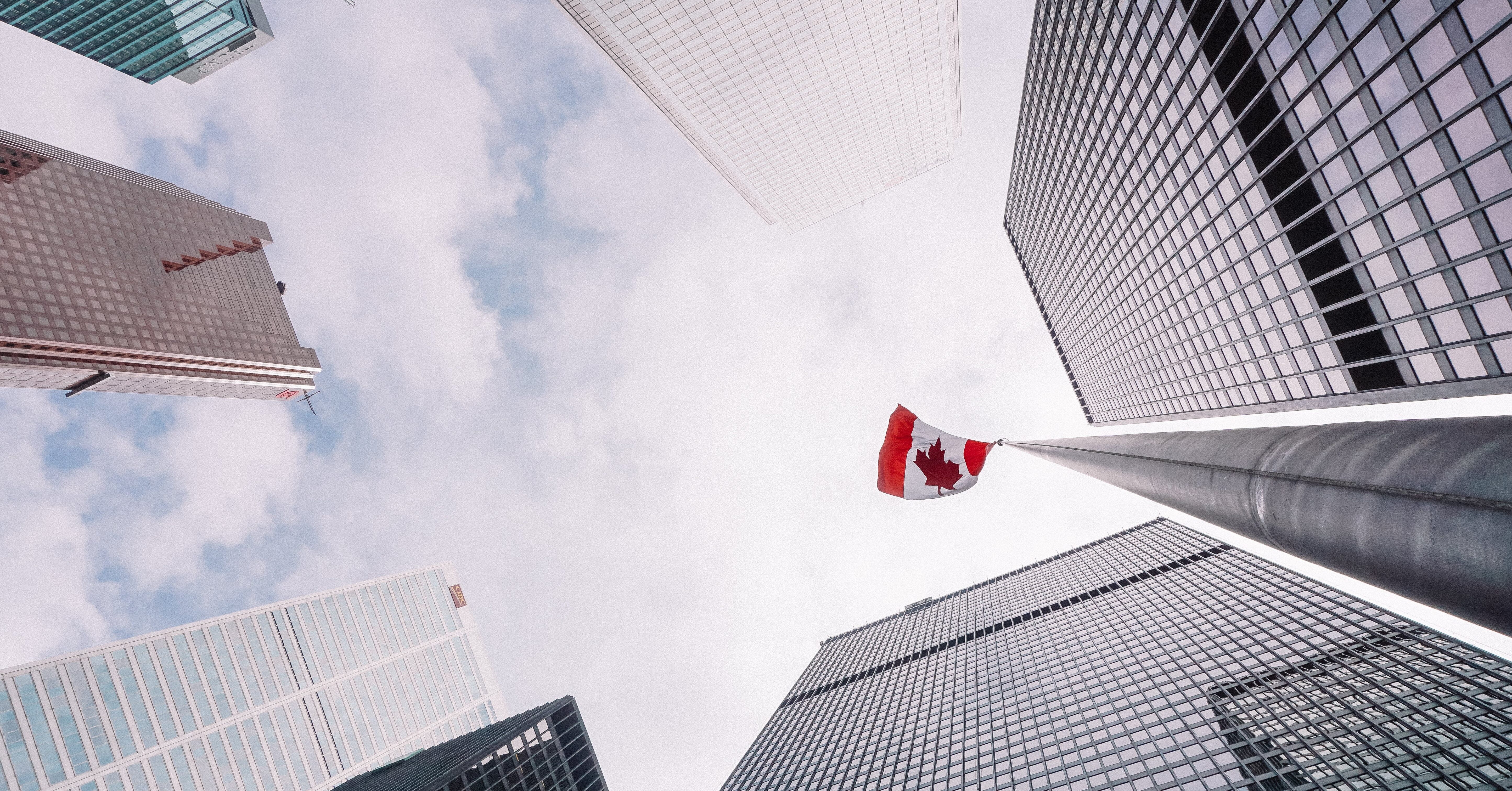
Discover the strategic advantage for U.S. banks embracing innovation with cost-effective Canadian nearshore IT support.
These are not your grandmother’s models: the impact of LLM’s on Document Processing
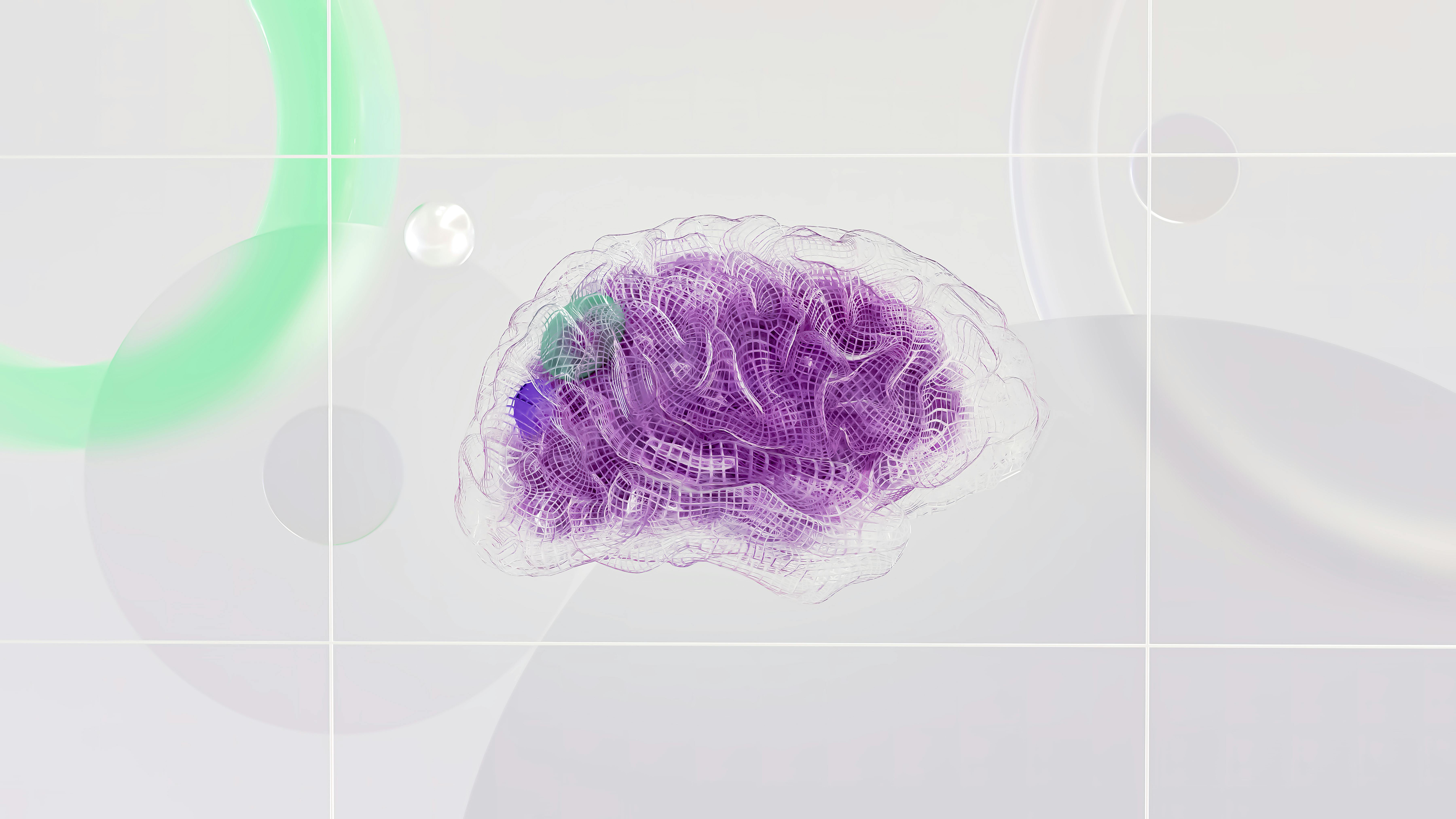
Explore the transformative influence of large language models (LLMs) on document processing in this insightful article. Discover how these cutting-edge models are reshaping traditional approaches, unlocking new possibilities in data analysis, and revolutionizing the way we interact with information.
From Chaos to Clarity: Achieving Operational Excellence through Business Process Improvement
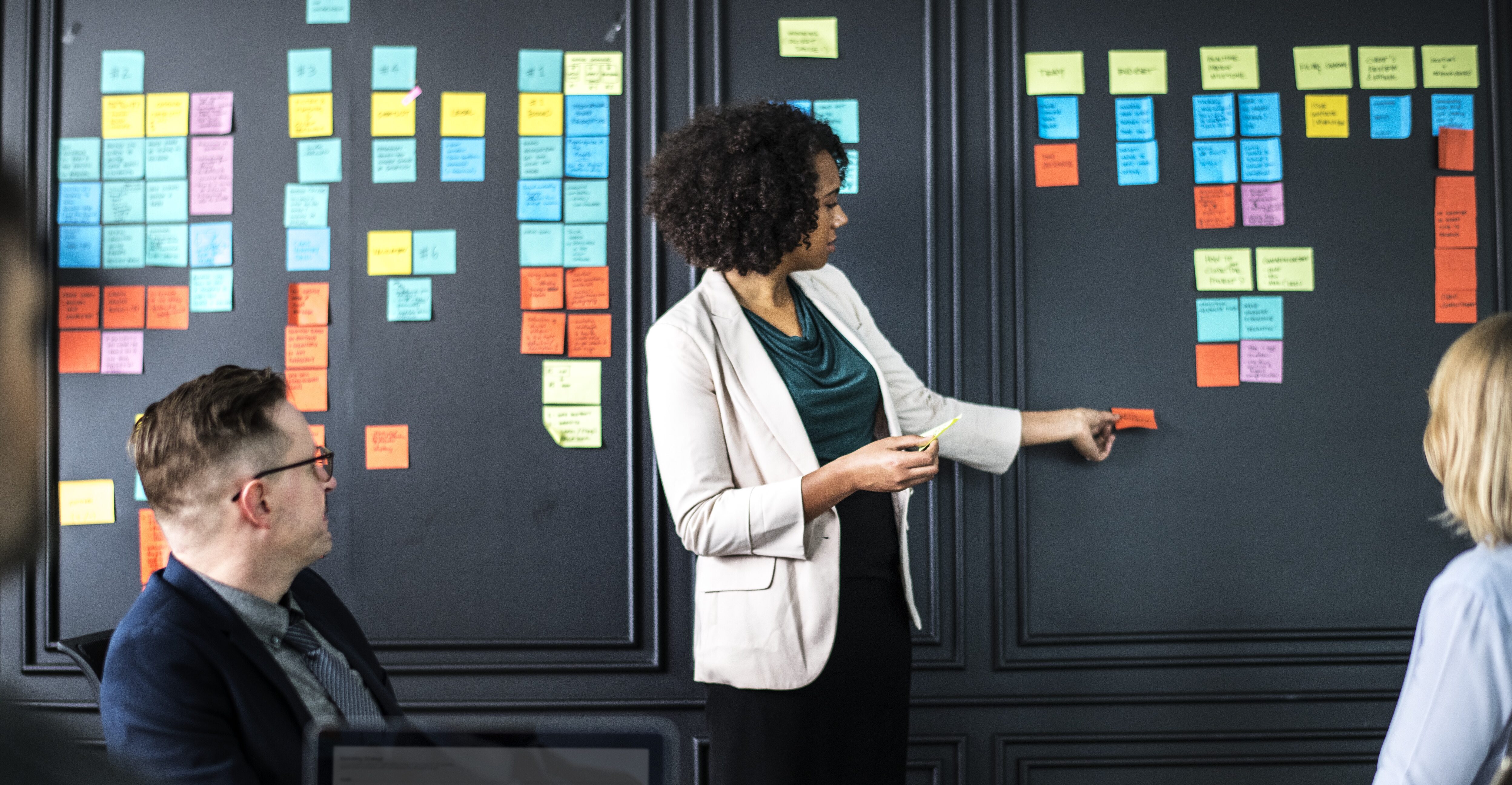
Discover transformative insights and strategies to streamline operations, enhance efficiency, and drive success.