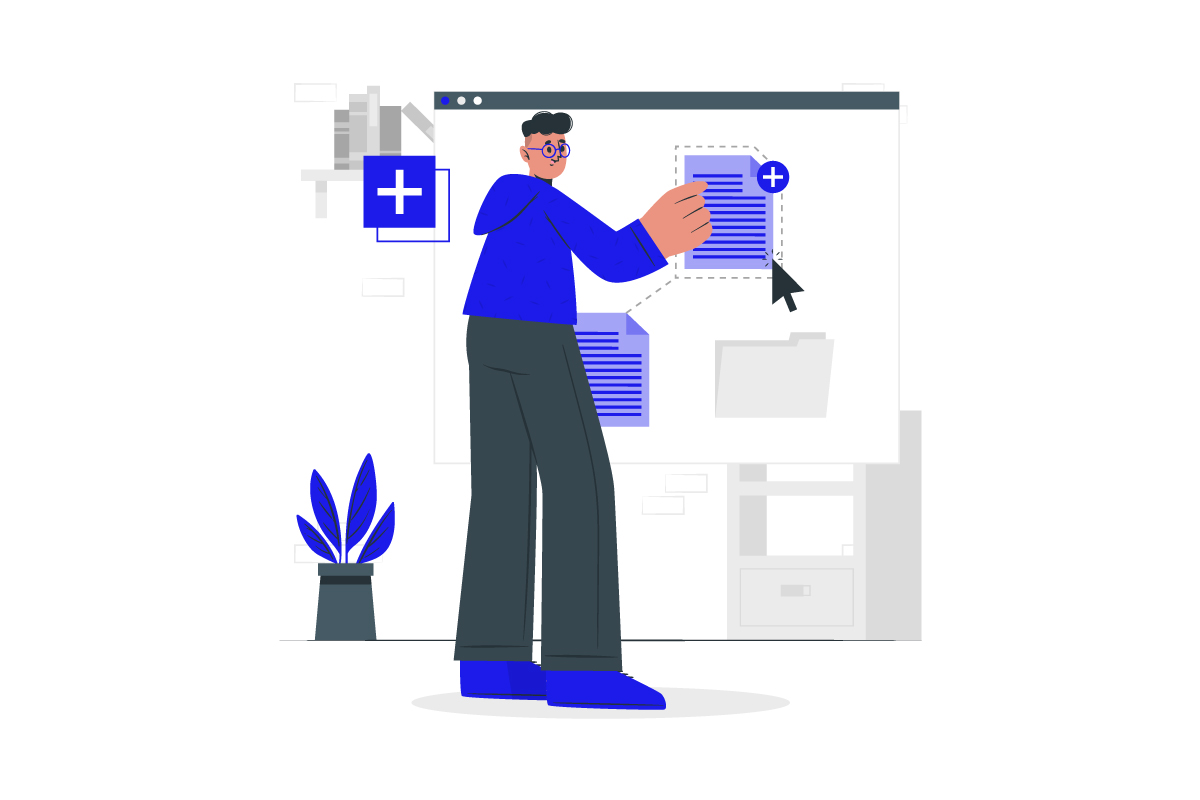
By Luciano Lera Bossi, Alejandro Nava and Parsa Morsal
One of the starting points of digital transformation, especially for financial institutions, is intelligent document processing or IDP. We previously explored classification as the first step in IDP. In this article, we will explore the next crucial step, extraction.
What is document extraction?
Document data extraction is a process of extracting data from structured or unstructured documents and converting them into usable data. It is also called intelligent data capture. With the rapid progress in document imaging technology such as the incorporation of natural language processing (NLP) and optical character recognition (OCR) as well as advanced analytics, we can now enable IT systems to understand the data that was thus far only on paper.
Powered by machine learning models and NLP, IDP systems can now bring the benefits of AI to document processing. The intelligence and detail offered by IDP systems today can be used for many functions including compliance and fraud. The level of granularity, accuracy, and speed offered by IDP systems today, can hugely impact the scale of digital transformation for your organization.
Document processing and the banking industry
The financial industry is no stranger to the benefits of IDP. In a recent study of 200 banks in the US, it was found that 66% of the respondents eliminated the need for manual processes for a typically labor-intensive industry thanks to IDP; and 87% cited accuracy and extraction as the key reasons for incorporating IDP into their systems. Given the emphasis on accuracy and extraction, it is important to understand how intelligent document processing coupled with OCR and NLP can give desired results.
OCR vs IDP
OCR as a document processing technology has been around the longest. OCR is used to extract handwritten or typed text in documents which can then be converted into data. While OCR has been synonymous with data extraction for many years, it is not without its challenges. Without intelligent processing of the data in understanding what the data is for, OCR may give inaccurate results. There can be errors in detecting a text block in an image (error in word detection), there may be errors in interpreting words correctly if there are differences in text alignment or spacing (error in word segmentation) or there may be errors in identifying a character bound in a character image (error in character recognition).
However, when combined with intelligent processes such as NLP and machine learning analytics, time-intensive processing tasks can be sped up with minimal errors. The biggest differentiator between OCR and IDP is that IDP can also handle documents that may be structured, semi-structured, or unstructured.
Structured Documents
Structured documents generally focus on collecting information in a precise format, guiding the person who is filling them with precise areas where each piece of data needs to be entered. These come in a fixed form and are generally called forms. Examples of structured documents include tax forms and credit reports.
Semi-structured Documents
Semi-structured documents are documents that do not follow a strict format the way structured forms do and are not bound to specified data fields. These don’t have a fixed form but follow a common enough format. They may contain paragraphs as well. Example of semi-structured documents could be employment contracts or gift letters.
Unstructured Documents
Unstructured documents are documents in which the information isn’t organized according to a clear, structured model. These files are all easily comprehensible by human beings, yet much more difficult for a robot. Examples of unstructured documents include mortgage commitment statements and municipal tax forms.
Textual and Visual data extraction in IDP
The two main aspects that efficient IDP solutions tackle are textual data extraction and visual data extraction. In textual data extraction, the entity extraction technique is applied to recognize text in a document. This is a machine learning approach where the software is exposed to thousands of documents and the machine “learns” to identify information and segregate it based on certain semantic parameters. Entity extraction can involve a variety of tools and techniques including neural networks to visual layout understanding. By using entity extraction methods, you can avoid going down the template route and thereby use the software on various kinds of documents.
In visual data extraction, IDP solutions can be designed to understand elements such as signatures, tables, checkboxes, logos, etc. Visual data extraction is more complex than textual data extraction as it involves detecting, analyzing, and extracting information from the regions with visual elements accurately while also denoising content that is not relevant. Using machine learning, advanced visual extraction models can also understand the structural relationship of the visual data and its relevance.
Choosing the right IDP solution that can handle both text and visual elements accurately across varying document types will ensure that there is no need for your back office to comb through documents once again.
Explore Kapti, our intelligent document processing software to find out how the power of machine learning and automated document workflows can transform your organization’s document processing experience.