Align, Assemble, Assure: A Framework for AI Adoption
Align, Assemble
Assure
A framework for Adopting and Scaling AI in the Enterprise
Executive Summary
In the rapidly evolving landscape of artificial intelligence (AI), enterprises face significant challenges in differentiating their AI capabilities to achieve strategic objectives. This article provides a comprehensive approach to organizing internal structures across business, technology, and governance/risk/compliance domains to build a robust and differentiated AI capability.
The first part of the article emphasizes the importance of Stakeholder Alignment. To ensure success, AI initiatives must align closely with the organization’s strategic objectives and core values. This alignment ensures that AI projects not only drive innovation but also resonate with the organizational ethos and mission. By integrating AI principles and strategies, enterprises can foster a culture that supports and accelerates AI adoption.
The second section delves into the Operating Model, which is crucial for driving AI innovation and efficiency. This involves defining the ideal team structures and identifying the essential capabilities required across people, processes, and technology. A robust operating model includes a well-defined AI platform that supports strategic objectives and maximizes returns. It also emphasizes the importance of ideation, experimentation, and the productionalization of AI projects to ensure they deliver tangible business value. Effective resource allocation and management are key to maximizing the returns on AI investments.
The third critical aspect covered is Governance, Risk, and Compliance. As AI technologies advance, so do the associated risks and regulatory requirements. It is imperative for enterprises to identify and manage potential risks linked to AI projects proactively. Establishing comprehensive compliance mechanisms ensures that AI applications adhere to all regulatory and ethical standards, thereby safeguarding the organization against legal and ethical pitfalls.
To address these multifaceted challenges, this article introduces the Align | Assemble | Assure (AAA) Framework for AI adoption within enterprises.
- Align: This phase focuses on ensuring that AI initiatives are in sync with the strategic objectives and core values of the enterprise. By developing a clear AI strategy and set of principles, organizations can provide a coherent direction for AI adoption that supports broader business goals.
- Assemble: This phase is about building the necessary capabilities across the organization. It involves organizing teams effectively and ensuring the right mix of talent, processes, and technology. By developing an AI use case portfolio, roadmap, business case, and budget, enterprises can prioritize AI investments and drive projects that offer the highest return on investment (ROI).
- Assure: The final phase focuses on risk management and compliance. This involves assessing AI risks comprehensively and ensuring compliance with internal policies and external regulations. Implementing an AI risk management framework and compliance measures ensures that AI initiatives are secure, ethical, and compliant with all necessary standards.
In the next section, we will explore in depth how the Blanc Labs AAA Framework for AI Adoption can be implemented using actionable and measurable steps.
Align
First, ensure that all AI initiatives are aligned with the company’s strategic objectives and core business values. This involves understanding the business landscape and determining how AI can solve existing problems or create new opportunities. By focusing on alignment, the enterprise ensures that every AI project drives meaningful impact and contributes positively to the overarching goals of the organization.
Here’s how you can effectively execute this alignment:
Identify Strategic Objectives
Business Goals: Begin by clearly understanding the core goals of the organization. What are the key performance indicators (KPIs) or business outcomes that matter most? How can AI contribute to these areas? Thinking through this at the beginning of the process will help you make the right choices pertaining to resources, time and effort, resulting in the long-term success of your AI strategy.
Value Alignment: Ensure that the planned AI initiatives resonate with the company’s values and culture. People are a consequential pillar in AI adoption. Aligning your goals and vision will establish a clarity of purpose, which will in turn drive employee motivation and participation.
Stakeholder Engagement
Collaboration: Engage with stakeholders across various departments to gather insights and identify needs that AI can address. This includes executives, operational staff, and IT teams. AI can improve processes across the mortgage value chain, starting with lead generation and pre-approval at the loan origination stage, going all the way up to default management. Examples of how AI can add value include automating routine tasks to allow employees to concentrate on strategic activities, helping underwriters make complex decisions faster by analyzing research and data; using data to tailor customer experiences, which can boost sales, customer retention, and engagement; and developing comprehensive AI-driven services like chatbots or specialized products for small businesses, which can increase both new and existing revenue streams.
Feedback Loops: Establish continuous communication channels to keep all stakeholders informed and involved in the AI integration process. This helps in adjusting strategies as needed based on real-world feedback and evolving business needs.
Market and Competitive Analysis
Benchmarking: Analyze competitors and industry standards to understand where AI can provide a competitive edge or is necessary to meet industry benchmarks.
Innovative Opportunities: Identify gaps in the current market that AI could fill, potentially opening new business avenues or improving competitive positioning.
Risk Assessment and Mitigation
Identifying Risks: Part of alignment involves understanding the potential risks associated with AI deployments, such as data privacy issues, biases in AI models, or unintended operational impacts.
Mitigation Strategies: Develop strategies to mitigate these risks upfront, ensuring that the AI initiatives proceed smoothly and with minimal disruption.
Scalability and Sustainability
Future-proofing: Consider how the AI initiatives align with long-term business strategies and technological advancements. Ensure that the solutions are scalable and adaptable to future business changes and technological evolution.
Regulatory Compliance
Legal and Ethical Considerations: Ensure that all AI deployments follow relevant laws and ethical guidelines, which is particularly important in industries like healthcare, finance, and public services.
Assemble
Next, assemble a dedicated cross-functional AI Center of Excellence (CoE). This centralized team should consist of experts in AI, data science, ethics, compliance, and business operations. The CoE acts as the hub for AI expertise and collaboration within the company, enabling the standardization of tools, techniques, and methodologies. It also facilitates the pooling of resources and knowledge, ensuring that AI projects across the organization benefit from a consistent approach and high levels of technical and ethical oversight.
To delve deeper into the “Assemble” part of the “Align, Assemble, Assure” framework using the people, process, technology, and organization structure components, we need to consider how each of these elements supports the creation of a robust AI capability within an enterprise.
People
Assembling the right talent is critical. This includes hiring and nurturing:
- AI Specialists: Data scientists, machine learning engineers, and AI researchers who can develop and optimize AI models.
- Technology Experts: Cloud architects, cyber security engineers, solution architects, software developers and testers who can build and support AI applications
- Business Domain Experts: Project managers and business analysts who understand the specific challenges and opportunities within the industry and can ensure that AI solutions are relevant and impactful.
- Support Roles: Risk, legal and compliance officers to oversee AI projects and ensure they align with business and regulatory requirements.
Process
Establishing clear processes ensures AI projects are executed efficiently and effectively:
Development Lifecycle:
Define a standard AI project lifecycle, from ideation and data collection to model training and deployment. Here is a detailed breakdown of the various stages of the project lifecycle, including challenges, solutions and how to measure impact:
Evaluation and Planning: Define critical performance indicators to measure success. Detail both functional and non-functional requirements, outline the necessary output formats, develop a comprehensive monitoring strategy, and define AI literacy requirements for the system. Additionally, determine the necessary data inputs and set clear criteria for explanations. Select the appropriate Generative AI technology, determine how it will be customized, and outline a high-level support and accountability framework. Evaluate and address the risks associated with these activities proportionally, documenting significant effects and strategies for risk mitigation.
Pro Tip: When setting up your evaluation framework, maintain a balance between technical precision and flexibility. This allows your team to adapt quickly to new insights or changes in technology without compromising the system’s integrity or performance. Regularly revisit and refine your performance metrics and requirements to ensure they remain aligned with your strategic goals and the evolving landscape of AI technology.
Data Set Up: Access, clean, and transform data to ensure it is high-quality, well-understood, and relevant for the specific use case. Address data privacy, security, legal, and ethical concerns by putting in place robust safeguards and compliance measures for both the input and output of data, making sure that data owners have approved its use. Set up strict guardrails for the input of information to third-party generative tools and the output from the solution. This includes establishing processes for refining or filtering the output before it reaches the end user, and setting clear restrictions on how the output can be utilized.
Pro Tip: Emphasize the importance of automation in cleansing and transforming your data. This not only saves time but also reduces human error, ensuring consistent data quality. Additionally, continually update and refine your data governance policies and generative AI guardrails to keep pace with technological advancements and evolving regulatory landscapes. This proactive approach will help maintain the integrity and security of your data, enhancing overall trust in your AI solutions.
Development: Start by selecting the optimal tool that matches your requirements in terms of size, language capabilities, and pre-trained features, along with the most appropriate integration method, whether it’s an API or an on-premises solution. Customize your tool using various advanced techniques such as fine-tuning, which involves teaching the tool to perform new or improved tasks like refining output formats, and Retrieval Augmented Generation (RAG), which enhances prompt responses and outputs by incorporating external knowledge sources. Develop and refine a systematic approach to prompt engineering to create, manage, and continuously improve prompts. Focus on refining the solution to boost performance, accuracy, and efficiency through methods like adjusting parameters, tuning hyperparameters, improving data quality, conducting feature engineering, and making architectural adjustments.
Pro Tip: Prioritize the scalability and adaptability of your tools and methods. As you refine and expand your AI applications, ensure that the tools you select can evolve with your needs and can integrate new features or data sources seamlessly. Regularly revisit your prompt engineering and customization strategies to keep them aligned with the latest advancements in AI technology, thus maintaining your competitive edge and maximizing the effectiveness of your solutions.
Implementation and Management: Integrate the AI solution into the operational applications, ensuring seamless transition into production environments. Assign clear ownership to oversee the solution’s lifecycle. Develop a support structure that includes performance-based Service Level Agreements (SLAs) and operational guidelines aimed at fulfilling the established non-functional requirements, and introduce practices for consistent data management. Enhance organizational understanding and capability through targeted AI training programs. Ensure diligent registration of any new use of AI. Complete all the necessary documentation to provide clear explanations and transparency regarding the AI solution’s functionalities and decisions.
Pro Tip: Establish a feedback loop between the operational performance and the development teams. This ensures that any insights gained from real-world application can be swiftly acted upon to refine the solution. Regularly updating your integration practices and operational protocols in response to these insights will help you maintain high standards of performance and reliability, ensuring that your AI system remains robust and effective in ever-changing environments.
Monitoring and Maintenance: Implement continuous monitoring of the deployed AI solution to assess its performance, accuracy, overall impact (including any unintended biases), usage, costs, and data management practices. Promptly investigate and resolve any issues or anomalies that arise during operation. Utilize the insights gathered from the monitoring process to regularly update, maintain, and enhance the solution. Maintain vigilant oversight of any updates made to the AI system. Ensure all new applications of the AI are properly registered. Occasionally, updates pushed by the supplier might require a comprehensive review due to potential significant changes. Provide ongoing reporting to highlight the benefits and value added by the AI solution.
Pro Tip: Consider implementing automated tools that can alert you to anomalies in real-time. Regularly scheduled reviews of the system’s outputs and operations can help preempt problems before they escalate, ensuring that the AI continues to operate efficiently and effectively. Moreover, documenting every adjustment and update not only aids in compliance and governance but also provides valuable historical data that can inform future enhancements and deployments.
The Role of AI Quality Assurance and Testing
AI development demands rigorous and continuous testing. The role of Quality Assurance (QA) and Testing is to assess the relevance and effectiveness of the training data, ensuring it performs as intended. This process starts with basic validation techniques, where QA engineers select portions of the training data for the validation phase. They test this data in specific scenarios to evaluate not only the algorithm’s performance on familiar data but also its ability to generalize to new, unseen data. Evaluation metrics such as accuracy, precision, recall, and the F1 score are defined based on the specific use case, recognizing that not all metrics are appropriate for every scenario.
If significant errors are detected during validation, the AI must undergo modifications, similar to traditional software development cycles. After adjustments, the AI is retested by the QA team until it meets the expected standards. However, unlike other software, AI testing by the QA team does not conclude after one cycle. QA engineers must repeatedly test the AI with various datasets for an indefinite period, depending on the desired thoroughness or available resources, all before the AI model goes into production.
During this repetitive testing phase, also known as the “training phase,” developers should test the algorithm on various fronts. Notably, QA teams will need to focus not on the code or algorithm itself but on whether the AI fulfills its intended function. QA engineers for AI testing will primarily work with hyperparameter configuration and training data, using cross-validation to ensure correct settings.
The final focus is on the training data itself, evaluating its quality, completeness, potential biases, or blindspots that might affect real-world performance. To effectively address these issues, QA teams need access to representative real-world data samples and a deep understanding of AI bias and ethics. This comprehensive approach will help them pose critical questions about the AI’s design and its ability to realistically model the scenarios it aims to predict, ensuring robustness and reliability across various applications.
Technology
To deliver comprehensive and effective AI solutions, enterprises need to establish robust technology and tooling capabilities within their AI platforms. These capabilities span several domains, each critical for developing, deploying, and managing Gen AI, Cognitive AI and machine learning (ML) models.
Gen AI Development is foundational for creating full-featured generative AI applications. Enterprises must have the capability to customize and deploy models to meet specific business needs. This includes fine-tuning model inputs and engineering prompts to enhance performance and relevance. Additionally, managing the lifecycle of models during development is crucial, ensuring that models are continuously improved and updated. Orchestrating various AI models allows enterprises to integrate multiple AI systems seamlessly, optimizing the overall functionality and efficiency of the AI platform.
Gen AI and ML Operations focus on the day-to-day management and fine-tuning of AI models once they are in production. This involves managing the lifecycle of models and their versions to maintain consistency and reliability. Effective access management to models ensures that only authorized personnel can modify or utilize these models, enhancing security. Fine-tuning ML models and their training is essential to adapt to new data and evolving business requirements. Furthermore, managing data sources efficiently ensures that the models are fed with accurate and relevant data, which is vital for generating reliable outputs.
Gen AI and ML Governance is essential for maintaining the integrity and compliance of AI systems. Enterprises need to monitor models continuously to ensure they produce accurate and appropriate responses. Safeguarding models from inappropriate or malicious inputs is critical to prevent misuse and potential harm. Compliance with legal and regulatory frameworks is another significant aspect, ensuring that AI operations adhere to the necessary standards and regulations. Managing the data leveraged by models helps maintain data privacy and security, which is paramount in today’s regulatory environment.
Cognitive AI Capabilities are designed to mimic human cognitive functions, providing advanced interactions through APIs and SDKs. These capabilities include understanding and translating languages, recognizing images and sounds, and extracting and summarizing data. By integrating these cognitive functions, enterprises can enhance their AI applications to provide more natural and intuitive interactions, thereby improving user experience and engagement.
Establishing these comprehensive technology and tooling capabilities within an enterprise AI platform is crucial for developing robust, secure, and compliant AI solutions. By focusing on these areas, enterprises can harness the full potential of AI, driving innovation and achieving strategic business objectives.
Organization Structure
Effective organization structure facilitates AI adoption and integration:
- Centralized AI Unit: An AI Center or hub, possibly under a Chief AI Officer, to centralize expertise and provide leadership.
- Cross-functional Teams: Integration of AI teams with other business units to promote collaboration and ensure AI solutions meet business needs.
- Change Management: Structures to support change management processes, helping the workforce adapt to new technologies and methods introduced by AI.
Assure
Finally, implement robust governance to assure the safety, compliance, and ethical integrity of AI deployments. This includes setting up frameworks for ongoing monitoring and evaluation of AI systems, ensuring they adhere to regulatory requirements and ethical standards. The governance process should also involve stakeholder engagement to maintain transparency and address any concerns related to AI projects.
Responsible AI
Responsible use of AI will require a commitment to ethical guidelines that prioritize:
Transparency & Explainability: Create AI systems that are both transparent and clear in their functioning. As an organization, you should be able to pinpoint and clarify AI-driven decisions and outcomes. This will be especially important in the context of customers and stakeholders.
Compliance: Designate responsible individuals for AI systems and ensure that the systems comply with regulatory standards. Conduct frequent audits to ensure the accuracy of your AI solutions, handle unforeseen outcomes, and check that the solutions fulfill legal and regulatory requirements.
Equity and Diversity: Design, develop and deploy AI in an ethical, fair and inclusive manner. Proactively strive to identify and address biases that can emerge from training data or decision-making algorithms.
Data Privacy: It’s imperative to safeguard personal data and prioritize individuals’ well-being, preventing any potential harm. Commit to respecting privacy rights, secure personal data, and mitigate potential risks and negative impacts on customers by obtaining consent and implementing responsible data practices.
Security: Create AI solutions that can withstand attacks, malfunctions and manipulation. Implement safeguards to secure AI data, services, networks and infrastructure to prevent unauthorized access, breaches or data manipulation.
Examples of Prohibited Use Cases:
Using a risk assessment framework guided by the ethical AI principles mentioned above, here is an example of use cases that will not pass:
- Assessing, categorizing, or evaluating individuals based on their biometric information, personal attributes, or social behavior.
- Using subliminal, manipulative, or misleading techniques to sway an individual’s behavior.
- Taking advantage of people’s vulnerabilities (e.g., their age, disability, or social/economic status).
- Emotion recognition, as defined by your legal department.
- Collecting biometric data indiscriminately.
- Generating false content that seems to represent a particular person.
See Also: Artificial Intelligence and Data Act Canada
The proposed Artificial Intelligence and Data Act (AIDA) aims to establish standards for the responsible design, development, and deployment of AI systems, ensuring they are safe and non-discriminatory. This legislation will require businesses to identify and mitigate the risks of their AI systems, and to provide transparent information to users.
Under AIDA, the level of safety obligations for AI systems will depend on the associated risks, and businesses will need to adhere to new regulations across the design, development, and deployment stages. The government is working to create regulations that align with existing standards, aiming to facilitate compliance for businesses. A new AI and Data Commissioner will monitor compliance to ensure AI systems are fair and non-discriminatory. By introducing this law, Canada is among the first countries to propose AI regulation, aiming to balance innovation with safety, and ensuring international competitiveness while considering the needs of all stakeholders.
For more information, view the full act.
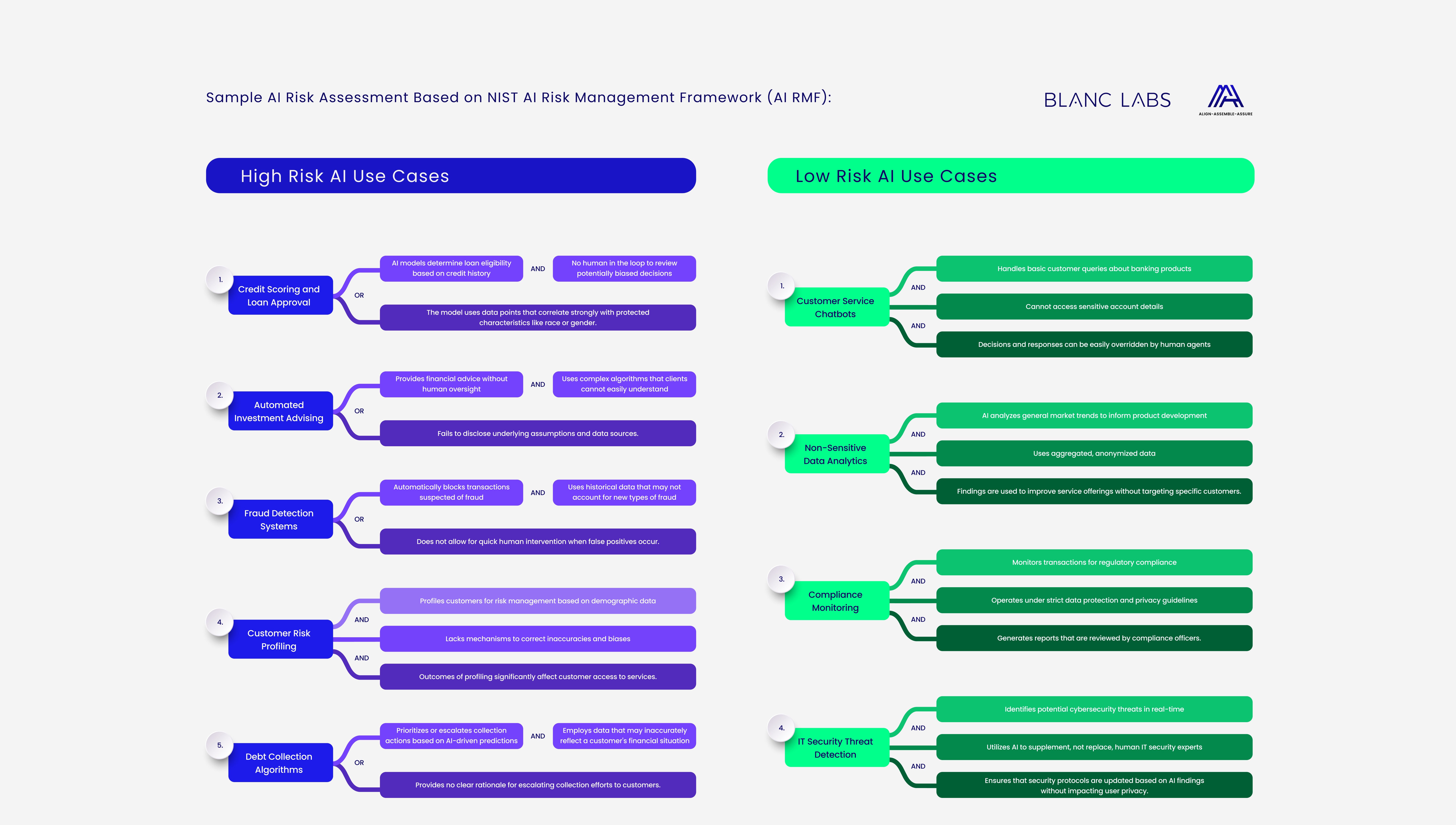
The diagram above shows how you can create your own AI risk assessment framework. Risk can be measured along four factors: personal information, decision-making, bias and external access.
In Conclusion
With the principle of “Align, Assemble, Assure,” the enterprise can methodically approach AI integration, ensuring that the efforts are strategically sound, well-supported by a specialized team, and maintained under stringent ethical and regulatory standards. This principle not only fosters innovation and efficiency but also builds trust and reliability in AI applications across the business.
Take the first step towards transforming your business with AI
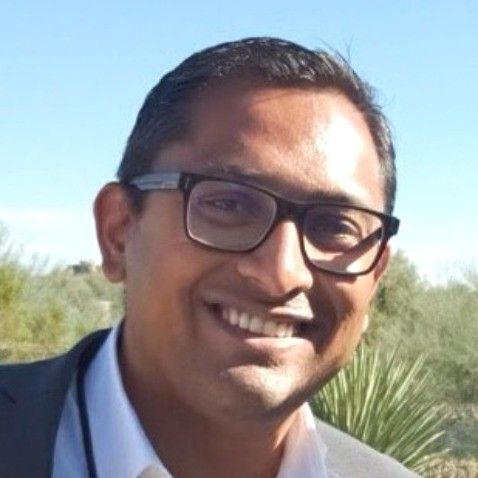
Prithvi Srinivasan, the Managing Director for Advisory Services, brings extensive expertise in technology strategy and digital transformation to drive mission-critical programs and advance financial institutions with AI and automation. His ability to transform complex strategies into successful transformations underscores his commitment to innovation and client value.